Understanding ML and its life cycle
At its core, ML is a process that uses computer algorithms to automatically discover the underlying patterns and trends in a dataset (which is a collection of observations with features, also known as variables), make a prediction, obtain the error measure against a ground truth (if provided), and "learn" from the error with an optimization process in order to make a prediction next time. At the end of the process, an ML model is fitted or trained so that it can be used to apply the knowledge it learned to apply a decision based on the features of a new observation. The first part, generating a model, is called training, while the second part is called prediction or inference.
There are three basic types of ML algorithms based on the way the training process takes place – supervised learning, unsupervised learning, and reinforcement learning. A supervised learning algorithm is given a set of observations with a ground truth from the past. A ground truth is a key ingredient to train a supervised learning algorithm, as it drives how the model learns and makes future predictions – hence the "supervised" in the name, as the learning is supervised by the ground truth. Unsupervised learning, on the other hand, does not require a ground truth for the observations to learn how to apply the prediction. It finds patterns and relationships solely based on the features of the observations. However, a ground truth, if it exists, would still help us validate and understand the accuracy of the model in the case of unsupervised learning. Reinforcement learning, often abbreviated as RL, has quite a different learning paradigm compared to the previous two. RL consists of an agent interacting with an environment with a set of actions, and corresponding rewards and states. The learning is not guided by a ground truth, rather by optimizing cumulative rewards with actions. The trained model in the end would be able to perform actions autonomously in an environment that would achieve the best rewards.
An ML life cycle
Now we have a basic understanding of what ML is, we can go broader to see what a typical ML life cycle looks like, as illustrated in the following figure:
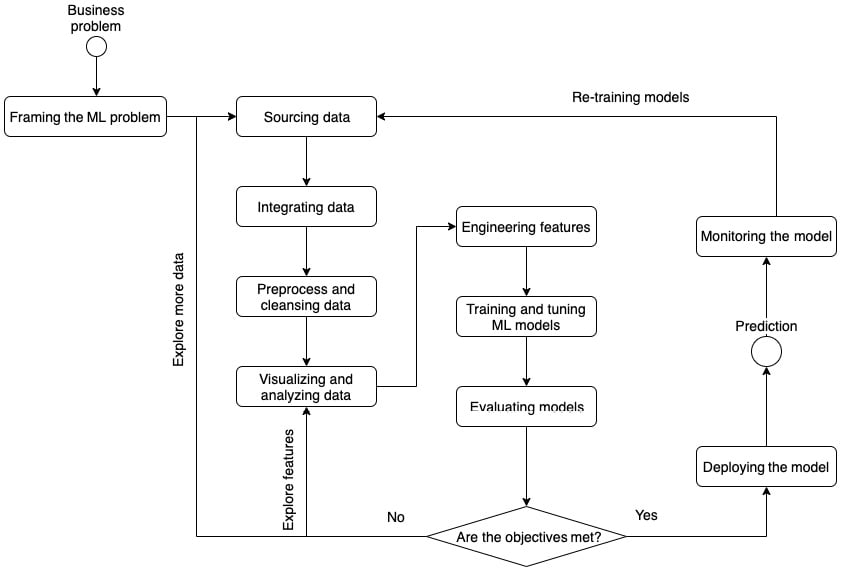
Figure 1.1 – The ML life cycle
Problem framing
The first step in a successful ML life cycle is framing the business problem into an ML problem. Business problems come in all shapes and forms. For example, "How do we increase sales of a newly released product?" and "How do we improve the QA Quality Assessment (QA) throughput on the assembly line?" Business problems such as these, usually qualitative, are not something ML can be directly applied to. But looking at the business problem statement, we should think about how it can be translated into an ML problem. We should ask questions like the following:
- "What are the key factors to the success of product sales?"
- "Who are the people that are most likely to purchase the product?"
- "What is the bottleneck in throughput in the assembly line?"
- "How do we know whether an item is defective? What differentiates a defective one from a normal one?"
By asking questions like these, we start to dig into the realm of pattern recognition, a process of recognizing patterns from the data at hand. Having the right questions that can be formulated into pattern recognition, we are a step closer to framing an ML problem. Then, we also need to understand what the key metric is to gauge the success of an approach, regardless of whether we use ML or other approaches. It is quite straightforward to measure, for example, daily product sales. We can also improve sales by targeting advertisements to the people that are mostly like to convert. Then, we get questions like the following:
- "How do we measure the conversion?"
- "What are the common characteristics of the consumers who have bought this product?"
More importantly, we need to find out whether there is even a target metric for us to predict! If there are targets, we can frame the problem as an ML problem, such as predicting future sales (supervised learning and regression), predicting whether a customer is going to buy a certain product or not (supervised learning and classification), or identifying defective items (supervised learning and classification). Questions that do not have a clear target to predict would fall into an unsupervised learning task in order to apply the pattern discovered in the data to future data points. Use cases where the target is dynamic and of high uncertainty, such as autonomous driving, robotic control, and stock price prediction, are good candidates for RL.
Data exploration and engineering
Sourcing data is the first step of a successful ML modeling journey. Once we have clearly defined both our business problem and ML problem with a basic understanding of the scope of the problem – meaning, what are the metrics and what are the factors – we can start gathering the data needed for ML. Data scientists explore the data sources to find out relevant information that could support the modeling. Sometimes, the data being captured and collected within the organization is easily accessible. Sometimes, the data is available outside your organization and would require you to reach out and ask for data sharing permission.
Sometimes, datasets can be sourced from the public internet and institutions that focus on creating and sharing standardized datasets for ML purposes, which is especially true for computer vision and natural language understanding use cases. Furthermore, data can arrive through streaming from websites and applications. Connections to a database, data lake, data warehouse, and streaming source need to be set up. Data needs to be integrated into the ML platform for processing and engineering before an ML model can be trained.
