Model underfitting and overfitting
In machine learning, the ultimate goal is to build a model that can generalize well on unseen data. However, sometimes, a model can fail to achieve this goal due to either underfitting or overfitting.
Underfitting occurs when a model is too simple to capture the underlying patterns in the data. In other words, the model can’t learn the relationship between the features and the target variable properly. This can result in poor performance on both the training and testing data. For example, in Figure 3.4, we can see that the model is underfitted, and it cannot present the data very well. This is not what we like in machine learning models, and we usually like to see a precise model, as shown in Figure 3.5:
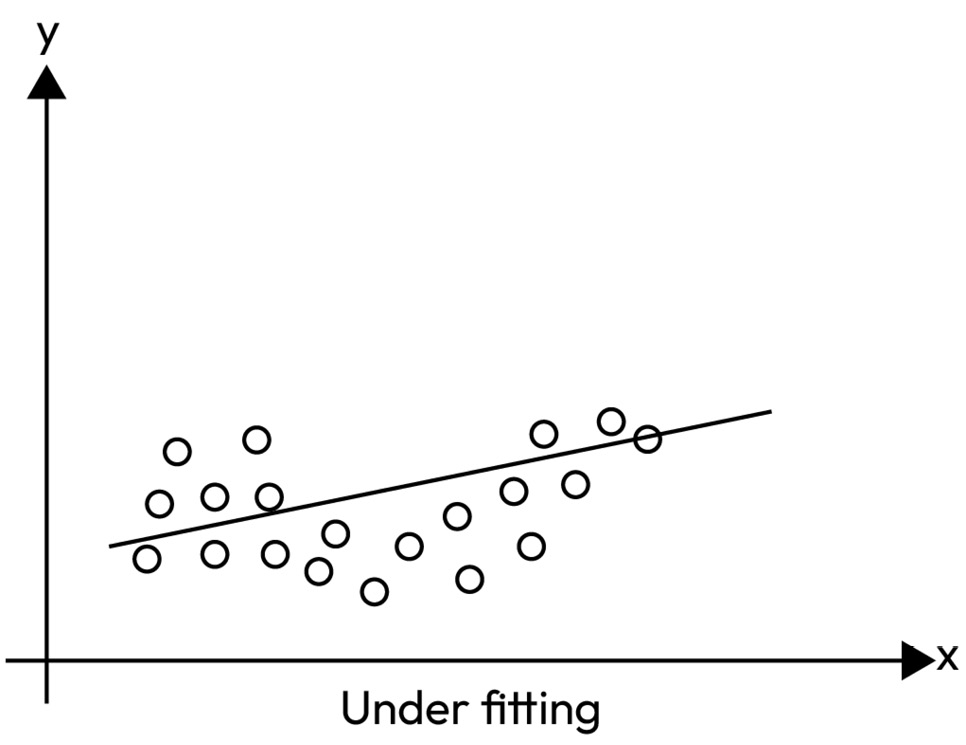
Figure 3.4 – The machine learning model underfitting on the training data
Underfitting happens when the model is not trained well, or the model complexity is not enough to catch the underlying pattern...