The Ocean Smart story
Ocean Smart is a fictitious seafood procuring, processing, distribution, and retailing company with locations scattered throughout the United States (US) and Canada that specializes in quality lobster, scallops, clams, crab, and various species of fish. Depending on the type of product and the medium by which customers would procure the product, they may go to their local supermarket to purchase live lobster, clams or crabs, fresh scallops or fish, and even "value-added" products that use any combination of the raw materials that can be processed into some sort of seafood pie, frozen and breaded fillets of fish, or a seafood medley to be served over rice or pasta. This encompasses the retail outlets from which a standard purchaser could buy a product to make a meal at home; however, it is just the beginning of where Ocean Smart-produced products may go. As we go deeper into the book, we will discuss how Ocean Smart is able to take advantage of Azure Cognitive Services to streamline operations; improve quality control (QC), customer support, engagement, and services; and predict what intermediate customers (distributors and wholesalers) and end customers will purchase.
The company was founded in the early 1980s when there was not a whole lot of regulation in the industry, and standards were dramatically different from company to company. This meant that the quality of seafood provided ranged widely depending on the source and how the product was handled in transit. Ocean Smart is just as much a success story in the world of logistics as it is in the other areas of expertise and quality standards achieved over time. This section will serve as an overview of company operations for how products are procured right through to how they are sold to end consumers and includes how the company strives to keep customers happy. We will see an overview of each of the areas in which Azure Cognitive Services has been able to help streamline operations and the other results Ocean Smart has realized because of these implementations.
As Ocean Smart decides it wants to use AI to improve operations, it evaluates the ability to build its own solutions by creating machine learning (ML) models and developing code. The company is able to wrap around those ML models and create development-operations (DevOps) and ML-operations (MLOps) practices to ensure standardization. It even goes as far as to compare public cloud vendors in what their offerings are regarding data science and related tools. What the company finds is that there is significant overhead—personnel, training, and much more—required to build all these types of solutions from scratch. Plus, there is a need to have in-house experts on business operations as well as data scientists who could either work with those experts directly or have at least some specific domain knowledge to be able to work independently to build the solutions. As a result of this discovery, Ocean Smart decides that it will be dramatically easier to use Azure Cognitive Services because of the service's ability to quickly and easily plug into any software that is running within the organization via the application programming interfaces (APIs) that are provided. This also helps the company to take advantage of the award-winning ML models that are already developed and deployed by Microsoft researchers, many of which have or are remarkably close to human parity, leading in many of the categories that all tools available in the space are compared against. The company wants to be able to compare how close to a human a machine can get in recognizing the same types of patterns, whether they be in vision, speech, text, or other areas of interest.
Now, let's take a look at a quick overview of some of the operations of the company and areas where Azure Cognitive Services can help streamline operations.
Dealing with paperwork using Knowledge Mining
Today, the company consists of boats for fishing, wharves for receiving products from its own and other fishermen, trucks for transportation of products, live, fresh, or frozen at sea, production facilities for processing of seafood, short-term holding facilities for keeping the product alive, and cold-storage facilities for longer-term storage of the products after processing into a consumable form. Each step of this process requires paperwork, comprising the following:
- Purchase receipt of the product off the boat
- Trucking paperwork, customs documents, and cost receipts for transportation
- Storage facility receiving and shipping documents
- Receiving documents at production facilities containing critical date code and weight information
- Paperwork required to capture information related to disassembly of the product and downstream production
- Shipping paperwork for transport to a storage facility or customer
- Sales information about the product when sold
- Quality reports of samples, protein levels, bacterial concerns, and so on
And this is just an idea of the amount of paperwork related to each transaction to ensure the safety of the consumers who will ultimately end up eating the product. Of course, there are also the regulatory requirements that need to be satisfied for the US Food and Drug Administration (FDA) and other governing bodies, who will want all traceability information about the products from source to table with accompanying paperwork.
When we see a glut of documents of any kind, but especially ones from a variety of sources including both typed and handwritten text, as well as documents containing images and other areas of analysis, it immediately becomes a great use case for a knowledge mining solution. Traditional knowledge mining or document management solutions are great for collecting documents, capturing some detail from within them, and adding tags and references to later be able to pull some details from the documents. However, when using Azure Cognitive Search with related cognitive services, the information that can be unlocked brings the solution to a whole different category of enterprise search. It really is the next-generation engine for relating documents, finding tone in the documents, and gathering significant details of everything that is contained within.