Managing data irregularity and heterogeneity is the second step in the ML life cycle. Data needs to be processed to remove irregularities such as missing values, incorrect data entry, and outliers because many ML algorithms have statistical assumptions that these irregularities would violate and render the modeling ineffective (if not invalid). For example, the linear regression model assumes that an error or residual is normally distributed, therefore it is important to check whether there are outliers that could contribute to such a violation. If so, we must perform the necessary preprocessing tasks to remedy it. Common preprocessing approaches include, but are not limited to, removal of invalid entries, removal of extreme data points (also known as outliers), and filling in missing values. Data also need to be processed to remove heterogeneity across features and normalize them into the same scale, as some ML algorithms are sensitive to the scale of the features and would develop a bias towards features with a larger scale. Common approaches include min-max scaling and z-standardization (z-score).
Visualization and data analysis is the third step in the ML life cycle. Data visualization allows data scientists to easily understand visually how data is distributed and what the trends are in the data. Exploratory Data Analysis (EDA) allows data scientists to understand the statistical behavior of the data at hand, figure out the information that has predictive power to be included in the modeling process, and eliminate any redundancy in the data, such as duplicated entries, multicollinearity, and unimportant features.
Feature engineering is the fourth step in the ML life cycle. Even with the various sources from which we are collecting data, ML models oftentimes benefit from engineered features that are calculated from existing features. For example, Body Mass Index (BMI) is a well-known engineered feature, calculated using the height and weight of a person, and is also an established feature (or risk factor, in clinical terms) that predicts certain diseases rather than height or weight alone. Feature engineering often requires extensive experience in the domain and experimentation to find out what recipes are adding predictive power to the modeling.
Modeling and evaluation
For a data scientist, ML modeling is the most exciting part of the life cycle (I think so; I hope you agree with me). You've formulated the problem in the language of ML. You've collected, processed the data, and looked at the underlying trends that give you enough hints to build an ML model. Now, it's time to build your first model for the dataset, but wait – what model, what algorithm, and what metric do we use to evaluate the performance? Well, that's the core of modeling and evaluation.
The goal is to explore and find out a satisfactory ML model, with an objective metric, from all possible algorithms, feature sets, and hyperparameters. This is definitely not an easy task and requires extensive experience. Depending on the problem type (whether it's classification, regression, or reinforcement learning), data type (as in whether it's tabular, text, or image data), data distribution (is there a class imbalance or outliers?), and domain (medical, financial, or industrial), you can narrow down the choice of algorithms to a handful. With each of these algorithms, there are hyperparameters that control the behavior and performance of the algorithm on the provided data. What is also needed is a definition of an objective metric and a threshold that meets the business requirement, using the metric to guide you toward the best model. You may blindly choose one or two algorithm-hyperparameter combinations for your project, but you may not reach the optimal solution in just one or two trials. It is rather typical for a data scientist to try out hundreds if not thousands of combinations. How is that possible?
This is why establishing a streamlined model training and evaluation process is such a critical step in the process. Once the model training and evaluation is automated, you can simply launch the process that helps you automatically iterate through the experimentations among algorithms and hyperparameters, and compare the metric performance to find out the optimal solution. This process is called hyperparameter tuning or hyperparameter optimization. If multiple algorithms are the subject of tuning, it can also be called multi-algorithm hyperparameter tuning.
Production – predicting, monitoring, and retraining
An ML model needs to be put in use in order to have an impact on the business. However, the production process is different from that of a typical software application. Unlike other software applications where business logic can be pre-written and tested exhaustively with edge cases before production, there is no guarantee that once the model is trained and evaluated, it will be performing at the same level in production as in the testing environment. This is because ML models use probabilistic, statistical, and fuzzy logic to infer an outcome for each incoming data point, and the testing, that is, the model evaluation, is typically done without true prior knowledge of production data. The best a data scientist can do prior to production is to create training data from a sample that closely represents real-world data, and evaluate the model with an out-of-sample strategy in order to get an unbiased idea of how the model would perform on unseen data. While in production, the incoming data is completely unseen by the model; how to evaluate live model performance, and how to take actions on that evaluation, are critical topics for productionizing ML models.
Model performance can be monitored with two approaches. One that is more straightforward is to capture the ground truth for the unseen data and compare the prediction against the ground truth. The second approach is to use the drift in data as a proxy to determine whether the model is going to behave in an expected way. In some use cases, the first approach is not feasible, as the true outcome (the ground truth) may lag behind the event for a long time. For example, in a disease prediction use case, where the purpose of ML modeling is to help a healthcare provider to find a likely outcome in the future, say three months, with current health metrics, it is not possible to gather a true ground truth less than three months or even later, depending on the onset of the disease. It is, therefore, impractical to only fix the model after obtaining it, should it be proven ineffective.
The second approach lies in the premise that an ML model learns statistically and probabilistically from the training data and would behave differently when a new dataset with different statistical characteristics is provided. A model would return gibberish when data does not come from the same statistical distribution. Therefore, by detecting the drift in data, it gives a more real-time estimate of how the model is going to perform. Take the disease prediction use case once again as an example: when data about a group of patients in their 30s is sent to an ML model that is trained on data with an average age of 65 for prediction, it is likely that the model is going to be clueless about these new patients. So we need to take action.
Retraining and updating the model makes sure that it stays performant for future data. Being able to capture the ground truth and detecting the data drift helps create a retraining strategy at the right time. The data that has drifted and the ground truth are the great input into the retraining process, as they will help the model to cover a wider statistical distribution.
Now that we have a clear idea of the basics of the uses and life cycle of ML development, let's take the next step and investigate how it can work with the cloud.