Many problems we find in science, engineering, and business are of the following form. We have a variable and we want to model/predict a variable
. Importantly, these variables are paired like
. In the most simple scenario, known as simple linear regression, both
and
are uni-dimensional continuous random variables. By continuous, we mean a variable represented using real numbers (or floats, if you wish), and using NumPy, you will represent the variables
or
as one-dimensional arrays. Because this is a very common model, the variables get proper names. We call the
variables the dependent, predicted, or outcome variables, and the
variables the independent, predictor, or input variables. When
is a matrix (we have different variables), we have what is known as multiple linear regression. In this and the following chapter, we will explore these and other...




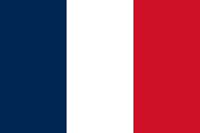


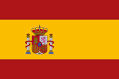



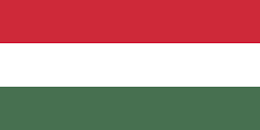

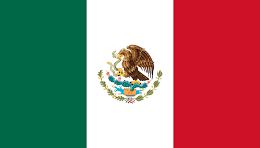


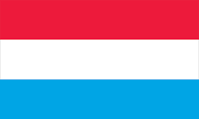



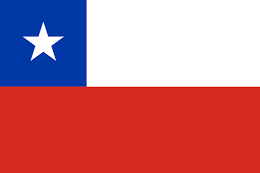


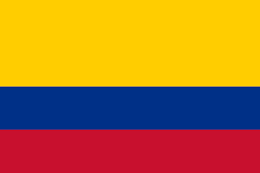
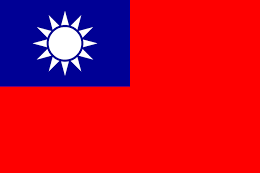


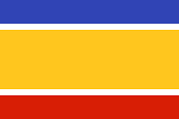
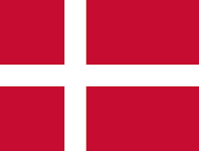
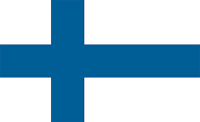



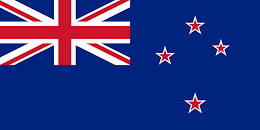





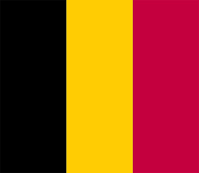

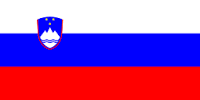




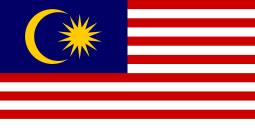


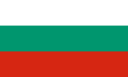