A knowledge mining solution is a large undertaking but produces tremendous value for the companies who are willing to take the time and implement the solution so that it spans the entire organization and becomes a resource for all. The following diagram shows a sample architecture containing some of the services that will be used alongside the Cognitive Search functions, which we will elaborate further on in the second part of the book:
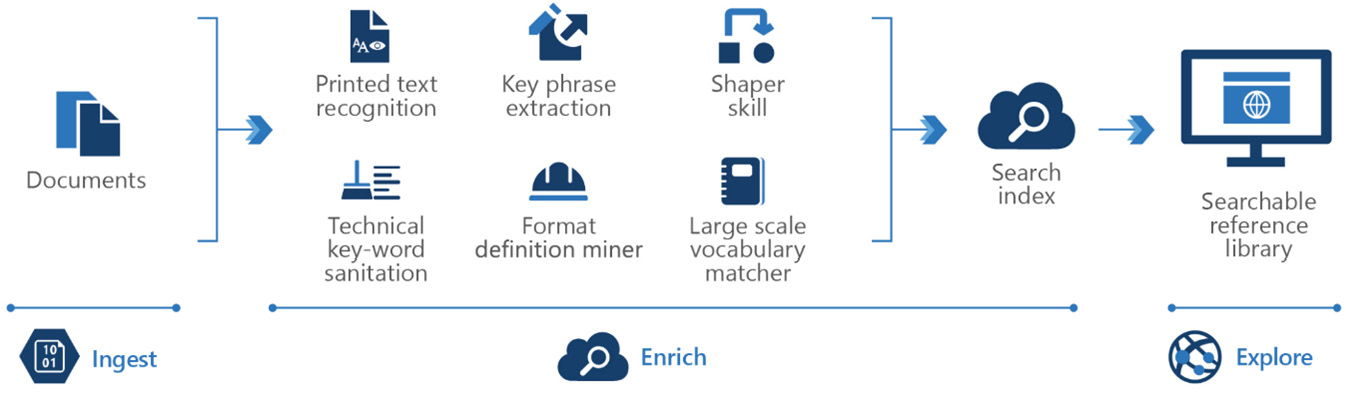
Figure 1.1 – Knowledge mining sample architecture
Next, we are going to use Form Recognizer to process financial documents.
Using Form Recognizer to process financial documents
Another area we will focus on is process- and expense-related documents for automatic processing using the Form Recognizer cognitive service. Form Recognizer allows us to capture relevant details in these documents that we can then store in a database or elsewhere for further use—for instance, processing an invoice using an accounts payable system and feeding the appropriate data we capture into the fields required, with appropriate validation steps along the way. This also helps develop an ML model in the background that can be trained to provide more accurate model training over time.
The Form Recognizer cognitive service offers the ability to streamline how documents, forms, receipts, and other assets are processed within an organization and is a key component in how robotic process automation (RPA) systems are implemented for optimized operations. Some common uses of these technologies are listed here:
- Expense report processing
- Accounts payable/accounts receivable approvals
- Other uses where data needs to be extracted from a form and feeds a system downstream
The following diagram shows a sample architecture using Form Recognizer, some related cognitive services, and other Azure services commonly deployed together to build a full solution:
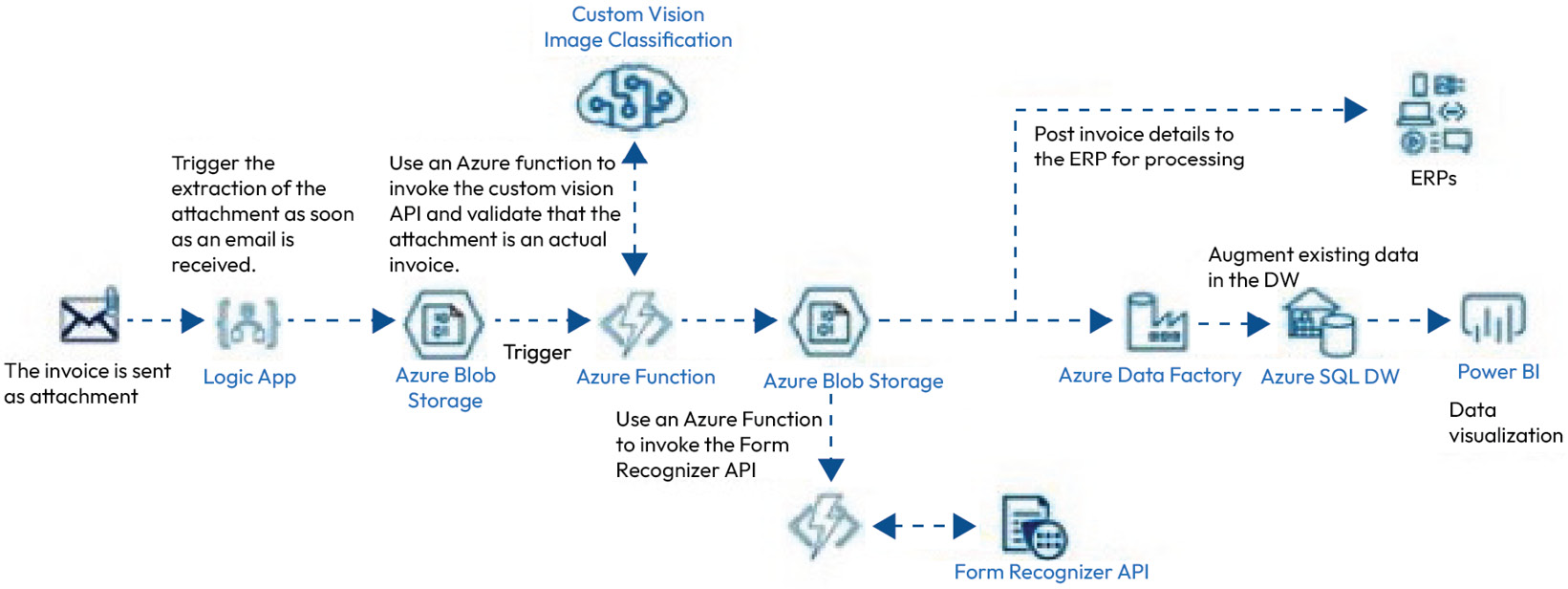
Figure 1.2 – Form Recognizer sample architecture
The next service we will discuss will help you to understand anomalous behavior among processes in your network.
