Improving data quality at the source
An Experian report conducted in 2021 found that 95% of business leaders reported a negative impact on their business due to poor quality data.10 This underscores the necessity for proactive measures to improve the quality of the data.
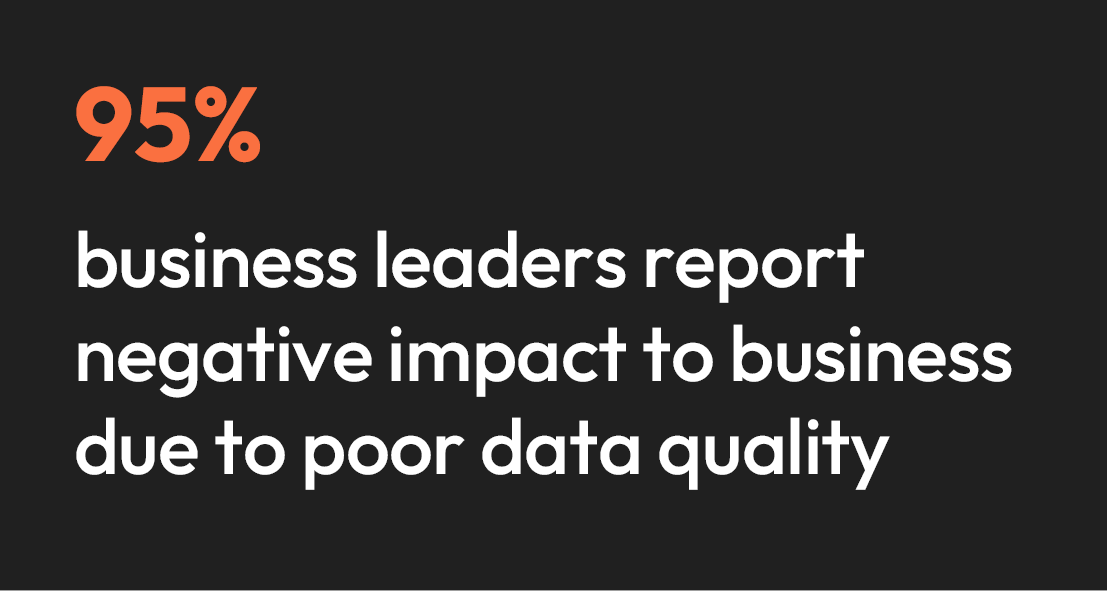
Data quality can only be improved at source. If the data source fails to capture information accurately, rectifying it later becomes futile. Similarly, inaccessible data sources can affect user access. If data is delivered infrequently, its timeliness cannot be retroactively improved. Likewise, if data sets are incomplete at the source, there’s nothing you can do to make them complete later.
You can try to work around some of these data quality issues downstream, typically in your data pipelines. For example, you could impute missing values using averages, the most common values, or machine learning algorithms, but these may be inaccurate, introduce bias, and be expensive to compute...