Indexing multiple documents using Bulk API
In this recipe, we will explore how to use the Elasticsearch client to ingest an entire movie dataset using the bulk API. We will also integrate various concepts we have covered in previous recipes, specifically related to mappings, to ensure that the correct mappings are applied to our index.
Getting ready
For this recipe, we will work with the sample Wikipedia Movie Plots dataset introduced at the beginning of the chapter. The file is accessible in the GitHub repository via this URL: https://github.com/PacktPublishing/Elastic-Stack-8.x-Cookbook/blob/main/Chapter2/dataset/wiki_movie_plots_deduped.csv.
Make sure that you have completed the previous recipes:
- Using an analyzer
- Creating index template
How to do it…
Head to the GitHub repository to download the Python script at https://github.com/PacktPublishing/Elastic-Stack-8.x-Cookbook/blob/main/Chapter2/python-client-sample/sampledata_bulk.py and then follow these steps:
- Update the
.env
file with theMOVIE_DATASET
variable, which specifies the path to the downloaded movie dataset CSV file:MOVIE_DATASET=<the-path-of-the-csv-file>
- Once the
.env
file is correctly configured, run thesampledata_bulk.py
Python script. During execution, you should see output similar to the following (note that, for readability, the output image has been truncated):
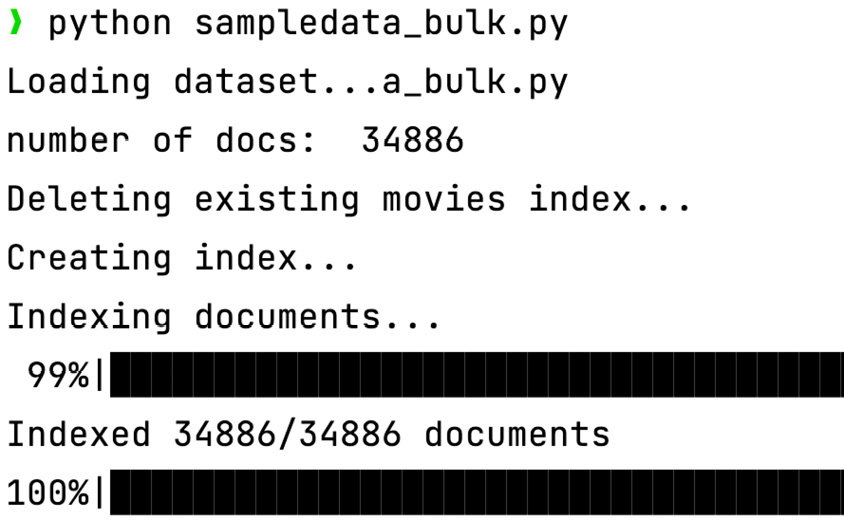
Figure 2.19 – The output of the sampledata_bulk.py script
- To verify that the new
movies
index has the appropriate mappings, head to Kibana | Dev Tools and execute the following command:GET /movies/_mapping
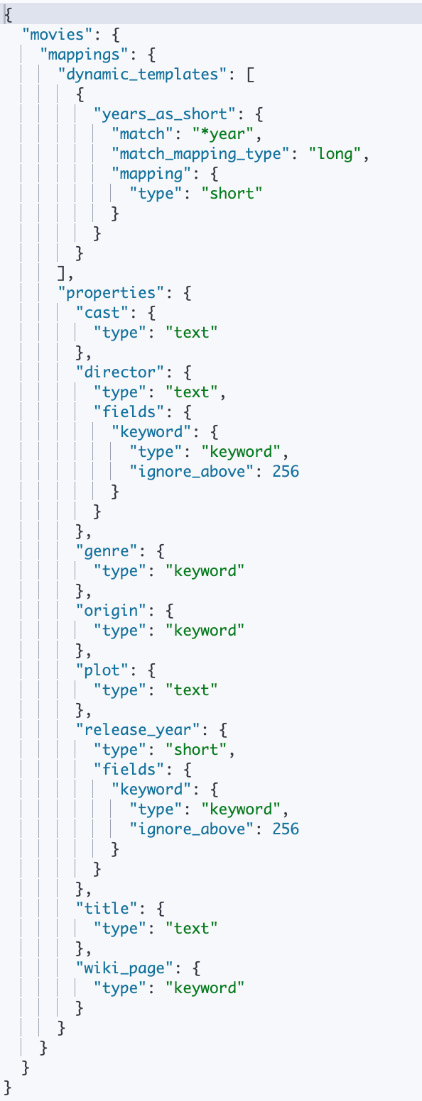
Figure 2.20 – The movies index with a new mapping
- As illustrated in Figure 2.20, dynamic mapping on the
release_year
field was applied to the newly createdmovies
index, despite a mapping being explicitly specified in the script. This occurred because an index template was defined in the Using dynamic templates in document mapping recipe, with the index pattern set tomovies*
. As a result, any index that matches this pattern will automatically inherit the settings from the template, including its dynamic mapping configuration. - Next, to verify that the entire dataset has been indexed, execute the following command:
GET /movies/_count
The command should produce the output illustrated in Figure 2.21. According to this output, your
movies
index should contain 34,886 documents:
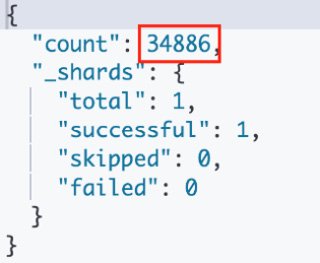
Figure 2.21 – A count of the documents in bulk-indexed movies
We have just set up an index with the right explicit mapping and loaded an entire dataset by using the Elasticsearch Python client.
How it works...
The script we’ve provided contains several sections. First, we delete any existing movies
