In this chapter, we will be focusing on the basics of neural networks, including input/output layers, hidden layers, and how the networks learn through forward and backpropagation. We will start with the standard multilayer perceptron networks, talk about their building blocks, and illustrate how they learn step-by-step. We will also introduce a few, popular standard models such as Convolutional Neural Networks (CNN), Restricted Boltzmann Machines (RBM), and recurrent neural network (RNN) as well as its variation Long Short-Term Memory (LSTM). We will outline the key, critical components for the successful application of the models, and explain some important concepts to help you gain a better understanding of why these networks work so well in certain areas. In addition to a theoretical introduction, we will also show example code snippets...




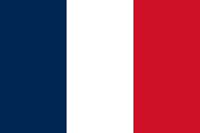


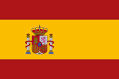



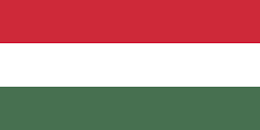

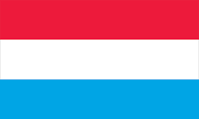





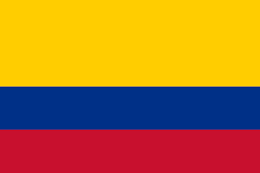
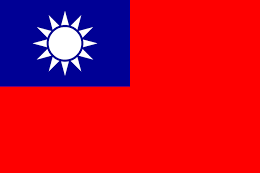
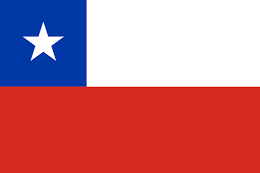



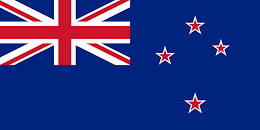
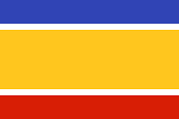
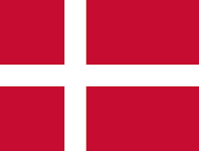
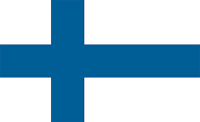







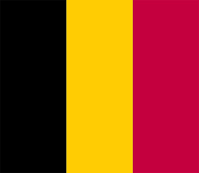

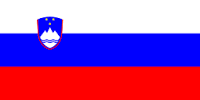





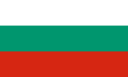


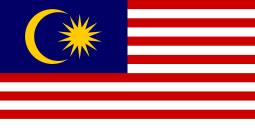



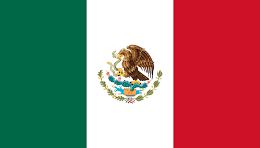
