We covered the terms on- and off-policy previously when we looked at MC training in Chapter 2, Monte Carlo Methods. Recall that the agent didn't update its policy until after an episode. Hence, this defines the TD(0) method of learning in the last example as an off-policy learner. In our last example, it may seem that the agent is learning online but it still, in fact, trains a policy or Q table externally. That is, the agent needs to build up a policy before it can learn to make decisions and play the game. Ideally, we want our agent to learn or improve its policy as it plays through an episode. After all, we don't learn offline nor does any other biological animal. Instead, our goal will be to understand how an agent can learn using on-policy learning. On-policy learning will be covered in Chapter 5, Exploring SARSA.




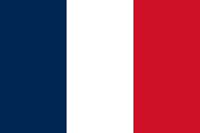


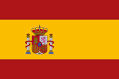




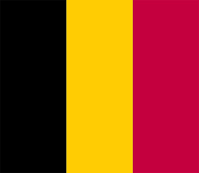
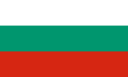
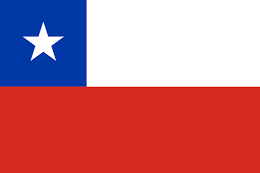
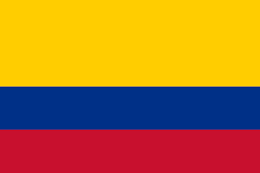
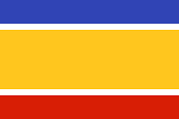

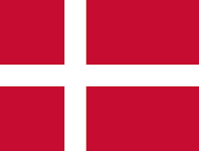



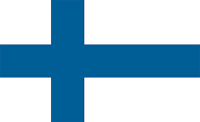

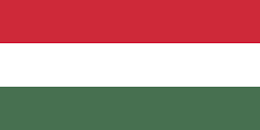






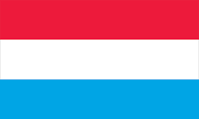
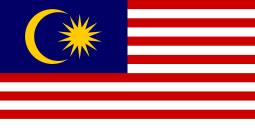

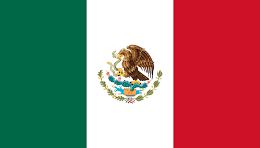

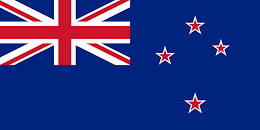







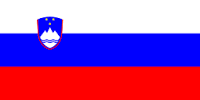




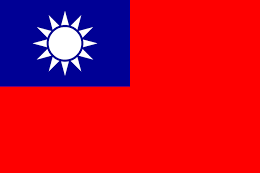


