Clustering is intended to group data variables that are found to be interrelated, based on observations of their attributes' values. However, given a scenario with a large number of attributes, the data scientist will find that some of the attributes will usually not be meaningful for a given cluster. In the example, we used earlier in this chapter (dealing with patient cases), we could have found this situation. Recall that we performed a hierarchical cluster analysis on smokers only. Those cases include many attributes, such as, sex, age, weight, height, no_hospital_visits, heartrate, state, relationship, Insurance blood type, blood_pressure, education, date of birth, current_drinker, currently_on_medications, known_allergies, currently_under_doctors_care, ever_operated_on, occupation, heart_attack, rheumatic_fever, heart_murmur, diseases_of_the_arteries...




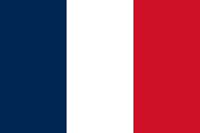


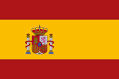




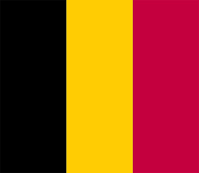
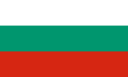
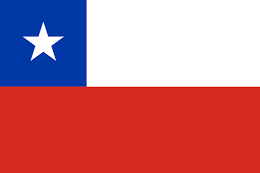
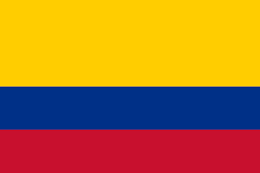
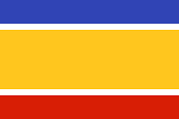

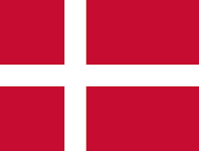



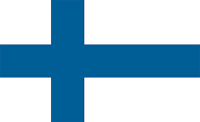

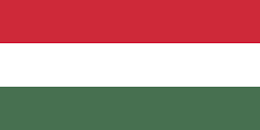






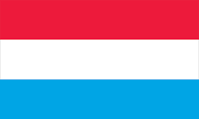
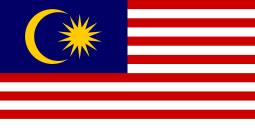

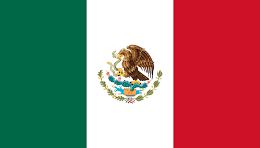

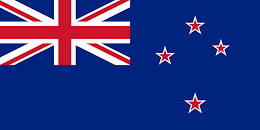







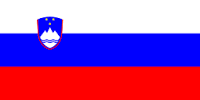




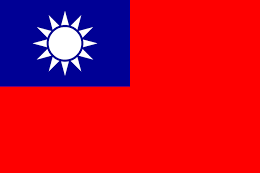


