Debugging in software development
If you want to use Python and its libraries to build machine learning and deep learning models, you need to make sure your code works as expected. Let’s consider the following examples of the same function for returning the multiplication of two variables:
- Correct code:
def multiply(x, y): z = x * y return z
- Code with a typo:
def multiply(x, y): z = x * y retunr z
- Code with an indentation issue:
def multiply(x, y): z = x * y return z
- Incorrect use of
**
for multiplication:def multiply(x, y): z = x ** y return z
As you can see, there could be typos in the code and issues with indentation that prevent the code from running. You might also face issues because of an incorrect operator being used, such as **
for multiplication instead of *
. In this case, your code will run but the expected result will be different than what the function is supposed to do, which is multiplying the input variables.
Error messages in Python
Sometimes, there are issues with our code that don’t let it continue running. These issues could result in different error messages in Python. Here are some examples of error messages you might face when you’re running your Python code:
SyntaxError
: This is a type of error you’ll get when the syntax you used in your code is not the correct Python syntax. It could be caused by a typo, such as havingretunr
instead ofreturn
, as shown previously, or using a command that doesn’t exist, such as usinggiveme
instead ofreturn
.TypeError
: This error will be raised when your code tries to perform an operation on an object or variable that cannot be done in Python. For example, if your code tries to multiply two numbers while the variables are in string format instead of float or integer format.AttributeError
: This type of error is raised when an attribute is used for an object that it is not defined for. For example,isnull
is not defined for a list. So,my_list.isnull()
results inAttributeError
.NameError
: This error is raised when you try to call a function, class, or other names and modules that are not defined in your code. For example, if you haven’t defined aneural_network
class in your code but call it in your code asneural_network()
, you will get aNameError
message.IndentationError
: Python is a programming language that relies on correct indentation – that is, the necessary spaces at the beginning of each line of code – to understand relationships between the lines. It also helps with code readability.IndentationError
is the result of the wrong type of indentation being used in your code. But not all wrong indentation, based on the objective you have in mind, results inIndentationError
. For example, the following code examples work without any error, but only the first one meets the objective of counting the number of odd numbers in a list. The bottom function returns the length of the input list instead. As a result, if you run the top part of the code, you will get 3 as the output, which is the total number of odd numbers in the input list, while the bottom part of the code returns 5, which is the length of the list. These types of errors, which don’t stop the code from running but generate an incorrect output, are called logical errors.
Here is some example code in which using the wrong indention results in wrong results without any error message:
def odd_counter(num_list: list): """ :param num_list: list of integers to be checked for identifying odd numbers :return: return an integer as the number of odd numbers in the input list """ odd_count = 0 for num in num_list: if (num % 2) == 0: print("{} is even".format(num)) else: print("{} is even".format(num)) odd_count += 1 return odd_count num_list = [1, 2, 5, 8, 9] print(f'Total number of odd numbers in the list: {odd_counter(num_list)}')
The following code runs but generates unintended results:
def odd_counter(num_list: list): """ :param num_list: list of integers to be checked for identifying odd numbers :return: return an integer as the number of odd numbers in the input list """ odd_count = 0 for num in num_list: if (num % 2) == 0: print("{} is even".format(num)) else: print("{} is even".format(num)) odd_count += 1 return odd_count num_list = [1, 2, 5, 8, 9] print(f'Total number of odd numbers in the list: {odd_counter(num_list)}')
There are other errors whose meanings are clear based on their name, such as ZeroDivisionError
when your code tries to return division by zero, IndexError
if your code tries to get a value based on an index that is greater than the length of a list, or ImportError
when you’re trying to import a function or class that cannot be found.
In the previous code examples, we used docstring
to specify the type of input parameter (that is, a list) and the intended output. Having this information helps you and new users of your code to better understand the code and resolve any issue with it quickly.
These are simple examples of issues that can happen in your software and pipelines. In machine learning modeling, you need to conduct debugging to deal with hundreds or thousands of lines of code and tens or hundreds of functions and classes. However, debugging could be much more challenging compared to these examples. It could be even more difficult if you need to start working on a piece of code that you have not written yourself when, for example, you’re joining a new team in the industry or academia. You need to use techniques and tools that help you debug your code with minimum effort and time. Although this book is not designed for code debugging, reviewing some debugging techniques could help you in developing high-quality code that runs as planned.
Debugging techniques
There are techniques to help you in the process of debugging a piece of code or software. You might have used one or more of these techniques, even without remembering or knowing their names. We will review four of them here.
Traceback
When you get an error message in Python, it usually provides you with the necessary information to find the issue. This information creates a report-like message about the lines of your code that the error occurred in, as well as the types of error and function or class calls that resulted in such errors. This report-like message is called a traceback in Python.
