Understanding ML
In traditional computer programming, a human programmer must write a clear set of instructions in order for a computer program to perform an operation or provide an answer to a question. In ML, however, a human (usually an ML engineer or data scientist) uses data and an algorithm to determine the best set of parameters for a model to yield answers or predictions that are usable. While traditional computer programs provide answers using exact logic (Yes/No, Correct/Wrong), ML algorithms involve fuzziness (Yes/Maybe/No, 80% certain, Not sure, I do not know, and so on).
In other words, ML is a technique for solving problems by using data along with an algorithm, statistical model, or a neural network, to infer or predict the desired answer to a question. Instead of explicitly writing instructions on how to solve a problem, we use a bunch of examples and let the algorithm figure out the best way (the best set of parameters) to solve the problem. ML is useful when it is impossible or extremely difficult to write a set of instructions to solve a problem. A typical example problem where ML shines is computer vision (CV). Though it is easy for any normal human to identify a cat, it is impossible or extremely difficult to manually write code to identify if a given image is of a cat or not. If you are a programmer, try thinking about how you would write this code without ML. This is a good mental exercise.
The following diagram illustrates where DL and ML sit in terms of AI:
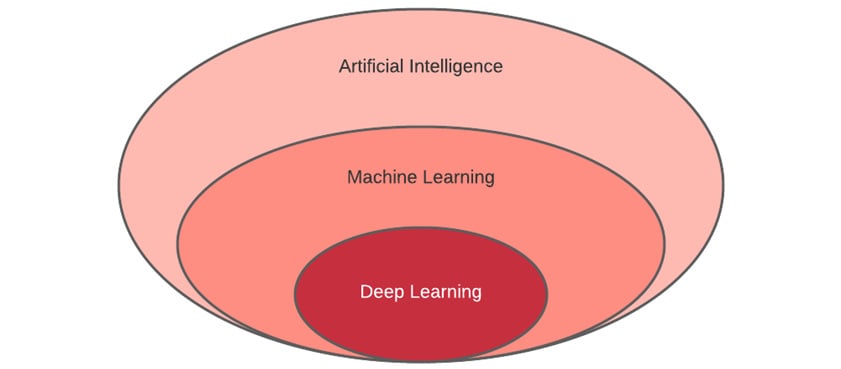
Figure 1.1 – Relationship between AI, ML, and DL
AI is a broad subject covering any basic, rule-based agent system that can replace a human operator, ML, and DL. But ML alone is another broad subject. It covers several algorithms, from basic linear regression to very deep convolutional neural networks (CNNs). In traditional programming, no matter which language or framework we use, the process of developing and building applications is the same. In contrast, ML has a wide variety of algorithms, and sometimes, they require a vastly different approach to utilize and build models from. For example, a generative adversarial network (GAN), which is an architecture used in many creative ML models to generate fake human faces, is trained differently to a basic decision tree model.
Because of the nature of ML projects, some practices in software engineering may not always apply to ML, and some practices, processes, and tools that are not present in traditional programming must be invented.