In the traditional approach of solving text-related problems, we would one-hot encode the word. However, if the dataset has thousands of unique words, the resulting one-hot-encoded vector would have thousands of dimensions, which is likely to result in computation issues. Additionally, similar words will not have similar vectors in this scenario. Word2Vec is an approach that helps us to achieve similar vectors for similar words.
To understand how Word2Vec is useful, let's explore the following problem.
Let's say we have two input sentences:
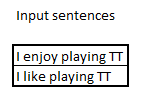
Intuitively, we know that enjoy and like are similar words. However, in traditional text mining, when we one-hot encode the words, our output looks as follows:
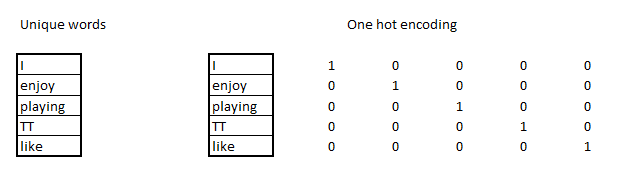
Notice that one-hot encoding results in each word being assigned a column. The major issue with one-hot encoding such as this is that the Eucledian distance...