The Q-learning algorithm, as we saw in Chapter 4, Q-Learning and SARSA Applications, has many qualities that enable its application in many real-world contexts. A key ingredient of this algorithm is that it makes use of the Bellman equation for learning the Q-function. The Bellman equation, as used by the Q-learning algorithm, enables the updating of Q-values from subsequent state-action values. This makes the algorithm able to learn at every step, without waiting until the trajectory is completed. Also, every state or action-state pair has its own values stored in a lookup table that saves and retrieves the corresponding values. Being designed in this way, Q-learning converges to optimal values as long as all the state-action pairs are repeatedly sampled. Furthermore, the method uses two policies: a non-greedy behavior policy to gather experience...




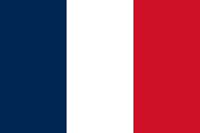


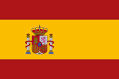




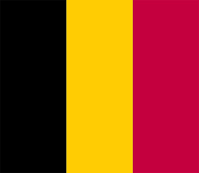
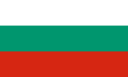
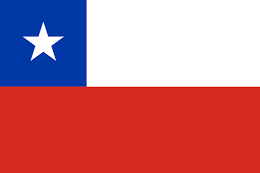
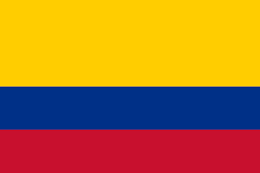
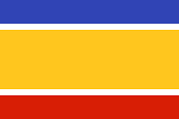

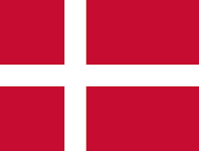



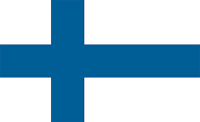

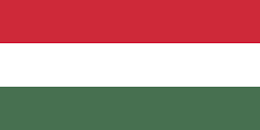






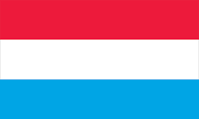
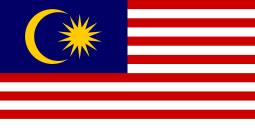

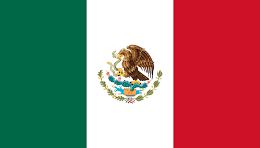

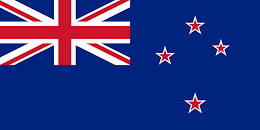







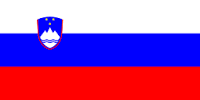




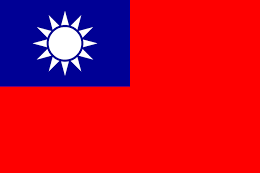