Consider the following code, in which the reverse_multiply
function is supposed to return a list of element-wise multiplication of an input list and its reverse. Here, reverse_multiply
uses the multiply
command to multiply the two lists. Since multiply
is designed for multiplying two float numbers, not two lists, the code returns the traceback message with the necessary information for finding the issue, starting from the bottom operation. It specifies that TypeError
occurred on line 8 within multiply
, which is the bottom operation, and then lets us know that this issue results in an error occurring on line 21, in reverse_multiply
, and eventually line 27 in the whole code module. Both the PyCharm IDE and Jupyter return this information. The following code examples show you how to use traceback to find necessary information so that you can debug a small and simple piece of Python code in both PyCharm and Jupyter Notebook:
def multiply(x: float, y: float): """ :param x: input variable of type float :param y: input variable of type float return: returning multiplications of the input variables """ z = x * y return z def reverse_multiply(num_list: list): """ :param num_list: list of integers to be checked for identifying odd numbers :return: return a list containing element-wise multiplication of the input list and its reverse """ rev_list = num_list.copy() rev_list.reverse() mult_list = multiply(num_list, rev_list) return mult_list num_list = [1, 2, 5, 8, 9] print(reverse_multiply(num_list))
The following lines show you the traceback error message when you run the previous code in Jupyter Notebook:
TypeError Traceback (most recent call last) <ipython-input-1-4ceb9b77c7b5> in <module>() 25 26 num_list = [1, 2, 5, 8, 9] ---> 27 print(reverse_multiply(num_list)) <ipython-input-1-4ceb9b77c7b5> in reverse_multiply(num_list) 19 rev_list.reverse() 20 ---> 21 mult_list = multiply(num_list, rev_list) 22 23 return mult_list <ipython-input-1-4ceb9b77c7b5> in multiply(x, y) 6 return: returning multiplications of the input variables 7 """ ----> 8 z = x * y 9 return z 10 TypeError: can't multiply sequence by non-int of type 'list' Traceback error message in Pycharm Traceback (most recent call last): File "<input>", line 27, in <module> File "<input>", line 21, in reverse_multiply File "<input>", line 8, in multiply TypeError: can't multiply sequence by non-int of type 'list'
Python traceback messages seem to be very useful for debugging our code. However, they are not enough for debugging large code bases that contain many functions and classes. You need to use complementary techniques to help you in the debugging process.
Induction and deduction
When you have found an error in your code, you can either start by collecting as much information as you can and try to find potential issues using the information, or you can jump into checking your suspicions. These two approaches differentiate induction from the deduction process in terms of code debugging:
- Induction: In the induction process, you start collecting information and data about the problem in your code that helps you come up with a list of potential issues resulting from the error. Then, you can narrow the list down and, if necessary, collect more information and data from the process until you fix the error.
- Deduction: In the deduction process, you come up with a short list of your points of suspicion regarding the issues in your code and try to find if any one of them is the actual source of the issue. You continue this process and gather more information and come up with new potential sources of the problem. You continue this process until you fix the problem.
In both approaches, you go through an iterative process of coming up with potential sources of issues and building hypotheses and then collect the necessary information until you fix the error in your code. If a piece of code or software is new to you, this process could take time. In such cases, try to get help from your teammates with more experience with the code to collect more data and come up with more relevant hypotheses.
Bug clustering
As stated in the Pareto principle, named after Vilfredo Pareto, a famous Italian sociologist and economist, 80% of the results originate from 20% of the causes. The exact number is not the point here. This principle helps us better understand that the majority of the problems and errors in our code are caused by a minority of its modules. By grouping bugs, we can hit multiple birds with one stone as resolving an issue in a group of bugs could potentially resolve most others within the same group.
Problem simplification
The idea here is to simplify the code so that you can identify the cause of the error and fix it. You could replace big data objects with smaller and even synthetic ones or limit function calling in a big module. This process could help you quickly eliminate the options for identifying the causes of the issues in your code, or even in the data format you have used as inputs of functions or classes in your code. Especially in a machine learning setting, where you might deal with complex data processes, big data files, or streams of data, this simplification process for debugging could be very useful.