Using Anomaly Detector for discovering abnormalities
The operations at Ocean Smart are complex, and overhead costs are incredibly significant, especially when it comes to the product market being among the most volatile in the whole food industry. The supply is limited to what is caught, and much more challenging to produce than a traditional farm-raised product such as cattle, pork, chicken, and other land-based animals. Ironically, the industry does not follow the traditional economic models of supply and demand. The independent fishermen are resistant to any one organization, or a small group of organizations, having control of market prices, so buying in greater volume does not necessarily mean buying prices can be reduced as a result. In a very cutthroat industry, relationships matter, but do not necessarily guarantee preference when it comes to purchasing power.
Natural disasters can wreak havoc on the entire industry and can cause significant cost fluctuations for the end consumer. Even today, you can walk into many seafood restaurants throughout the world and find seafood prices marked as Market. This volatility also causes challenges in planning what will be produced and sold at the manufacturing stage of the overall process and what can be promised to distributors. When it comes to finished goods, in whatever form (fresh, frozen, or live), however, there is much opportunity to take advantage of pricing based on supply and demand. The process by which purchase prices are settled can depend on which distributor has which product. There is a weekly, and sometimes daily even, tug of war in the industry between buyers and sellers to determine product pricing that requires significant visibility into which product is moving where and how much of each type is available. As the details have been captured over time regarding the settled prices, fishers, product type, product quality, product region, time of year, and more details, we could use the Anomaly Detector service to test if one of our parameters is somehow out of a "normal" range.
The Anomaly Detector service monitors all the data being collected and calls out areas of concern to the procurement team, giving them the ability to accept the anomaly and provide feedback to the system. Again, this will improve an ML model that has been developed over time or allow the team to go in a different direction with the transaction.
The following diagram provides a sample architecture for Anomaly Detector and other common services in Azure used alongside it for building a solution.
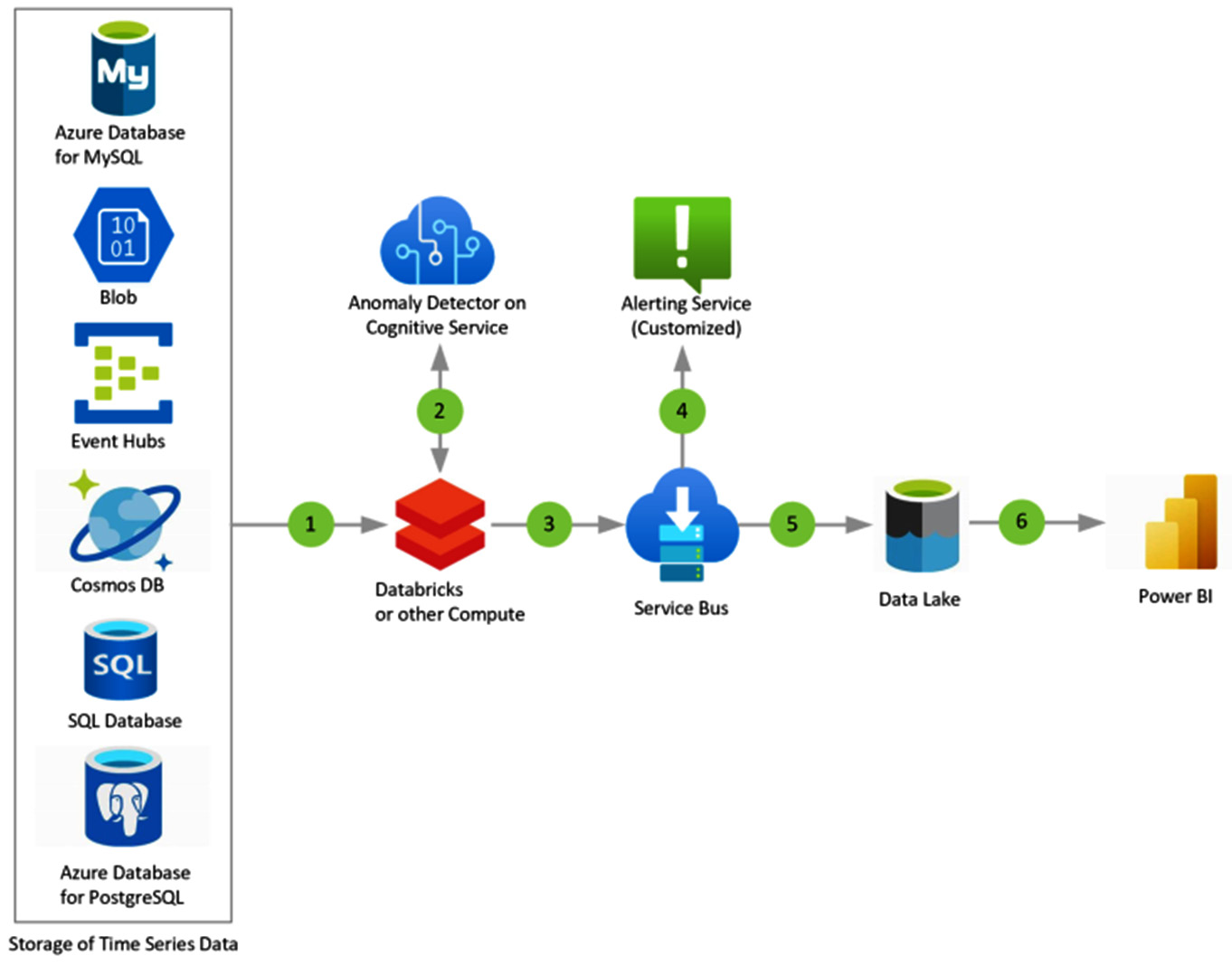
Figure 1.3 – Anomaly Detector sample architecture
The process pictured in the preceding diagram in a simplified version is outlined here:
- Data is ingested from some source in Azure.
