scikit-learn
Scikit-learn was initially created by David Cournapeau in 2007 as a way to easily create machine learning models in the Python programming language. Since its inception, the library has grown immensely in popularity because of its ease of use, wide adoption within the machine learning community, and flexibility of use. scikit-learn is usually the first machine learning package that's implemented by practitioners using Python because of the large number of algorithms available for classification
, regression
, and clustering
tasks and the speed with which results can be obtained.
For example, scikit-learn's LinearRegression
class is an excellent choice if you wish to quickly train a simple regression model, whereas if a more complex algorithm is required that's capable of learning nonlinear relationships, scikit-learn's GradientBoostingRegressor
or any one of the support vector machine
algorithms are great choices. Likewise, with classification or clustering tasks, scikit-learn offers a wide variety of algorithms to choose from.
The following are a few of the advantages and disadvantages of using scikit-learn for machine learning purposes.
The advantages of scikit-learn are as follows:
- Mature: Scikit-learn is well-established within the community and used by members of the community of all skill levels. The package includes most of the common machine learning algorithms for classification, regression, and clustering tasks.
- User-friendly: Scikit-learn features an easy-to-use API that allows beginners to efficiently prototype without having to have a deep understanding or having to code each specific mode.
- Open source: There is an active open source community working to improve the library, add documentation, and release regular updates, which ensures that the package is stable and up to date.
The disadvantage of scikit-learn is as follows:
Neural network support is lacking: Estimators with ANN algorithms are minimal.
Note
You can find all the documentation for the scikit-learn library here: https://scikit-learn.org/stable/documentation.html.
The estimators in scikit-learn can generally be classified into supervised learning and unsupervised learning techniques. Supervised learning occurs when a target
variable is present. A target
variable is a variable of the dataset that you are trying to predict, given the other variables. Supervised learning
requires the target variable to be known and models are trained to correctly predict this variable. Binary classification
using logistic regression
is a good example of a supervised learning technique.
In unsupervised learning
, no target variable is given in the training data, but models aim to assign a target variable. An example of an unsupervised learning technique is k-means clustering. This algorithm partitions data into a given number of clusters based on its proximity to neighboring data points. The target
variable that's assigned may be either the cluster number or cluster center.
An example of utilizing a clustering example in practice may look as follows. Imagine that you are a jacket manufacturer and your goal is to develop dimensions for various jacket sizes. You cannot create a custom-fit jacket for each customer, so one option you have to determine the dimensions for jackets is to sample the population of customers for various parameters that may be correlated to fit, such as height and weight. Then, you can group the population into clusters using scikit-learn's k-means clustering
algorithm with a cluster number that matches the number of jacket sizes you wish to produce. The cluster-centers that are created from the clustering algorithm become the parameters that the jacket sizes are based on.
This is visualized in the following figure:
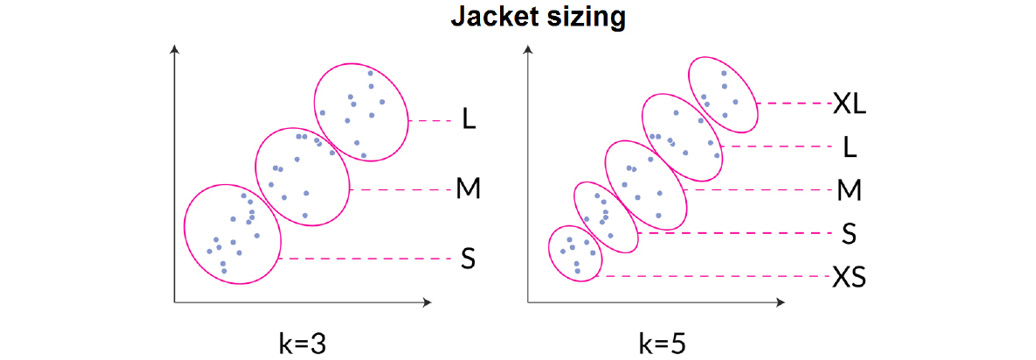
Figure 1.23: An unsupervised learning example of grouping customer parameters into clusters
There are even semi-supervised learning
techniques in which unlabeled data is used in the training of machine learning models. This technique may be used if there is only a small amount of labeled data and a copious amount of unlabeled data. In practice, semi-supervised learning produces a significant improvement in model performance compared to unsupervised learning.
The scikit-learn library is ideal for beginners as the general concepts for building machine learning pipelines can be learned easily. Concepts such as data preprocessing (the preparation of data for use in machine learning models), hyperparameter tuning (the process of selecting the appropriate model parameters), model evaluation (the quantitative evaluation of a model's performance), and many more are all included in the library. Even experienced users find the library easy to use in order to rapidly prototype models before using a more specialized machine learning library.
Indeed, the various machine learning techniques we've discussed, such as supervised and unsupervised learning, can be applied with Keras using neural networks with different architectures, all of which will be discussed throughout this book.