Humans in the machine learning life cycle
Developing and improving different components of a machine learning life cycle to bring a reliable and high-performance model to production is a collaborative effort that can benefit from expert and non-expert human feedback (Figure 17.1):
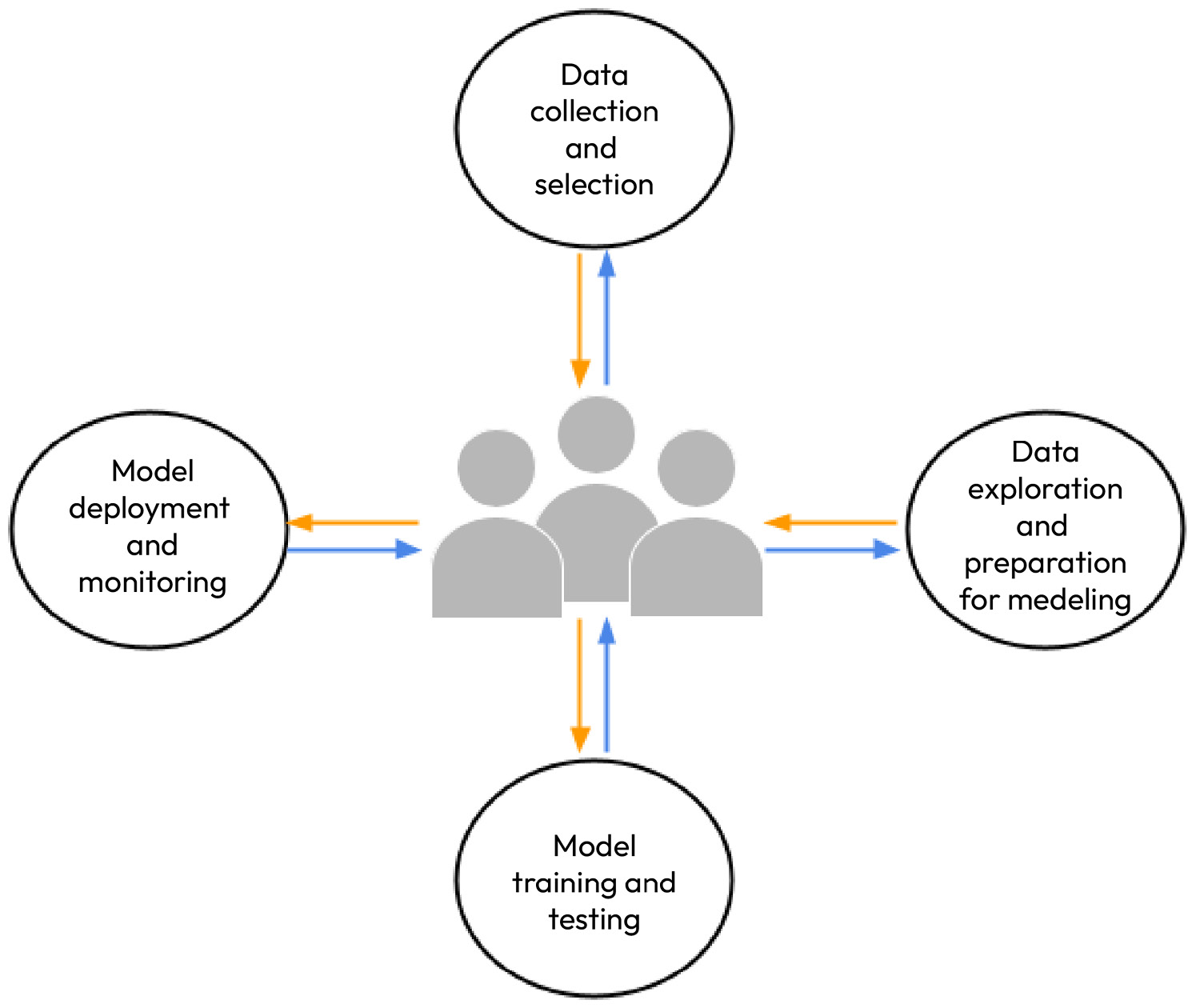
Figure 17.1 – Humans in the machine learning life cycle
For example, a radiologist can help in annotating radiological images while most people with good vision capabilities can easily label cat and dog images. But incorporating human feedback is not limited to data annotation at the beginning of a life cycle.
We can benefit from human intelligence and expertise to improve data preparation, feature engineering, and representation learning aspects of a life cycle, as well as model training and testing, and eventually model deployment and monitoring. In each of these stages, human feedback can be incorporated either passively or actively, which allows us to bring a better...