



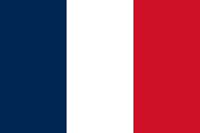


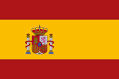



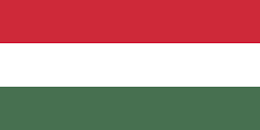

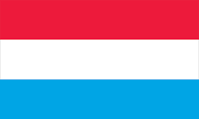





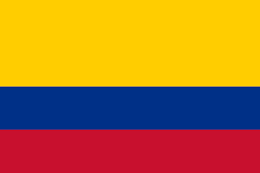
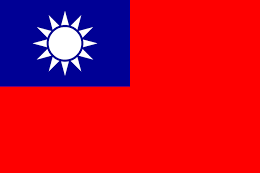
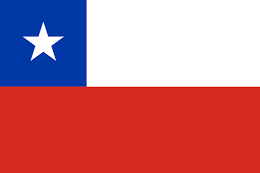



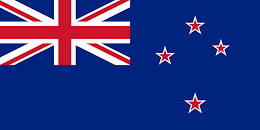
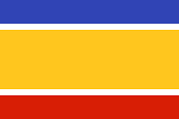
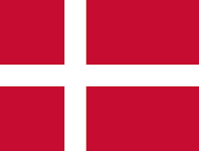
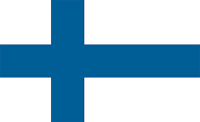