- Some compute—in this case, Azure Databricks—is used to manipulate and prepare the data and serve it to the Anomaly Detector service for monitoring.
- When anomalous behavior is detected, an event is triggered and sent to Azure Service Bus for further action.
- An alert is sent to appropriate parties for follow-up action using the service of the developer's choosing.
- The output of the data is then logged in Azure Data Lake Storage (ADLS).
- Power BI is used to visualize the results of the logged data.
Next, we are going to discuss how human sight can be augmented using the Computer Vision service.
Using Computer Vision to detect product quality issues early
After procurement, the manufacturing (or production process, as it is commonly known in the industry) is when the raw materials are disassembled, filleted, prepared, or enhanced by adding seasonings or breading, then cooked, frozen, and packed for distribution. A key part of this process is determining the quality of the product while it is being produced. Simply put, we take the incoming weight of the product and the cost of procurement, including landing the product at a production facility, do whatever production is to be performed to add further value to the product, and understand the overall cost of goods sold as a basis for what the selling price will be. Knowing that the selling price will fluctuate depending on demand and seasonality means that we need to take considerable care when setting these prices. Of course, each of the parts has a different value, similarly to the beef industry where various parts of the animal have different values (Filet mignon is highly sought after compared to chuck steak) so, for instance, the tail of a lobster is valued significantly higher and generates more saleable product than the legs of that same lobster.
As an example, the cost of a pound of tail meat would be four to five times the cost of a pound of leg meat to a grocery store. Because of the value assigned to each of the parts, it is critical to know if the product is somehow damaged upon procurement or within the production process. A tail missing a flipper or having an obvious crack is not going to be sold at the full price but will not be thrown out either. It will either be put in a "B-grade" batch or pushed to a different location to be processed in a different way— perhaps cooking. On the other hand, if a leg were to be crushed, cracked, or similar, it would likely just be thrown away because it is not worth salvaging. This is true for many of the varieties of saleable products, and each will be handled in a way that responds to their market value.
A process for capturing quality issues at the production level will significantly help avoid downstream customer complaints and returns, meaning the product would need to be destroyed and Ocean Smart needs to provide a refund to the customer. As a result, we will employ the Custom Vision cognitive service to capture potential quality issues that get past initial QC testers. By capturing images of lobster tails or irregular scallops prior to packaging, and alerting key personnel to the concern, the downstream losses can be avoided in the future.
The Computer Vision API gives the ability to handle images in several ways. Here are just a few examples of how the service can be used:
- Classifying images based on the content and aligning with similar images
- Grabbing data from screenshots or images captured for cataloging event-related information
- Comparing captured images from cameras and comparing against "known good" images for QC purposes
The Computer Vision service has ML models already developed for image classification and other uses but we can also use Custom Vision for training and deploying custom ML models to compare against.
