Long Short-Term Memory (LSTM)
LSTMs are RNNs whose main objective is to overcome the shortcomings of the vanishing gradient and exploding gradient problem. The architecture is built such that they remember data and information for a long period of time.
LSTMs were designed to overcome the limitation of the vanishing and exploding gradient problems. LSTM networks are a special kind of RNN, which are capable of learning long-term dependencies. They are designed to avoid the long-term dependency problem; being able to remember information for long intervals of time is how they are wired. The following diagram displays a standard recurrent network where the repeating module has a tanh activation function. This is a simple RNN; in this architecture, we often have to face the vanishing gradient problem:
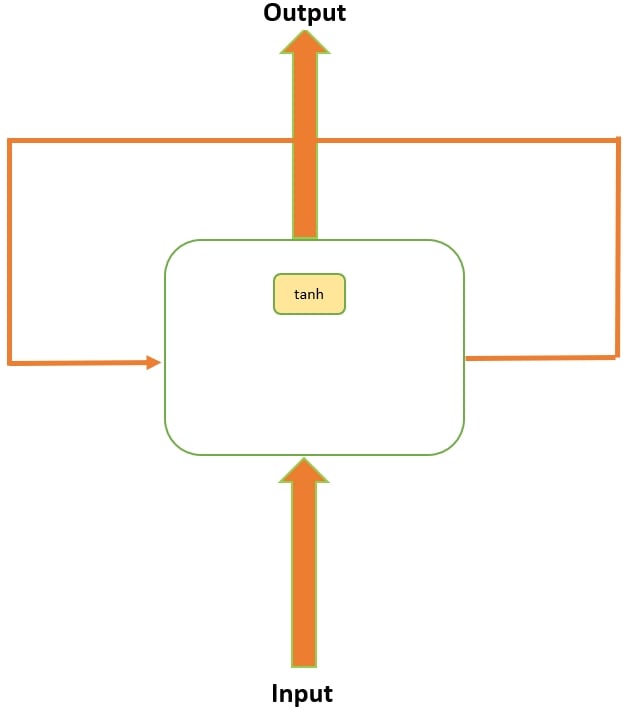
Figure 9.12: A simple RNN model
LSTM architecture is similar to simple RNNs but their repeating module has different components, as shown in the following diagram:
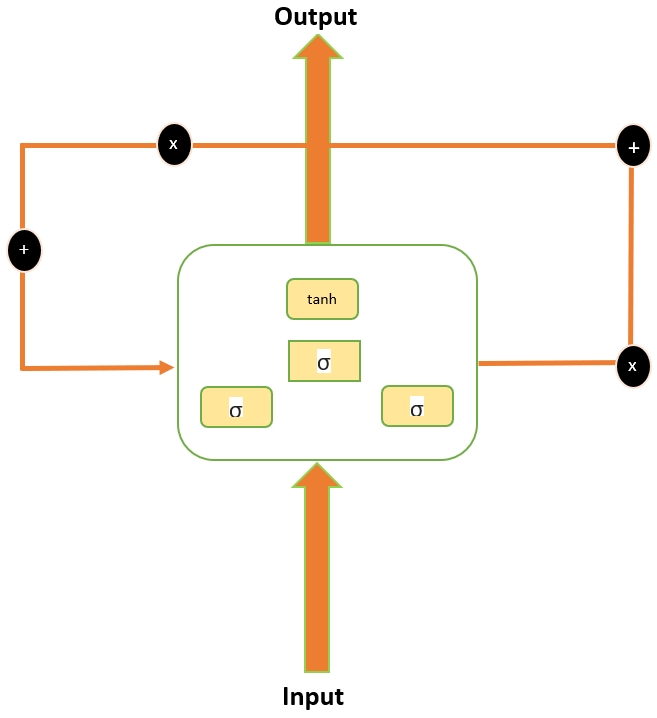
Figure 9.13: The LSTM model architecture...