-
The main use is dimensionality reduction, to force the network to learn important features, making it possible to reconstruct the original input. The downside of AE is that the latent space represented by the hidden layer is not continuous, making it hard to sample since the decoder won't be able to make sense of some of the points.
- The reconstruction loss penalizes the network when it creates outputs that are different from the input.
- In VAE, the latent space is continuous and smooth, making it possible to sample any point of the space and interpolate between two points. It is achieved by having the latent variables follow a probability distribution of P(z), often a Gaussian distribution.
- The KL divergence measures how much two probability distributions diverge from each other. When combined with the reconstruction loss...




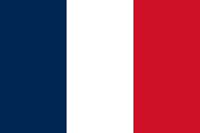


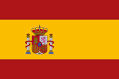



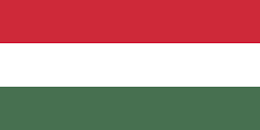

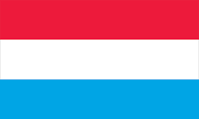





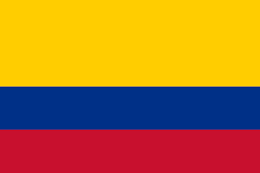
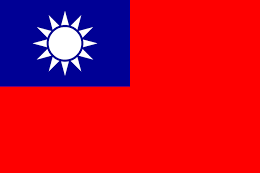
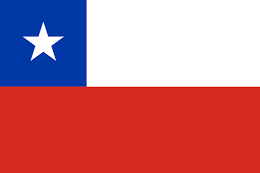



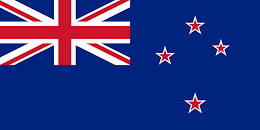
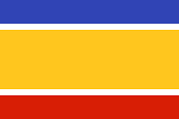
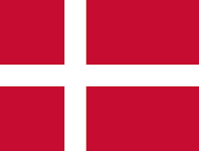
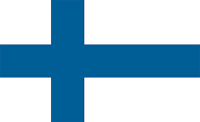







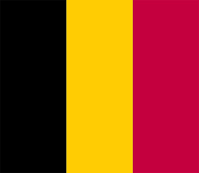

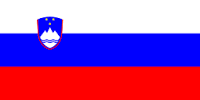





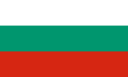


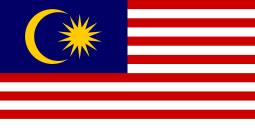



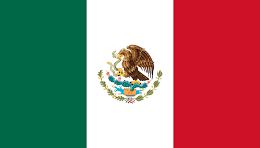