Debuggers
Each IDE you might use, such as PyCharm, or if you use Jupyter Notebook to experiment with your ideas using Python, has built-in features for debugging. There are also free or paid tools you can benefit from to facilitate your debugging processes. For example, in PyCharm and most other IDEs, you can use breakpoints as pausing places when running a big piece of code so that you can follow the operations in your code (Figure 1.3) and eventually find the cause of the issue:
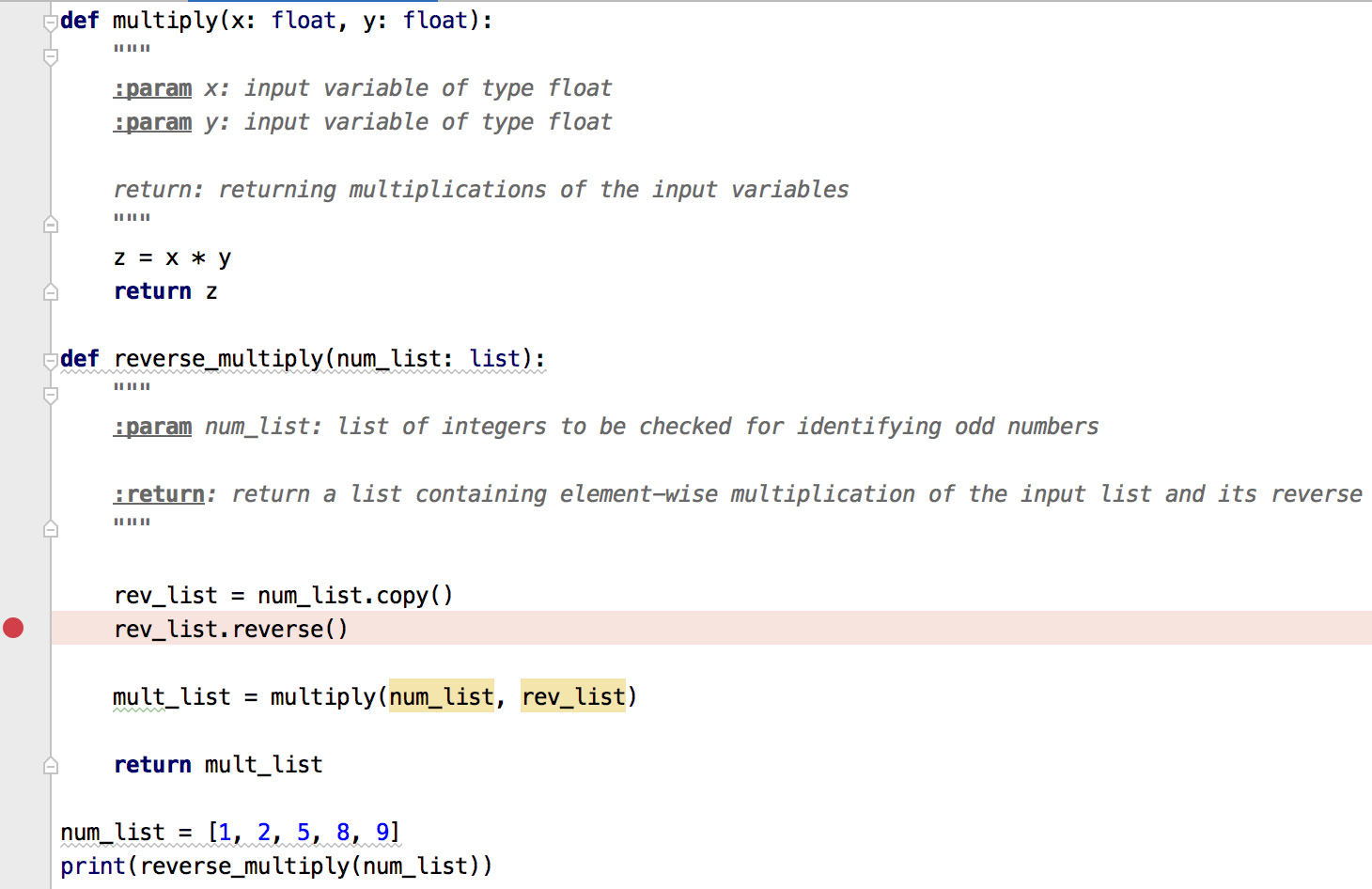
Figure 1.3 – Using breakpoints in PyCharm for code debugging
The breakpoint capabilities in different IDEs are not the same. For example, you can use PyCharm’s conditional breakpoints to speed up your debugging process, which helps you not execute a line of code in a loop or repeat function calls manually. Read more about the debugging features of the IDE you use and consider them as another tool in your toolbox for better and easier Python programming and machine learning modeling.
The debugging techniques and tools we’ve briefly explained here, or those you already know about, could help you develop a piece of code that runs and provides the intended results. You could also follow some best practices for high-quality Python programming and building your machine learning models.
Best practices for high-quality Python programming
Prevention is better than a cure. There are practices you can follow to prevent or decrease the chance of bugs occurring in your code. In this section, we will talk about three of those practices: incremental programming, logging, and defensive programming. Let’s look at each in detail.
Incremental programming
Machine learning modeling in practice, in academia or industry, is beyond writing a few lines of code to train a simple model such as a logistic regression model using datasets that already exist in scikit-learn
. It requires many modules for processing data, training and testing model and postprocessing inferences, or predictions to assess the reliability of the models. Writing code for every small component, then testing it and writing test code using PyTest, for example, could help you avoid issues with each function or class you wrote. It also helps you make sure that the outputs of one module that feed another module as its input are compatible. This process is what is called incremental programming. When you write a piece of software or pipeline, try to write and test it piece by piece.
