Advanced techniques – prefix and prompt tuning
You might be wondering; isn’t there some sophisticated way to use optimization techniques and find the right prompt, without even updating the model parameters? The answer is yes, there are many ways of doing this. First, let’s try to understand prefix tuning.
Prefix tuning
This technique was proposed (13) by a pair of Stanford researchers in 2021 specifically for text generation. The core idea, as you can see in the following diagram from their paper, is that instead of producing a net-new model for each downstream task, a less resource-intensive option is to create a simple vector for each task itself, called the prefix.
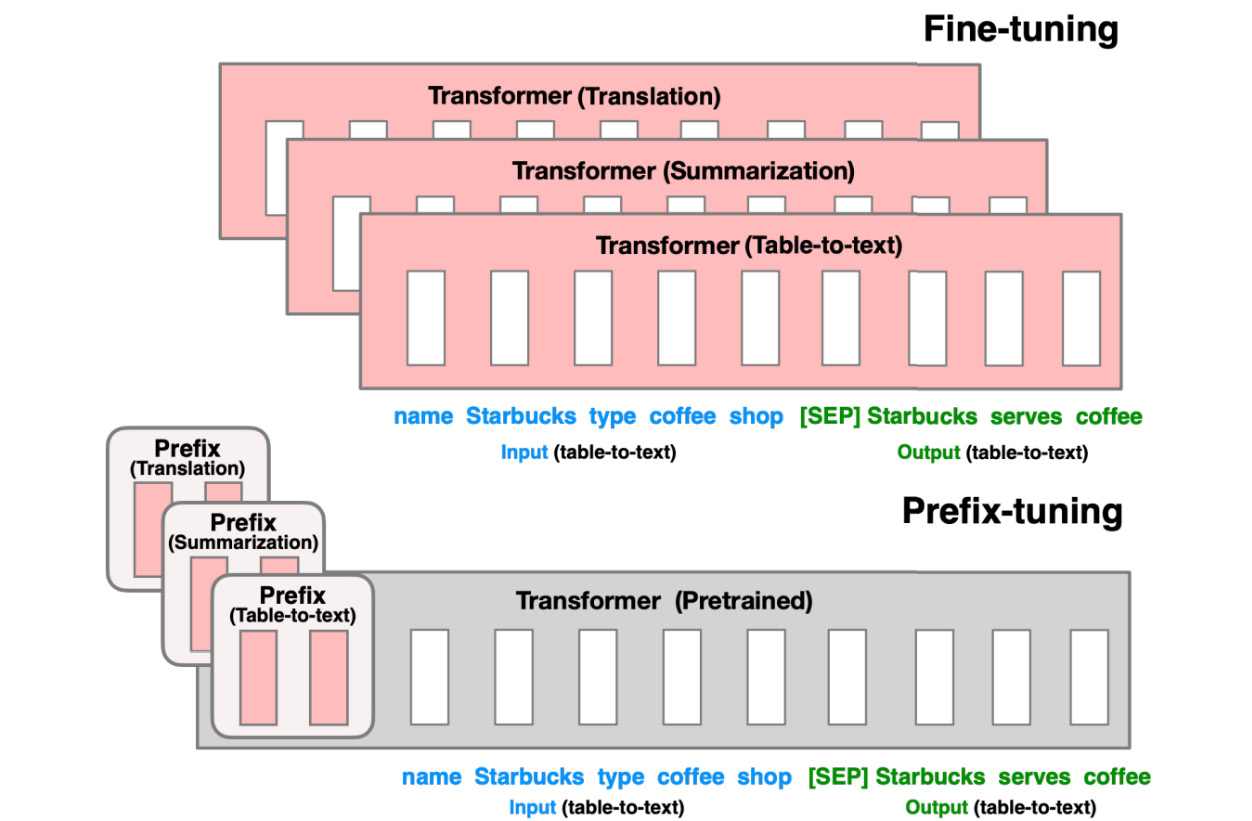
Figure 13.5 – Prefix tuning
The core idea here is that instead of fine-tuning the entire pretrained transformer for each downstream task, let’s try to update just a single vector for that task. Then, we don’t need to store all of the model...