Machine learning work flow in general involves two set of operations: one involving training the model and the other testing the trained model with newer datasets.
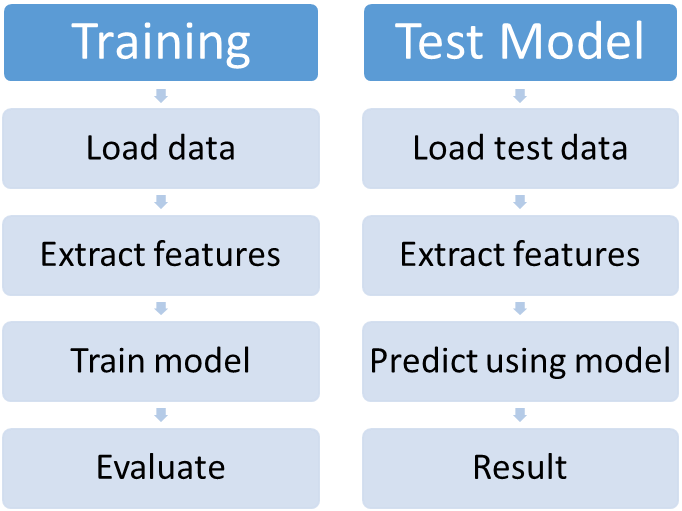
The ML workflow requires iterations over the same set of training data over and over again while only changing the tuning parameters. The objective of such an iterative process is to achieve minima in error while evaluating the values on training data and only then the model is fed with test data to give resultant output. In order to combine all these sequences of data pre-processing, feature extraction, model fitting, and validation stages in a ML workflow, spark introduced Pipelines.