Writing better tests and benchmarks with pytest-benchmark
The Unix time
command is a versatile tool that can be used to assess the running time of small programs on a variety of platforms. For larger Python applications and libraries, a more comprehensive solution that deals with both testing and benchmarking is pytest
, in combination with its pytest-benchmark
plugin.
In this section, we will write a simple benchmark for our application using the pytest
testing framework. For those who are, the pytest
documentation, which can be found at http://doc.pytest.org/en/latest/, is the best resource to learn more about the framework and its uses.
You can install pytest
from the console using the pip install pytest
command. The benchmarking plugin can be installed, similarly, by issuing the pip install pytest-benchmark
command.
A testing framework is a set of tools that simplifies writing, executing, and debugging tests, and provides rich reports and summaries of the test results. When using the pytest
framework, it is recommended to place tests separately from the application code. In the following example, we create a test_simul.py
file that contains the test_evolve
function:
from simul import Particle, ParticleSimulator def test_evolve(): particles = [ Particle( 0.3, 0.5, +1), Particle( 0.0, -0.5, -1), Particle(-0.1, -0.4, +3) ] simulator = ParticleSimulator(particles) simulator.evolve(0.1) p0, p1, p2 = particles def fequal(a, b, eps=1e-5): return abs(a - b) < eps assert fequal(p0.x, 0.210269) assert fequal(p0.y, 0.543863) assert fequal(p1.x, -0.099334) assert fequal(p1.y, -0.490034) assert fequal(p2.x, 0.191358) assert fequal(p2.y, -0.365227)
The pytest
executable can be used from the command line to discover and run tests contained in Python modules. To execute a specific test, we can use the pytest path/to/module.py::function_name
syntax. To execute test_evolve
, we can type the following command in a console to obtain simple but informative output:
$ pytest test_simul.py::test_evolve platform linux -- Python 3.5.2, pytest-3.0.5, py-1.4.32, pluggy-0.4.0 rootdir: /home/gabriele/workspace/hiperf/chapter1, inifile: plugins: collected 2 items test_simul.py . =========================== 1 passed in 0.43 seconds ===========================
Once we have a test in place, it is possible for you to execute your test as a benchmark using the pytest-benchmark
plugin. If we change our test
function so that it accepts an argument named benchmark
, the pytest
framework will automatically pass the benchmark
resource as an argument (in pytest
terminology, these resources are called fixtures). The benchmark
resource can be called by passing the function that we intend to benchmark as the first argument, followed by the additional arguments. In the following snippet, we illustrate the edits necessary to benchmark the ParticleSimulator.evolve
function:
from simul import Particle, ParticleSimulator def test_evolve(benchmark): # ... previous code benchmark(simulator.evolve, 0.1)
To run the benchmark, it is sufficient to rerun the pytest test_simul.py::test_evolve
command. The resulting output will contain detailed timing information regarding the test_evolve
function, as shown here:
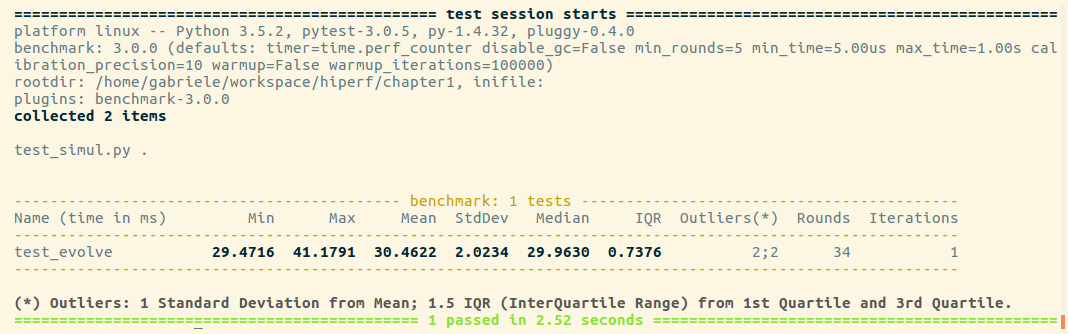
Figure 1.4 – Output from pytest
For each test collected, pytest-benchmark
will execute the benchmark
function several times and provide a statistic summary of its running time. The preceding output shown is very interesting as it shows how running times vary between runs.
In this example, the benchmark in test_evolve
was run 34
times (Rounds
column), its timings ranged between 29
and 41
milliseconds (ms) (Min
and Max
), and the Average
and Median
times were fairly similar at about 30
ms, which is actually very close to the best timing obtained. This example demonstrates how there can be substantial performance variability between runs and that, as opposed to taking timings with one-shot tools such as time
, it is a good idea to run the program multiple times and record a representative value, such as the minimum or the median.
pytest-benchmark
has many more features and options that can be used to take accurate timings and analyze the results. For more information, consult the documentation at http://pytest-benchmark.readthedocs.io/en/stable/usage.html.