




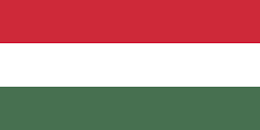

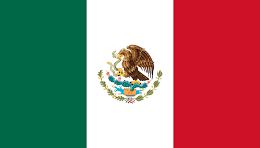


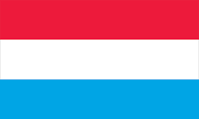



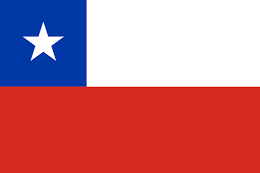



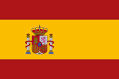


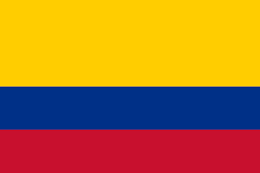
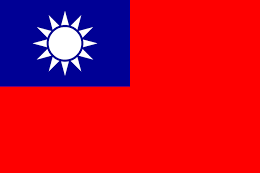


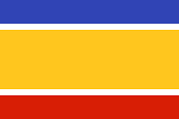
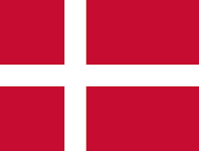
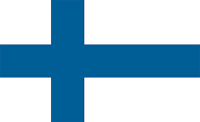



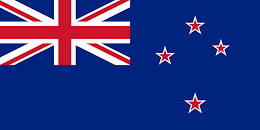


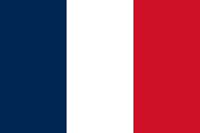



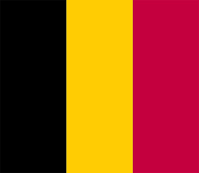

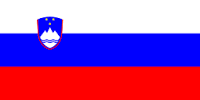




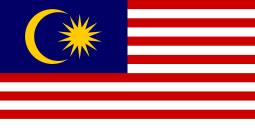


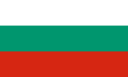




Deep learning and 3D vision research have led to major developments in the field of robotics and computer graphics. However, there is a dearth of systems that allow easy loading of popular 3D datasets and get the 3D data across various representations converted into modern machine learning frameworks. To overcome this barrier, researchers at NVIDIA have developed a 3D deep learning library for PyTorch called ‘Kaolin’. Last week, the researchers published the details of Kaolin in a paper titled “Kaolin: A PyTorch Library for Accelerating 3D Deep Learning Research”.
https://twitter.com/NvidiaAI/status/1194680942536736768
Kaolin provides an efficient implementation of all core modules that are required to build 3D deep learning applications. According to NVIDIA, Kaolin can slash the job of preparing a 3D model for deep learning from 300 lines of code down to just five.
A differentiable renderer is a process that supplies pixels as a function of model parameters to simulate a physical imaging system. It also supplies derivatives of the pixel values with respect to those parameters. With an aim to allow users the easy use of popular differentiable rendering methods, Kaolin provides a flexible and modular differentiable renderer.
It defines an abstract base class called ‘DifferentiableRenderer’ which contains abstract methods for each component in a rendering pipeline. The abstract methods allowed in Kaolin include geometric transformations, lighting, shading, rasterization, and projection. It also supports multiple lighting, shading, projection, and rasterization modes.
One of the important aspects of any computer vision task is visualizing data. Kaolin delivers visualization support for all of computer vision representation types. It is implemented via lightweight visualization libraries such as Trimesh, and pptk for running time visualization.
The researchers say, “While we view Kaolin as a major step in accelerating 3D DL research, the efforts do not stop here. We intend to foster a strong open-source community around Kaolin, and welcome contributions from other 3D deep learning researchers and practitioners.” The researchers are hopeful that the 3D community will try out Kaolin, and contribute to its development.
Many developers have expressed interest in the Kaolin PyTorch Library.
https://twitter.com/RanaHanocka/status/1194763643700858880
https://twitter.com/AndrewMendez19/status/1194719320951197697
Read the research paper for more details about Kaolin’s roadmap. You can also check out NVIDIA’s official announcement.
Facebook releases PyTorch 1.3 with named tensors, PyTorch Mobile, 8-bit model quantization, and more
Transformers 2.0: NLP library with deep interoperability between TensorFlow 2.0 and PyTorch, and 32+ pretrained models in 100+ languages
Introducing ESPRESSO, an open-source, PyTorch based, end-to-end neural automatic speech recognition (ASR) toolkit for distributed training across GPUs
Baidu adds Paddle Lite 2.0, new development kits, EasyDL Pro, and other upgrades to its PaddlePaddle deep learning platform
CNCF announces Helm 3, a Kubernetes package manager and tool to manage charts and libraries