Working with Real-World Datasets
There are plenty of open datasets available online these days. The following are some popular sources of open datasets:
Kaggle: A platform for hosting data science competitions. The official website is https://www.kaggle.com/.
UCI Machine Learning Repository: A collection of databases, domain theories, and data generators that are used by the machine learning community for the empirical analysis of machine learning algorithms. You can visit the official page via navigating to https://archive.ics.uci.edu/ml/index.php URL.
data.gov.in: Open Indian government data platform, which is available at https://data.gov.in/.
World Bank Open Data: Free and open access to global development data, which can be accessed from https://data.worldbank.org/.
Increasingly, many private and public organizations are willing to make their data available for public access. However, it is restricted to only complex datasets where the organization is looking for solutions to their data science problem through crowd-sourcing platforms such as Kaggle. There is no substitute for learning from data acquired internally in the organization as part of a job that offers all kinds of challenges in processing and analyzing.
Significant learning opportunity and challenge concerning data processing comes from the public data sources as well, as not all the data from these sources are clean and in a standard format. JSON, Excel, and XML are some other formats used along with CSV, though CSV is predominant. Each format needs a separate encoding and decoding method and hence a reader package in R. In our next section, we will discuss various data formats and how to process the available data in detail.
Throughout this chapter and in many others, we will use the direct marketing campaigns (phone calls) of a Portuguese banking institution dataset from UCI Machine Learning Repository. (https://archive.ics.uci.edu/ml/datasets/bank+marketing). The following table describes the fields in detail:
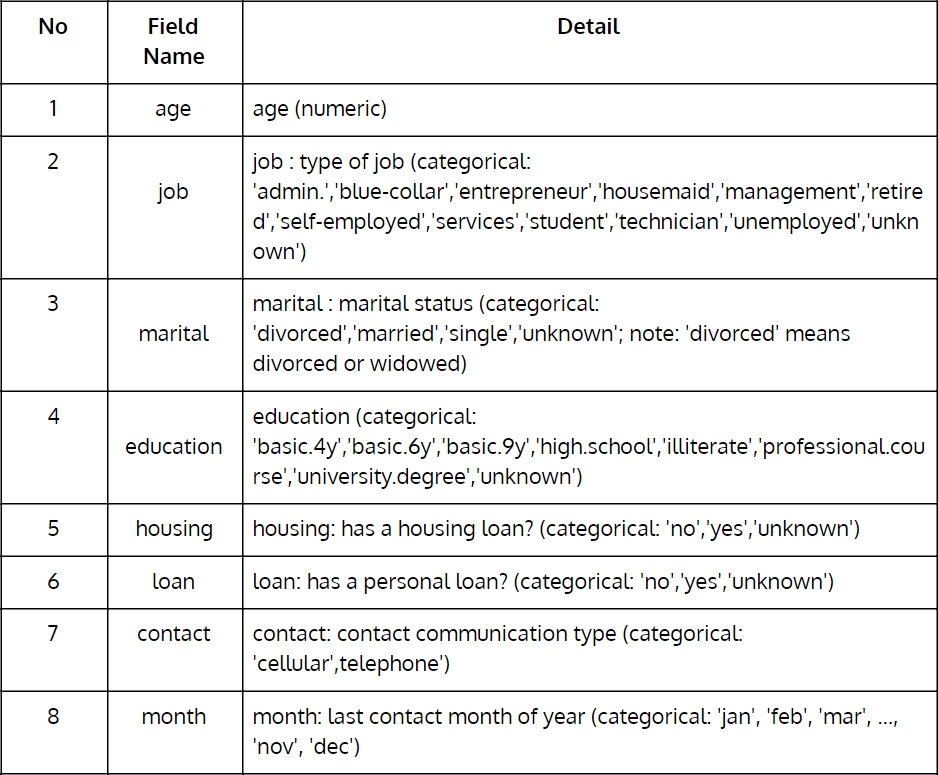
Figure 1.1: Portuguese banking institution dataset from UCI Machine Learning Repository (Part 1)
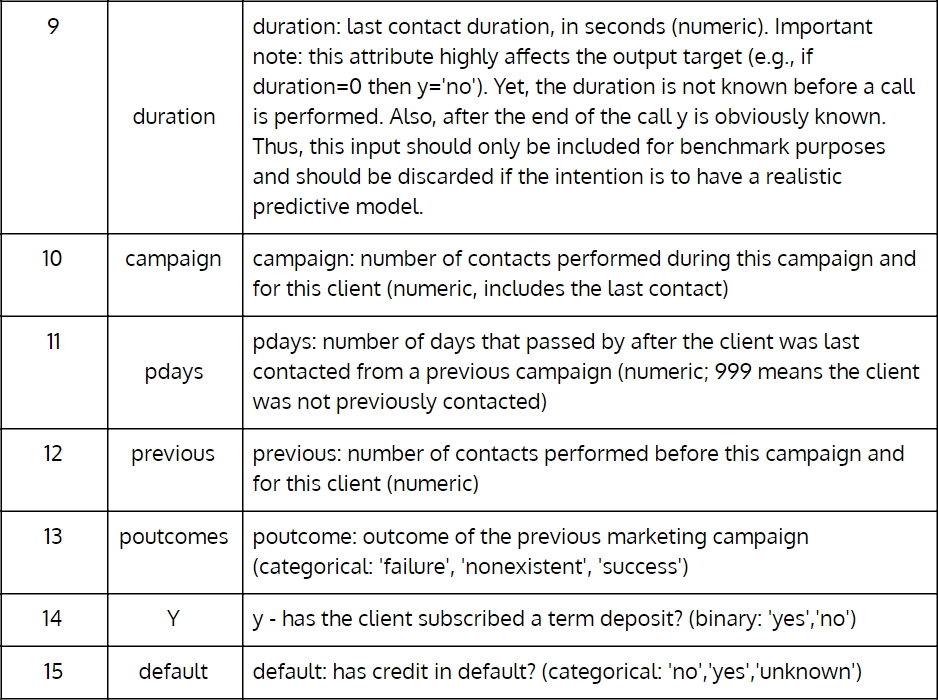
Figure 1.2: Portuguese banking institution dataset from UCI Machine Learning Repository (Part 2)
In the following exercise, we will download the bank.zip dataset as a ZIP file and unzip it using the unzip method.
Exercise 1: Using the unzip Method for Unzipping a Downloaded File
In this exercise, we will write an R script to download the Portuguese Bank Direct Campaign dataset from UCI Machine Learning Repository and extract the content of the ZIP file in a given folder using the unzip function.
Preform these steps to complete the exercise:
First, open R Studio on your system.
Now, set the working directory of your choice using the following command:
wd <- "<WORKING DIRECTORY>" setwd(wd)
Note
R codes in this book are implemented using the R version 3.2.2.
Download the ZIP file containing the datasets using the download.file() method:
url <- "https://archive.ics.uci.edu/ml/machine-learning-databases/00222/bank.zip" destinationFileName <- "bank.zip" download.file(url, destinationFileName,method = "auto", quiet=FALSE)
Now, before we unzip the file in the working directory using the unzip() method, we need to choose a file and save its file path in R (for Windows) or specify the complete path:
zipFile<-file.choose()
Define the folder where the ZIP file is unzipped:
outputDir <- wd
Finally, unzip the ZIP file using the following command:
unzip(zipFile, exdir=outputDir)
The output is as follows:
Figure 1.3: Unzipping the bank.zip file