



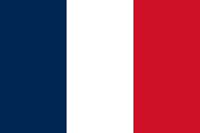


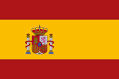



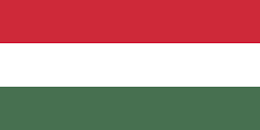

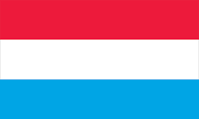





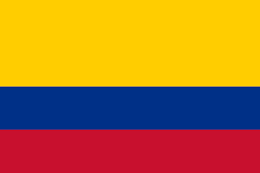
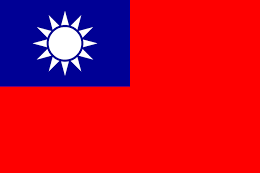
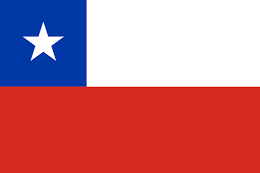



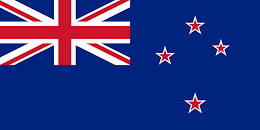
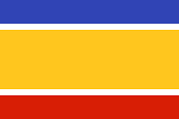
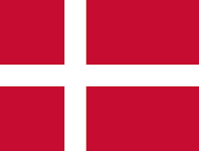
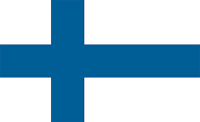







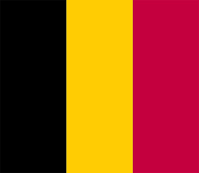

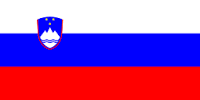





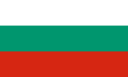


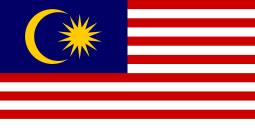



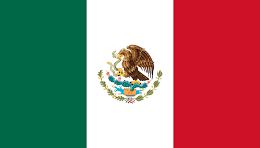

Last month, researchers from the University of Surrey and St Petersburg National Research University of Information Technologies, Mechanics and Optics, published a paper, titled, How to model fake news. The paper states,
“Until now there has been no clear understanding of how to define fake news, much less how to model it. This paper addresses both these issues. A definition of fake news is given, and two approaches for the modelling of fake news and its impact in elections and referendums are introduced.”
According to the researchers, “fake news is information that is inconsistent with factual reality. It is information that originates from the ‘sender’ of fake news, is transmitted through a communication channel and is then received, typically, by the general public. Hence any realistic model for fake news has to be built on the successful and well-established framework of communication theory.”
The false stories on the internet have made it difficult for many to distinguish what is true from what is false. Fake news is a threat to the democratic process in the US, the UK, and elsewhere. For instance, the existence of Holocaust denialists illustrates how doubts about a major historical event can gain traction with certain individuals.
Fake news has become a serious concern to society so much so that it can even endanger the democratic process. The gravity of this issue has become widely acknowledged especially after the 2016 US presidential election and the ‘Brexit’ referendum in the UK on the membership of the European Union.
The researchers have presented two approaches for the modeling of fake news in elections, The first is based on the idea of a representative voter, useful to obtain a qualitative understanding of the effects of fake news. The other is based on the idea of an election microstructure, useful for practical implementation in concrete scenarios.
The researchers categorized voters into two categories, namely Category I voters and Category II voters. Category I voters are the ones who are unaware of the existence of the fake news. Category II voters are the ones that possess the knowledge that there may be fake news in circulation, but they do not know how many pieces of fake news have been released, or at what time.
According to the first approach, those who are influenced by fake news are not viewed as being irrational. But they lack the ability to detect and mitigate the changes caused by the fake news. The transition from the behavioral model of an individual to that of the electorate brings the idea of a ‘representative voter’ whose perception represents the aggregation of the diverse views held by the public at large. The category I voters are an example of the representative voter as they can’t detect or mitigate the fake news.
The researchers examine the problem of estimating the release times of fake news that generates a new type of challenge in communication theory. This estimate is required for characterizing a voter. The voter is aware of the potential presence of fake news but is not sure which items of information are fake.
The researchers show an illustration of the dynamics of opinion-poll statistics in a referendum in the presence of a single piece of fake news. An application to an election where multiple pieces of news (fake) are released at random times is considered. For instance, the qualitative behavior of the dynamics of the opinion-poll statistics during the 2016 US presidential election can be replicated by the model suggested by the researchers.
Further analysis done by the researchers introduce an ‘election microstructure’ model in which an information-based scheme is used to describe the dynamical behavior of individual voters and the resulting collective voting behavior of the electorate under the influence of fake news. The category II voters are an example of election microstructure as these voters have information about the fake news but aren’t aware of the entire pieces of news and the precise time.
The modeling framework proposed in this paper uses Wiener’s philosophy. The authors have applied and extended techniques of filtering theory, which is a branch of communication theory that aims at filtering out noise in communication channels, in a novel way to generate models that are well-suited for the treatment of fake news.
The mathematical aspect used in election microstructure model is the same as the one in representative voter framework. The only difference is that in election microstructure model the signal in the information process can be transmitted by a sender (e.g., the candidate).
According to the researchers, techniques like Deep learning and other related techniques can help in the detection and prevention of fake news. However, to address the issues surrounding the impact, it is important that a consistent mathematical model is developed that describes the phenomena resulting from the flow of fake news. Such a model should be intuitive and tractable, so that model parameter can be calibrated against real data, and so that predictions can be made, either analytically or numerically.
In both the approaches, suggested by the researchers, the results illustrate the impact of fake news in elections and referendums. The researchers have further demonstrated that by merely estimating the presence of fake news, an individual is able to largely mitigate the effects. The researchers have described the two categories of voters and they further conclude that the Category II voters know the parameters of the fake news terms.
The researchers plan to include such optimal release strategies in their models. The election microstructure approach might also get developed further by allowing dependencies between the various factors. Also, the researchers plan to introduce several different information processes reflecting the news consumption preferences of different sections of society. These additions might be challenging but an interesting direction for research might come up.
To know more about the modeling techniques for fake news, check out the paper How to model fake news.
BabyAI: A research platform for grounded language learning with human in the loop, by Yoshua Bengio et al
Facebook is reportedly rating users on how trustworthy they are at flagging fake news
Four 2018 Facebook patents to battle fake news and improve news feed