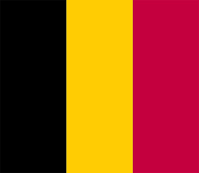

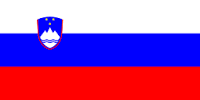





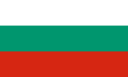


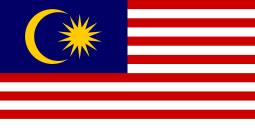



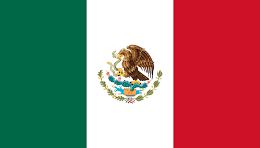

Healthcare systems generate copious amounts of data and use them for disease prediction. However, the necessary software to generate meaningful insights from this unstructured data is often not in place. Hence, drug and disease discovery end up taking time. Machine Learning algorithms can discover signatures of diseases at rapid rates by allowing systems to learn and make predictions based on the previously processed data. They can also be used to determine which chemical compounds could work together to aid drug discovery. Thus the time-consuming process of experimenting and testing millions of compounds is eliminated. With the fast discovery of diseases, the chances of detecting symptoms earlier and the probability of survival increases. It also boosts available treatment options.
IBM has collaborated with Teva Pharmaceutical to discover new treatment options for respiratory and central nervous system diseases using Machine Learning algorithms such as predictive and visual analytics that run on IBM Watson Health Cloud. To gain more insights on how IBM Watson is changing the face of healthcare, check this article.
Precision Medicine revolves around healthcare practices specific to a particular patient. This includes analyzing a person’s genetic information, health history, environmental exposure, and needs and preferences to guide diagnosis for diseases and subsequent treatment. Here, machine learning algorithms are utilized to sift through vast databases of patient data to identify factors such as their genetic history and predisposition to diseases, that could strongly determine treatment success or failure. ML techniques in precision medicine exploit molecular and genomic data to assist doctors in directing therapies to patients and shed light on disease mechanisms and heterogeneity. It also predicts what diseases are likely to occur in the future and suggests methods to avoid them.
Cellworks, a Life Sciences Technology company, brings together a SaaS-based platform for generating precision medicine products. Their platform analyses the genomic profile of the patient and then provides patient-specific reports for improved diagnosis and treatment.
CTI and MRI scans for radiological diagnosis and interpretation are burdensome and laborious (not to mention, time-consuming). They involve segmentation—differentiating between healthy and infectious tissues—which when done manually has a good probability of resulting in errors and misdiagnosis. Machine Learning algorithms can speed up the segmentation process while also increasing accuracy in radiotherapy planning. ML can provide physicians information for better diagnostics which helps in obtaining accurate tumor location. It also predicts radiotherapy response to help create a personalized treatment plan.
Apart from these, ML algorithms find use in medical image analysis as they learn from examples. This involves classification techniques which analyze images and available clinical information to generate the most likely diagnosis. Deep Learning can also be used for detecting lung cancer nodules in early screening CT scans and displaying the results in useful ways for clinical use.
Google’s machine-learning division, DeepMind, is automating radiotherapy treatment for head and neck cancers using scans from almost 700 diagnosed patients. An ML algorithm scans the reports of symptomatic patients against these previous scans to help physicians develop a suitable treatment process. Arterys, a cloud-based platform, automates cardiac analysis using deep learning.
A large number of neurological diseases develop gradually or in stages, so the decay of the brain happens over time. Traditional approaches to neurological care such as peak activation, EEG epileptic spikes, Pronator drift etc., are not accurate enough to diagnose and classify neurological and psychiatric disorders. This is because they are typically used for end results assessment rather than for progressive analysis on how the brain disease develops. Moreover, timely personalized neurological treatments and diagnoses rely highly on the constant availability of an expert. Machine Learning algorithms can advance the science of detection and prediction by learning how the brain progressively develops into these conditions. Deep Learning techniques are applied in the area of neuroimaging to detect abstract and complex patterns from single-subject data to detect and diagnose brain disorders. Machine learning techniques such as SVM, RBFN, and RF are amalgamated with PDT (Pronator drift tests) to detect stroke symptoms based on quantification of proximal arm weakness using inertial sensors and signal processing.
Machine Learning algorithms can also be used for detecting signs of dementia before its onset. The Douglas Mental Health University Institute uses PET scans to train ML algorithms to spot signs of dementia by analyzing it against scans of patients who have mild cognitive impairment. Then they run the scans belonging to symptomatic patients on the trained algorithm to predict the possibility of dementia.
Epidemic predictions traditionally rely on manual accounting. This includes self-reports or aggregation of information from healthcare services such as reports by different health protection agencies like CDC, NHIS, National Immunization Survey etc. However, they are time-consuming and error-prone. Thus predicting and prioritizing the outbreaks becomes challenging. ML algorithms can automatically perform analysis, improve calculations and verify information with minimal human intervention.
Machine learning techniques like support vector machines and artificial neural networks can predict the epidemic potential of a disease and provide alerts for disease outbreak. They do this using data collected from satellites, and from real-time social media updates, historical information on the web, and other sources. They also use geospatial data such as temperature, weather conditions, wind speed, and other data points to predict the magnitude of impact an epidemic can cause in a particular area and to recommend necessary measures for preventing and containing them early on.
AIME, a medical startup, has come up with an algorithm to predict outcome and even the epicenter of epidemics such as dengue fever before their occurrence.
Machine Learning can bring about a change in traditional hospital management systems by envisioning hospitals as a digital patient-centric care center. These include automating routine tasks such as billing, admission and clearance, monitoring patients’ vitals etc. With administrative tasks out of the way, hospital authorities could fully focus on the care and treatment of patients. ML techniques such as computer vision can be used to feed all the vital signs of a patient directly into the EHR from the monitoring devices. Smart tracking devices are also used on patients to provide real-time whereabouts. Predictive analysis techniques provide continuous stream of real-time images and data. This analysis can sense risk and prioritize activities for the benefit of all patients. ML can also automate non-clinical functions, including pharmacy, laundry, and food delivery.
The John Hopkins Hospital has its own command center that uses predictive analytics for efficient operational flow.
The digital health era focuses on health and wellness rather than diseases. The incorporation of machine learning in healthcare provides an improved patient experience, a better public health management, and reduces costs by automating manual labour. The next step in this amalgamation is a successful collaboration of clinicians and doctors with machines. This would bring about a futuristic health revolution with improved, precise, and more efficient care and treatment.