indexes to make sure we start from a clean slate. This is the reason you did not see the award_year
and review_year
fields in the new mapping shown in Figure 2.20. We then use the create_index
method to create the movies
index and specify the settings and the mappings we wish to apply to the documents that will be stored in this index.
Then, there is the generate_actions
function that yields a document for each row in our CSV dataset. This function is then used by the streaming_bulk
helper method.
The streaming_bulk
helper function in the Elasticsearch Python client is used to perform bulk indexing of documents in Elasticsearch. It is like the bulk
helper function, but it is designed to handle large datasets.
The streaming_bulk
function accepts an iterable of documents and sends them to Elasticsearch in small batches. This strategy allows you to efficiently process substantial datasets without exhausting system memory.
There’s more…
The Elasticsearch Python Client provides several helper functions for the bulk API, which can be challenging to use directly because of its specific formatting requirements and other considerations. These helpers accept an instance of the es
class and an iterable
action, which can be any iterable or generator.
The most common format for the iterable
action is the same as that returned by the search()
method. The bulk()
API accepts the index
, create
, delete
, and update
actions. The _op_type
field is used to specify an action, with _op_type
defaulting to index
. There are several bulk helpers available, including bulk()
, parallel_bulk()
, streaming_bulk()
, and bulk_index()
. The following table outlines these helpers and their preferred use cases:
Bulk helper functions |
Use cases |
|
This helper is used to perform bulk operations on a single thread. It is ideal for small- to medium-sized datasets and is the simplest of the bulk helpers. |
|
This helper is used to perform bulk operations on multiple threads. It is ideal for large datasets and can significantly improve indexing performance. |
|
This helper is used to perform bulk operations on a large dataset that cannot fit into memory. It is ideal for large datasets and can be used to stream data from a file or other source. |
|
This helper is used to perform bulk indexing operations on a large dataset that cannot fit into memory. It is ideal for large datasets and can be used to stream data from a file or other source. |
Table 2.1 – Bulk helper functions and their associated use cases
See also
If you are interested in more examples of bulk ingest using the Python client, check out the official Python client repository: https://github.com/elastic/elasticsearch-py/tree/main/examples/bulk-ingest.