In the Tuning hyperparameters using grid search and cross-validation recipe in Chapter 8, Identifying Credit Default with Machine Learning, we described how to use grid search and randomized search to find the (possibly) best set of hyperparameters for our model. In this recipe, we introduce another approach to finding the optimal set of hyperparameters, this time based on the Bayesian methodology. The main motivation for the Bayesian approach is that both grid search and randomized search make uninformed choices, either through exhaustive search over all combinations or through a random sample. This way, they spend a lot of time evaluating bad (far from optimal) combinations, thus basically wasting time. That is why the Bayesian approach makes informed choices of the next set of hyperparameters to evaluate, this way reducing the time spent...




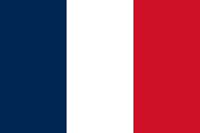


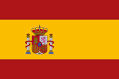



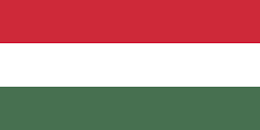

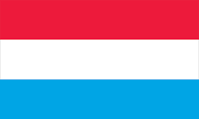





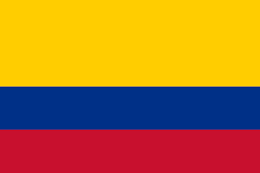
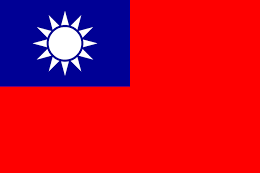
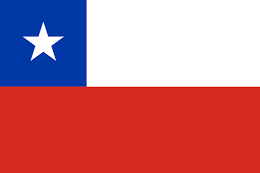



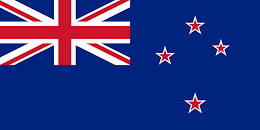
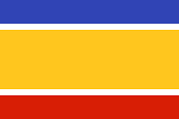
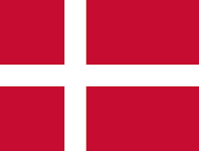
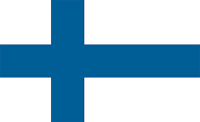







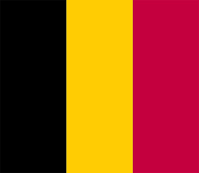

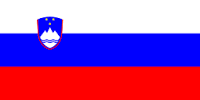





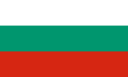


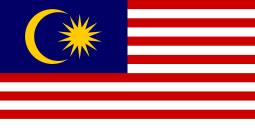



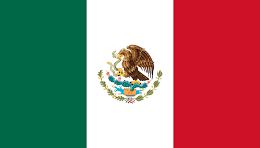
