When doing different kinds of analyses, especially long-term ones, we might want to consider inflation. Inflation is the general rise of the price level of an economy over time. Or to phrase it differently, the reduction of the purchasing power of money. That is why we might want to decouple the inflation from the increase of the stock prices caused by, for example, the companies’ growth or development.
We can naturally adjust the prices of stocks directly, but in this recipe, we will focus on adjusting the returns and calculating the real returns. We can do so using the following formula:
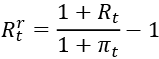
where Rrt is the real return, Rt is the time t simple return, and
stands for the inflation rate.
For this example, we use Apple’s stock prices from the years 2010 to 2020 (downloaded as in the previous recipe).
How to do it…
Execute the following steps to adjust the returns for inflation:
- Import libraries...