Logging
Every car has a series of dashboard lights that get turned on when there is a problem with the car. These problems could stop the car from running or cause serious damage if they’re not acted upon, such as low gas or engine oil change lights. Now, imagine there was no light or warning, and all of a sudden, the car you are driving stops or makes a terrible sound, and you don’t know what to do. When you develop functions and classes in Python, you can benefit from logging to log information, errors, and other kinds of messages that help you in identifying potential sources of issues when you get an error message. The following example showcases how to use error and info as two attributes of logging. You can benefit from different attributes of logging in terms of the functions and classes you write to improve data and information gathering while running your code. You can also export the log information in a file using basicConfig()
, which does the basic configuration for the logging system:
import logging def multiply(x: float, y: float): """ :param x: input variable of type float :param y: input variable of type float return: returning multiplications of the input variables """ if not isinstance(x, (int, float)) or not isinstance(y, (int, float)): logging.error('Input variables are not of type float or integer!') z = x * y return z def reverse_multiply(num_list: list): """ :param num_list: list of integers to be checked for identifying odd numbers :return: return a list containing element-wise multiplication of the input list and its reverse """ logging.info("Length of {num_list} is { list_len}".format(num_list=num_list, list_len = len(num_list))) rev_list = num_list.copy() rev_list.reverse() mult_list = [multiply(num_list[iter], rev_list[iter]) for iter in range(0, len(num_list))] return mult_list num_list = [1, 'no', 5, 8, 9] print(reverse_multiply(num_list))
When you run the previous code, you will get the following messages and output:
ERROR:root:Input variables are not of type float or integer! ERROR:root:Input variables are not of type float or integer! [9, 'nononononononono', 25, 'nononononononono', 9]
The logged error messages are the results of attempting to multiply 'no'
, which is a string with another number.
Defensive programming
Defensive programming is about preparing yourself for mistakes that can be made by you, your teammates, and your collaborators. There are tools, techniques, and Python classes to defend the code against such mistakes, such as assertions. For example, using the following line in your code stops it, if the conditions are met, and returns an error message stating AssertionError: Variable should be of
type float
:
assert isinstance(num, float), 'Variable should be of type float'
Version control
The tools and practices we covered here are just examples of how you can improve the quality of your programming and decrease the amount of time needed to eliminate issues and errors in your code. Another important tool in improving your machine learning modeling is versioning. We will talk about data and model versioning in Chapter 10, Versioning and Reproducible Machine Learning Modeling, but let’s briefly talk about code versioning here.
Version control systems allow you to manage changes in your code and files that exist in a code base and help you in tracking those changes, gain access to the history of changes, and collaborate in developing different components of a machine learning pipeline. You can use version control systems such as Git and its associated hosting services such as GitHub, GitLab, and BitBucket for your projects. These tools let you and your teammates and collaborators work on different branches of code without disrupting each other’s work. It also lets you easily go back to the history of changes and find out when a change happened in the code.
If you have not used version control systems, don’t consider them as a new complicated tool or programming language you need to start learning. There are a couple of core concepts and terms you need to learn first, such as commit
, push
, pull
, and merge
, when using Git. Using these functionalities could be even as simple as a few clicks in an IDE such as PyCharm if you don’t want to or know how to use the command-line interface (CLI).
We reviewed some commonly used techniques and tools to help you in debugging your code and high-quality Python programming. However, there are more advanced tools built on top of models such as GPT, such as ChatGPT (https://openai.com/blog/chatgpt) and GitHub Copilot (https://github.com/features/copilot), that you can use to develop your code faster and increase the quality of your code and even your code debugging efforts. We will talk about some of these tools in Chapter 14, Introduction to Recent Advancements in Machine Learning.
Although using the preceding debugging techniques or best practices to avoid issues in your Python code helps you have a low-bug code base, it doesn’t prevent all the problems with machine learning models. This book is about going beyond Python programming for machine learning to help you identify problems with your machine learning models and develop high-quality models.
Debugging beyond Python
Eliminating code issues doesn’t resolve all the issues that may exist in a machine learning model or a pipeline for data preparation and modeling. There could be issues that don’t result in any error message, such as problems that originate from data used for modeling, and differences between test data and production data (that is, data that the model needs to be used for eventually).
Production versus development environments
The development environment is where we develop our models, such as our computers or cloud environments we use for development. It is where we develop our code, debug it, process data, train models, and validate them. But what we do in this stage doesn’t affect users directly.
The production environment is where the model is ready to be used by end users or could affect them. For example, a model could get into production in the Amazon platform for recommending products, be delivered to other teams in a banking system for fraud detection, or even be used in hospitals to help clinicians in diagnosing patients’ conditions better.