The problem life cycle
You learned about IoT and explored its logical stack to understand more about People, Processes, Data, and Things. The core agenda of this book is to solve IoT business problems using Decision Science. Problem solving has been an art and has its origin ever since mankind evolved. I would like to introduce The Problem Life Cycle to learn how the problem keeps evolving. Understanding this topic is very essential to solve better problems in IoT.
Every industry has been trying to solve a problem. E-retail solved the problem of inconvenience in physical shopping for busy and working consumers, the printing press solved the problem of mass producing documents for the consumers, and so on. A few visionaries such as Apple Inc. have tried to solve a problem by first creating it. The iPod and iPad were devices that were a part of this revolution. The biggest challenge in solving a problem is that the problem evolves. If we take a deeper look at the problem life cycle, we can understand that the problem evolves from a Muddy to Fuzzy and finally to a Clear state and keeps repeating the cycle:
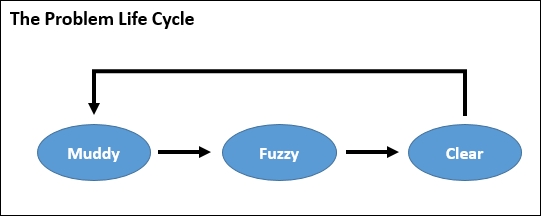
The problem life cycle
Lets take a simple example to understand this better. Consider the Marketing problem. Every organization wants to promote their products and services better by marketing them. Marketing has been a problem since ages. Lets assume that the inception of marketing happened with the invention of the printing press. Initially, the problem for marketing would be in the muddy phase, where a team of analysts would try to get the best strategy to market a product or service in place. Back then, newspapers and print media were the only medium, and the strategies and nature of the problem was very much limited to them. When the problem is new, it is in the muddy stage; we have no clear idea about how to solve it. We would try to understand the problem by experimenting and researching. Gradually, we gain some knowledge about the system and problem and then define a couple of best strategies and guidelines to solve the problem. This is when the problem evolves to the fuzzy stage. Here, the solution for the problem is still not clear, but we have a fair understanding of how to go about it. Finally, after a lot of research and experiments from a large pool of people sharing their results and understandings, we might finally have a concrete methodology in place that can be used as a complete guide to solve the problem. This is when the problem reaches the clear stage. It is the pinnacle of the problem solving methodology where we have a clear understanding about how to tackle the problem and solve it. However, one fine day, a big disruption happens and the problem that was finally in the clear state collapses and returns to the muddy stage. In the case of marketing, when people aced the best strategies to advertise using print media and newspapers, it collapsed with the invention of the radio. All of a sudden, the nature of the problem changed and it required a radically different approach to solve it. The experts, who had concrete approaches and strategies for the problem solving back then, had to revisit and start from the beginning as the problem went back to the muddy stage. The problem life cycle kept evolving, and this was repeated when television was introduced and again when social media was introduced. Today, with the social media booming and expanding to newer areas, we have the marketing problem currently stable at the fuzzy state. Soon, with the advent of virtual reality and augmented reality, it is expected to roll back to the muddy phase.
To get more real, lets relate the scenario with a more recent version of the problem. Consider a social media analyst trying to solve a problem: optimizing targets for sponsored ads that need to be placed in the Facebook newsfeed for a user based on his behavior. If we find the user to be a football enthusiast, we would insert an ad into his newsfeed for a sportswear brand. To keep things simple, assume that we are the first ones to do this and no one has ever attempted this in history. The problem will currently be in the muddy state. So logically, there would be no references or material available over the Internet for our help and research. Our problem solving task begins by identifying the users interest. Once he has been identified as a potential user with an interest in football, we need to place a sponsored ad in his newsfeed. How do we discover the users interest? There are a variety of metrics that can help us discover his interests, but for simplicity, lets assume that the users interests will be identified purely by the Status Updates he posts on his wall.
We can then simply try to analyze the statuses updated by the person and define his interests. If the word Football or names of any popular football players or football teams appear more than a desired threshold, we can possibly say that he would be following football and hence would be a potential target. Based on this simple rule, we create better strategies and algorithms where our accuracy of finding the potential users can be reached with the minimum amount of time and effort. Gradually, the problem moves from the muddy stage to the fuzzy stage. We now have a good amount of understanding regarding the problem. We may not have the best and most effective solution for the problem, but we definitely have a fair idea to get started and find a solution without too much research. Over a period of time, we, and many other similar folks, conduct various experiments, publish various blogs and research papers of the results, and help others learn from our methods and experiment more. Eventually, there would be a time when we will have attempted the exhaustive solution paradigms and have the knowledge for the best and most effective solution for any sort of analysis in that domain. Finally, it reaches its pinnacle point-the clear stage.
One day, all of a sudden, Facebook and other social media giants launch a new feature. Users can now share photos along with their status updates. A radical change will be seen in the way the user will now use the social network. People tend to post more photos than text updates. All the thought-leadership frameworks and research papers and blogs that proved to be highly successful earlier now seem to be ineffective. We are not sure how to analyze photos updated by the user in order to understand his interests. Unfortunately, the problem goes back to the muddy stage. These big changes keep happening again and again. After photos, it will be videos, then audios, and so on, and the cycle keeps repeating as usual. Recently, the user behavior on social networks has dramatically changed. People post more pictures than type any comment or status updates. These photos may or may not be symbolic of the message that the user wants to convey. Sarcasm or satire may be the objective. The memes that get viral over the Internet have no clear message embedded in them. It may be sarcasm or simple smileys that the user wants to comment on. Analyzing the meaning of these images (memes) to understand the actual message that the user wants to convey with algorithms and computers to find out his interests is a challenging deal.
Hence, understanding the problem life cycle helps prepare us better for the evolution of the problem and adapt the problem solving strategies better and faster.