Transformer architecture
A Transformer is a type of Seq2Seq model (discussed in the previous chapter). Transformer models can work with both image and text data. The Transformer model takes in a sequence of inputs and maps that to a sequence of outputs.
The Transformer model was initially proposed in the paper Attention is all you need by Vaswani et al. (https://arxiv.org/pdf/1706.03762.pdf). Just like a Seq2Seq model, the Transformer consists of an encoder and a decoder (Figure 10.1):
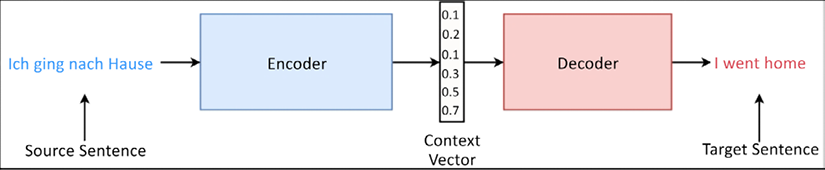
Figure 10.1: The encoder-decoder architecture
Let’s understand how the Transformer model works using the previously studied Machine Translation task. The encoder takes in a sequence of source language tokens and produces a sequence of interim outputs. Then the decoder takes in a sequence of target language tokens and predicts the next token for each time step (the teacher forcing technique). Both the encoder and the decoder use attention mechanisms to improve performance. For...