The following diagram shows a sample architecture of the Custom Vision service and a simplified flow of how the service processes images it receives and compares or other activities downstream:
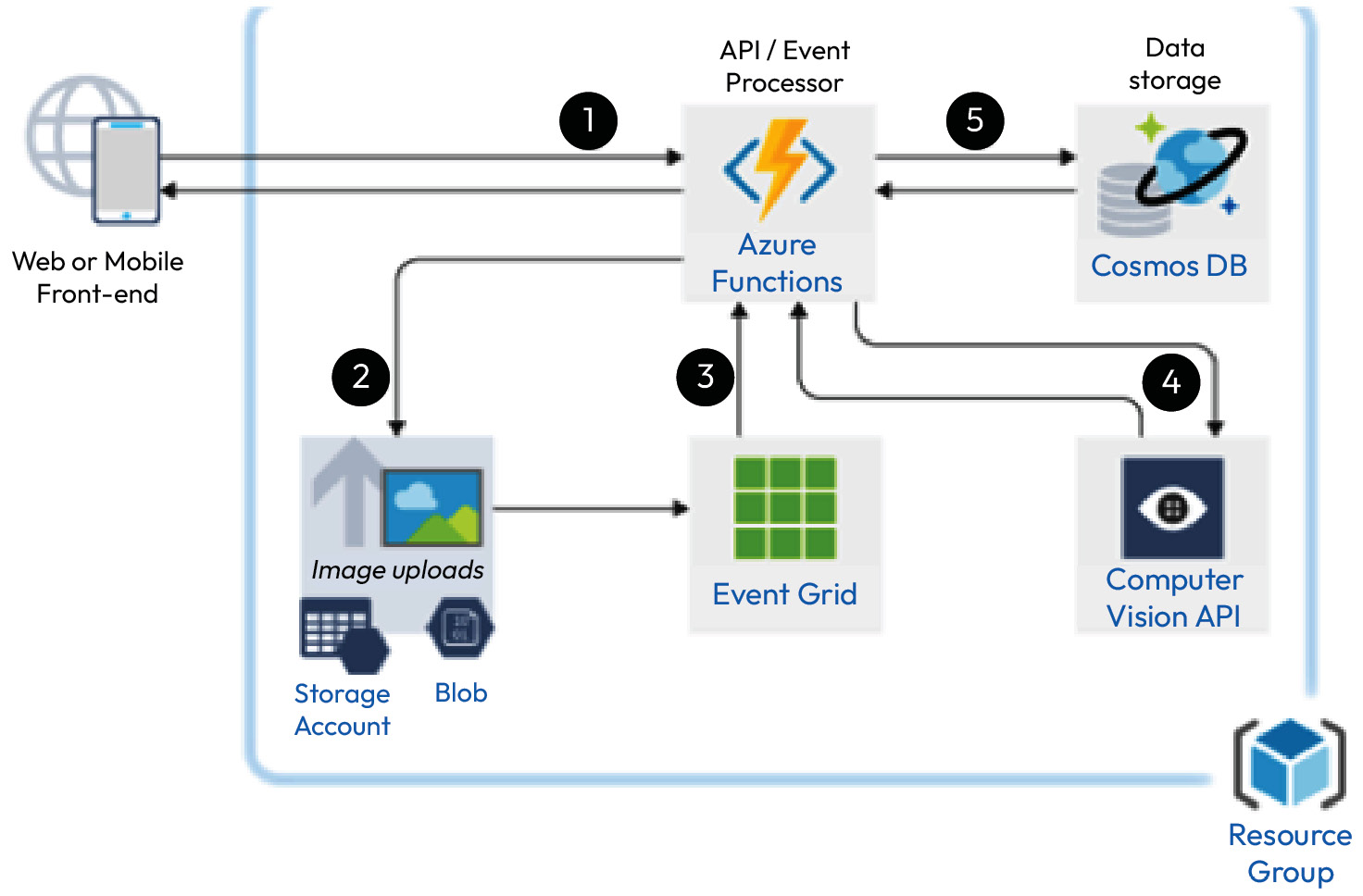
Figure 1.4 – Computer Vision sample architecture
Next, we are going to discuss how to protect corporate internet services from inappropriate content being displayed.
Applying Content Moderator to avoid the posting of inappropriate material
Once all goods are produced, they are ready for sale to a distributor, restaurant, grocery chain, fish market, via direct sales through a website, or another outlet. Ocean Smart is concerned about having a recognizable brand to consumers, and it typically runs various social campaigns and has a modern, interactive website, plus other marketing materials that will help make the brand stand out to consumers. But this comes with the caveat that the company maintains its reputation for quality. If a review of Ocean Smart's product is published in a forum somewhere or on a social networking website, positive or negative, it is important for the sales and marketing teams to be aware. The last thing the team wants to hear is that the public thinks poorly of their product or the Chief Executive Officer (CEO) comes to them with negative publicity. The company needs to be very aware of any situations that could enhance or serve as a detriment to the Ocean Smart family of brands in the market. All these channels need to be monitored for content as well as responded to in a timely manner to avoid any escalation of a negative situation. With data that is collected from social media outlets and review websites, we can use the Text Analytics cognitive service to gauge sentiment analysis in positive, neutral, or negative form, then build in automated responses as well as information being reported to customer services for appropriate action to be taken. We can also use the Content Moderator cognitive service to ensure no inappropriate content can be published to any of the Ocean Smart digital platforms or brand websites.
The Content Moderator cognitive service has three key areas that can help to moderate content: images, text, and video. The intent behind the service is to ensure that content does not get displayed on a website or other digital outlet where one of the preceding media types could be consumed by an inappropriate audience. When it is deployed, several other services can be expected to be used as part of the architecture, as follows:
- Event Hubs will capture live data streams.
- Azure Functions will parse text and content.
- Azure web services will host the application.
