Step 5: Identify Potential Data Sources and Instrumentation Strategy
The next step is to brainstorm with the business stakeholders what data you might need to make the predictions identified in Step 4. To facilitate the data sources brainstorming, we simply add the phrase "and what data might you need to make that prediction?" to the prediction statement.
For example:
- What will revenues and profits likely be next year…and what data might you need to make that prediction? The data source suggestions might include commodity price history, economic conditions, trade tariffs, fertilizer and pesticide prices, weather conditions, fuel prices, and more.
- How much fertilizer will I likely need next planting season…and what data might you need to make that prediction? The data source suggestions might include pesticide and herbicide usage history, weather conditions, crops to be planted, pest forecasts, soil conditions, and more.
We complete the brainstorming session between the business stakeholders and the data science team by creating a matrix of ranked data sources, using the aggregated judgement and experience of the business stakeholders, that estimates their potential predictive relevance for each Use Case (see Figure 2.6).
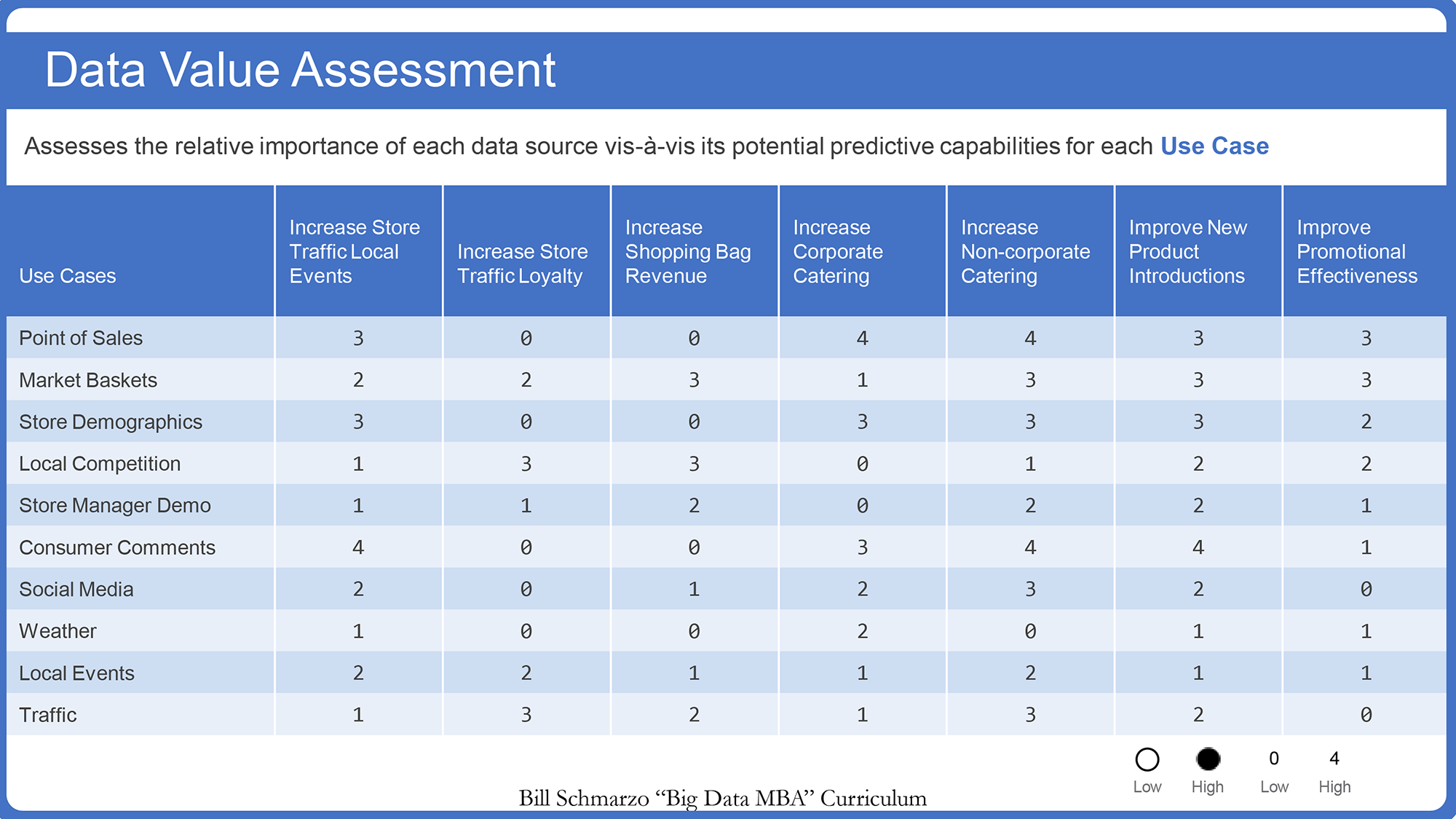
Figure 2.6: Data Value Assessment Matrix example
The data science team can then use the relative data source rankings in Figure 2.6 to start their analytic exploration process.
DEAN OF BIG DATA TIP:
Note: do not try to pass judgement on the viability of the data sources during the stakeholder brainstorming session. The data science team will have time later to determine the viability of the identified data sources.