Bayesian linear models
In this section, we are going to extend the standard linear regression model using the Bayesian paradigm. One of the goals is to put prior knowledge on the parameters of the models to help to solve the over-fitting problem.
Over-fitting a model
One immense benefit of going Bayesian when doing a linear model is to have better control of the parameters. Let's do an initial experiment to see what happens when the parameters are completely out of control.
We are going to generate a simple model in R and look at the parameters when they are fitted with the standard approach for linear models.
Let's first generate some data points at random to obtain 10 variables and plot them:
N <- 30 x <- runif(N, -2, 2) X <- cbind(rep(1, N), x, x^2, x^3, x^4, x^5, x^6, x^7, x^8) matplot(X, t='l')
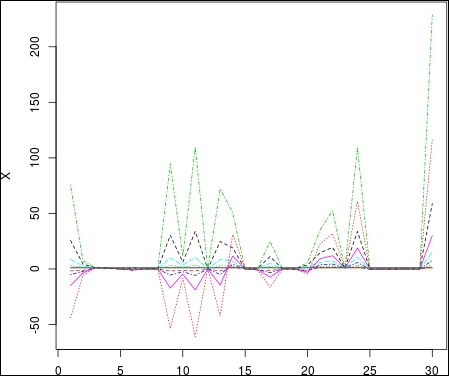
Next we generate the dependent variable following the model:
y = Xβ + ϵ
Here, ϵ is a Gaussian noise of variance σ2. We use the following code in R and plot the variable y. As we use randomly...