The architecture shown in the following diagram gives a good overview of the flow of information when the Content Moderator service is deployed for the protection of a regional chat service online:
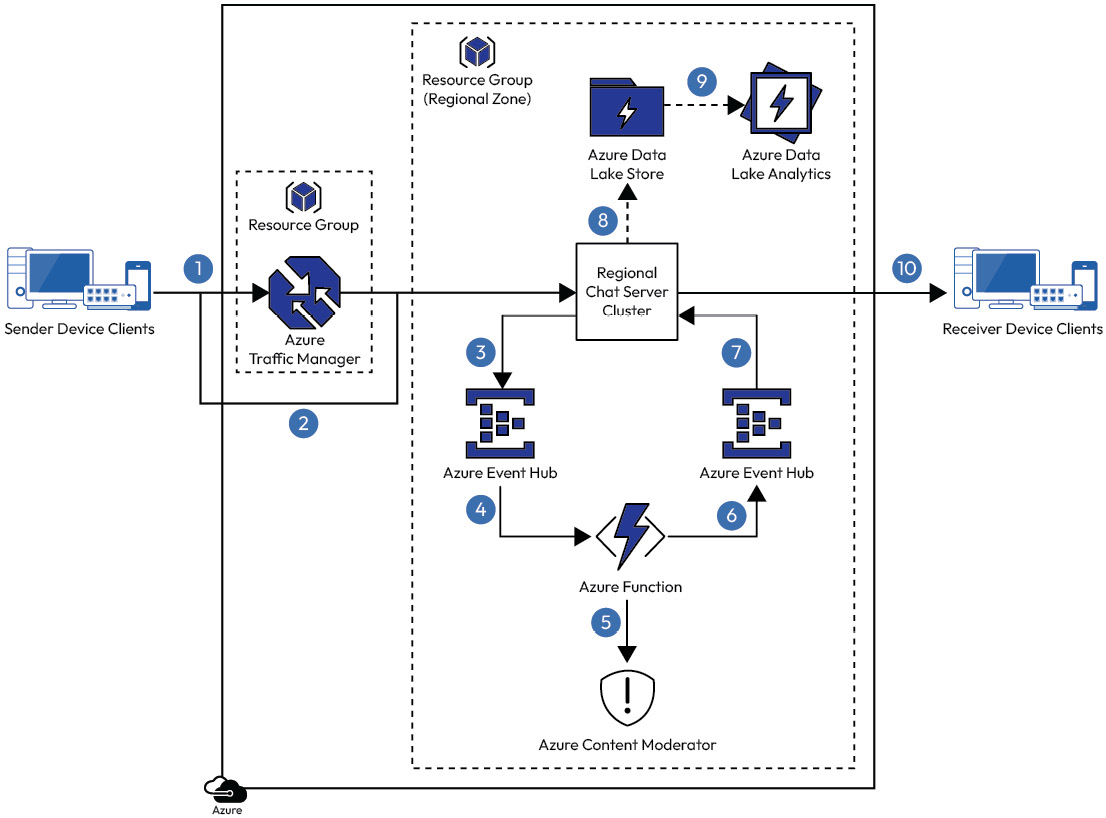
Figure 1.5 – Content Moderator sample architecture
Next, we're going to discuss a service that companies can deploy on their website for making personal recommendations to customers.
Using the Personalizer service for product recommendations
The end consumer of a product is not generally known by the team on the production floor except perhaps by the type of packaging that is being used. There are several ways a product can be packed, depending on if it is going to a distributor who will then sell the product to a restaurant, chain of restaurants, or another type of food producer. There are also products that are specifically packaged for optimal display in retail outlets such as grocery stores, fish markets, and specialty stores where the product is much more visible and presented in a way that will try to entice a consumer, as with most retail packaging.
Customers can order the product of their choice directly from Ocean Smart through its e-commerce website. As a customer makes purchases and patterns are determined, the website can either make recommendations for previously purchased products or suggestions for comparable items the customer may like based on their history. There is also a capability that allows for additional recommendations when the customer puts a product into their "cart" such as a marinade or additional product from the store that might pair well with the product they have already added to their cart.
The Azure Personalizer service is a useful tool for just this type of application. Whether looking to make recommendations for other projects or to show commonly paired products that are purchased together by customers, the Personalizer service helps to build those recommendations. The service will improve its accuracy over time, and more of a user profile is built about the consumer of the content. Also included with the service are tools for building a "mock" scenario that could be presented to a user of the site, leading to an improved recommendation.
The Personalizer cognitive service is a resource that helps organizations to align products or make recommendations for location placement on screen. These recommendations are based on optimal positioning backed by an ML model that is developed over time and offers the ability to improve the model with a feedback capability. Because the service is embedded within a web application, many of the services required will be a part of a traditional website deployment.
The architecture in the following diagram gives an overview of how the Personalizer service is used to add recommendations in real time based on what a customer has chosen to add to their cart or explored on the website:
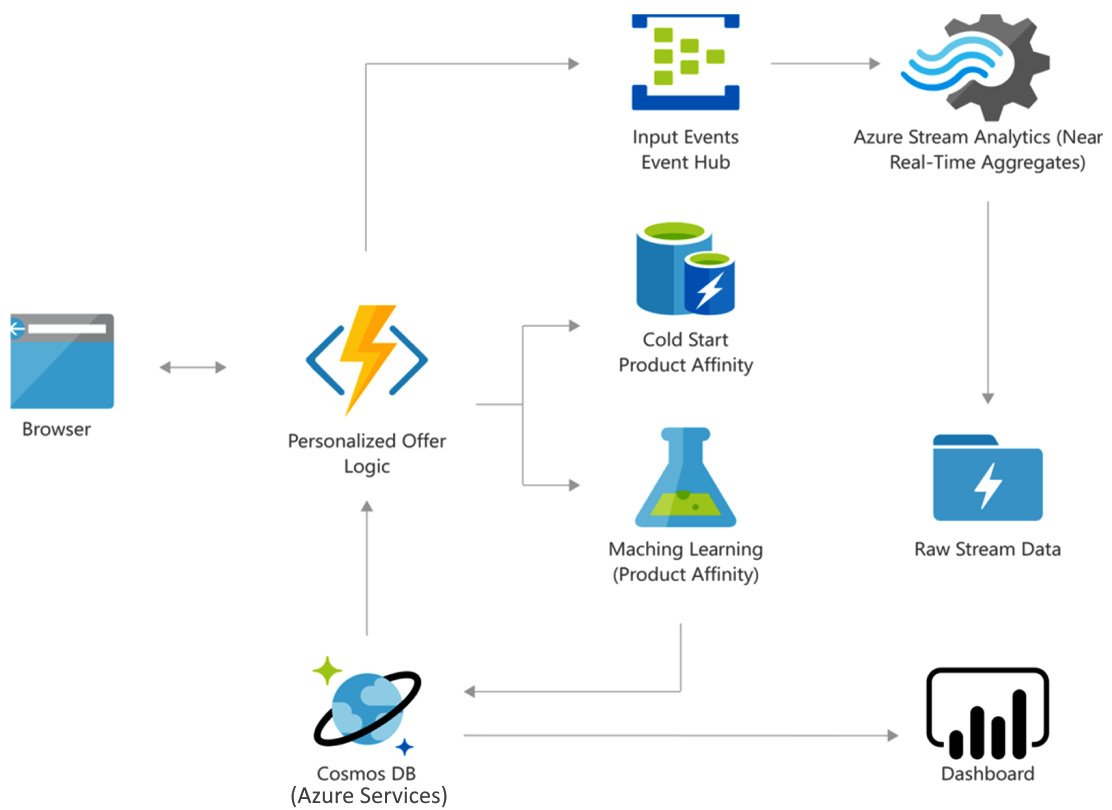
Figure 1.6 – Sample Personalizer service architecture
By implementing the Personalizer service, there was a 15% increase in profit from website sales at Ocean Smart due to the paired items that were offered and new items the customer had not purchased in the past when these were suggested. This increase in profit also drove the direct sales teams to start making similar suggestions when making sales to their customers to also increase sales. We will discuss this in more detail in Chapter 12, Using Personalizer to Cater to Your Audience.
Next, we will explore some of the options and capabilities you have when applying Speech cognitive services to your organization.
Applying Speech services for call center improvements
With any quality product being sold, there needs to be a reputation for backing that product with quality customer service. In the case of Ocean Smart, if a customer has a poor experience with one of its products and they contact customer services for some sort of resolution, there are several ways they can approach this. The website has a "contact us" form where a user can leave feedback for the team. There is a need to route the feedback to the correct employee or team for handling the feedback in the appropriate manner, but most important is how communication is handled where a customer complains about the quality of the product directly. Another more likely way customers will complain is by calling customer services directly.
As has become standard for corporations, Ocean Smart has an automated attendant phone system that helps to triage and route the nature of calls coming in. An example of this could be the following:
- "If you would like to let us know that your experience with our product is not quite what you expected, press 1, or say 'issue with product'."
- "If you would like to tell us how great our product is, press 2, or say 'this product is great'."
- "If you would like to make a suggestion, press 3, 'I'd like to make a suggestion'."
Each of these responses, whether verbally or through the keypad, will then route the call to a team/person or, depending on the time of day, for example, route the call to a voicemail box that is likely to be emailed to a team/person for handling during normal business hours. Using Speech cognitive services, the system also recognizes several different languages to help customers who do not speak English fluently, further enhancing the customer service experience. There has been considerable care in the way these types of concerns can be expressed so that customers feel as though they are being heard by the company and that the company will make it right. Ocean Smart is using the following services together to build a "call center" reference architecture:
- Speech-to-text
- Language Understanding Intelligent Service (LUIS)
- Text-to-speech
- Sentiment
- Translator
By employing this group of services, Ocean Smart can greatly enhance the customer service experience and maintain brand loyalty.
The Speech services can be applied to a host of activities related to the translation of speech, speech-to-text, text-to-speech, and language recognition activities such as keyword, speaker, and intent from recorded or real-time audio. There are many existing use cases for how speech services can be used, so the example later in Part 3, The other Cognitive Services that will help your company optimize operations, of this book will cover a call center reference architecture for improving the customer service experience.
The following diagram shows a common architecture deployed when working with the Speech services for transcribing call center activities into a text-readable format. These files are stored in Blob storage for use with later activities such as sentiment analysis, translation, and other related services downstream:
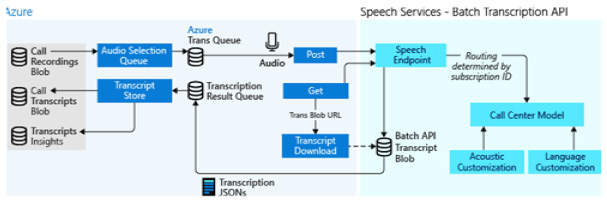
Figure 1.7 – Speech services sample architecture
LUIS is used to take natural language and process it to literally put words into action. Rudimentary versions of this service involve calling an automated phone system and being prompted "Say yes or no", for example. Depending on the response, the caller is then prompted to respond to the next question as the flow of information continues within the automated system until the caller is routed to the correct person, department, answering service, and so on. The following diagram shows a reference architecture representative of a solution that can be used to deploy an intelligent chatbot with related LUIS services:
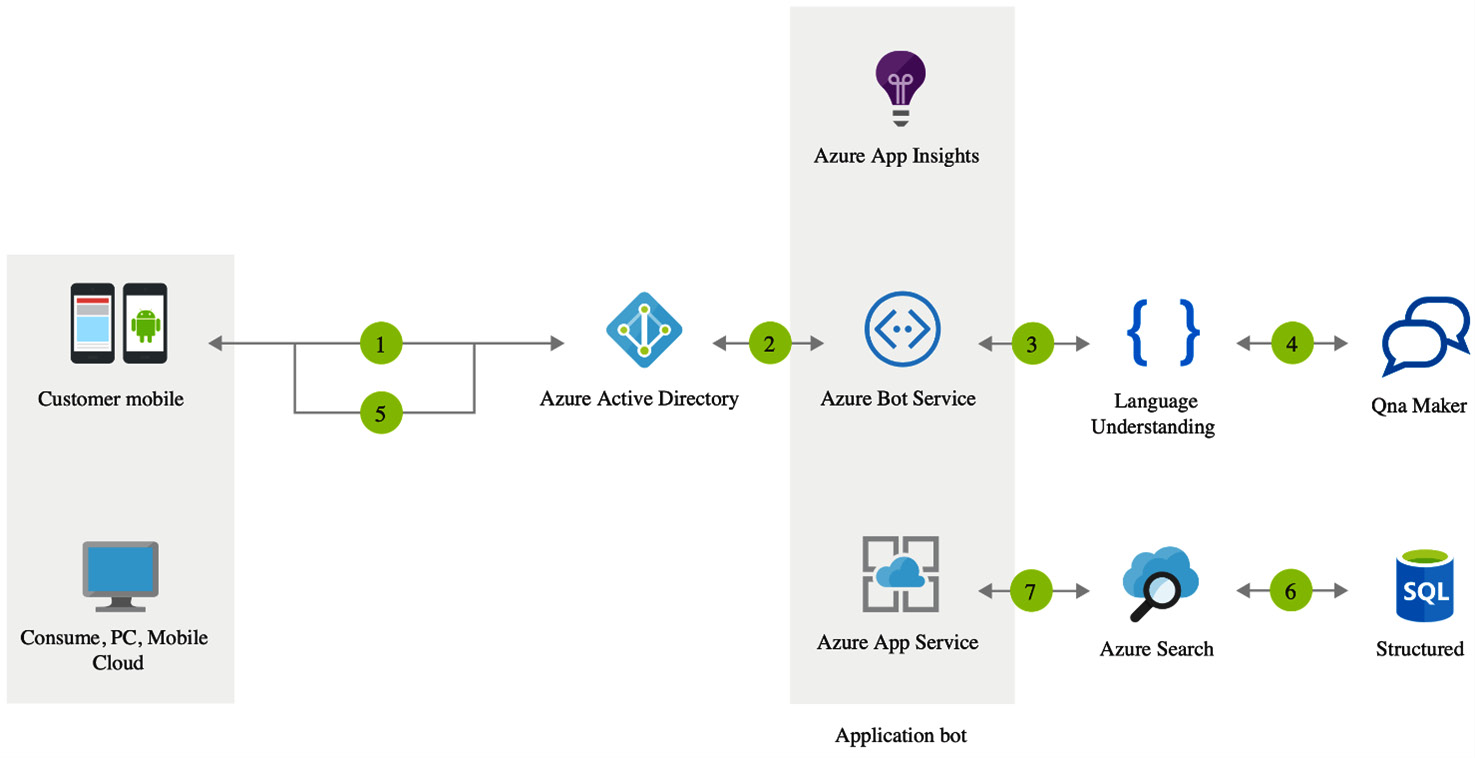
Figure 1.8 – LUIS sample architecture
Next, let's look at how we can calculate ROI