



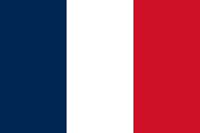


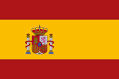



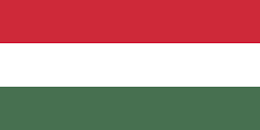

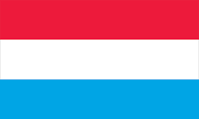





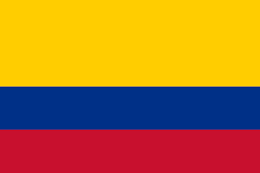
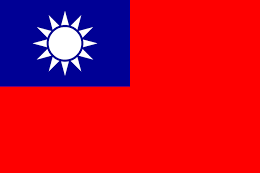
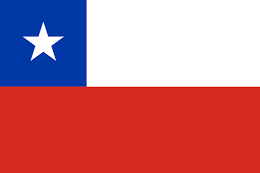



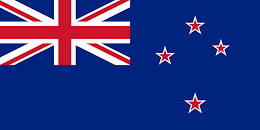
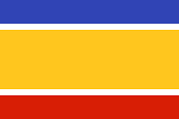
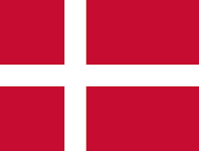
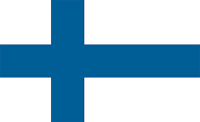







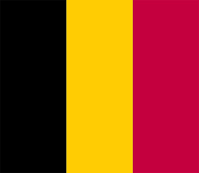

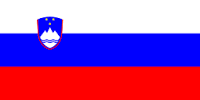





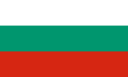


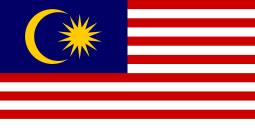



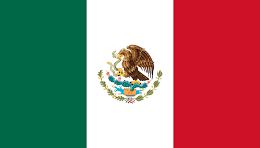

We already know that mobile is a big market with growing opportunities. We are also hearing about the significant revenue that the IoT will generate. Machina Research predicts that the revenue opportunity will increase to USD$4 trillion by 2025. In the mainstream, both of these technologies are heavily reliant on the cloud, and as they become more pervasive, issues such as response delay and privacy are starting to surface. That’s where Edge Computing and Edge Analytics can help.
As the demand for more complex applications on mobile increases, we needed to offload some of the computational demands from the mobile device. An example is speech recognition and processing applications such as Siri, which needs to access cloud-based servers in order to process user’s requests. Cloud enabled a wide range of services to be delivered on mobile due to almost unlimited processing and storage capability. However, the trade-off was the delay arising from the fact that the cloud infrastructure was often a large distance from the device.
The solution is to move some of the data processing and storage to either a location closer to the device (a “cloudlet” or “edge node”) or to the device itself. “Fog Computing” is where some of the processing and storage of data occurs between the end devices and cloud computing facilities. “Mist Computing” is where the processing and storage of data occurs in the end devices themselves. These are collectively known as “Edge Computing” or “Edge Analytics” and, more specifically for mobile, “Mobile Edge Computing” (MEC).
As a developer of either mobile or IoT technology, Edge Analytics provides significant benefits.
In essence, the proximity of the cloudlets or edge nodes to the end devices reduces latency in response. Often high bandwidth is possible and jitter is reduced. This is particularly important where a service is sensitive to response delays or to services with high processing demands such as VR or AR.
By processing the raw data either in the end device or in the cloudlet, the demands that are placed on the central cloud facility is reduced because smaller volumes of data need to be sent to the cloud. This allows a greater number of connections to that facility.
Maintaining privacy is a significant concern for IoT. Processing data in either end devices or at cloudlets gives the owner of that data the ability to control the data that is release before it is sent to the cloud. Further, the data can be made anonymous or aggregated before transmission.
Developers of mobile or IoT technology are able to use more contextual information and a wider range of SDKs specific to the device.
In March this year, Amazon AWS had a server outage, causing significant disruption for many services that relied upon their S3 storage service. Edge computing and analytics could effectively allow your service to remain operational through a fallback to a cloudlet.
IoT technology is being used to monitor and then manage traffic conditions in a specific location. Identifying traffic congestion, determining the source of that congestion and determining alternative routes requires fast data processing. Using cloud computing results in response delays associated with the transmission of significant volumes of data both to and from the cloud. Edge Analytics means the data is processed closer to that location, and then sent to drivers in much shorter time.
Another example is supporting the distribution of localized content such as the addition of advertisements to a video stream that is only being distributed within a small area without having to divert the stream to another server for processing.
As with all emerging technology, there are a number of open or unresolved issues for Edge Analytics. A major and non-technical issue is, what is the business model that will support the provision of cloudlets or edge nodes, and what will be the consequent cost of providing these services? Security also remains a concern: how will the perimeter security of cloudlets compare to that implemented in cloud facilities? As IoT continues to grow, so will the variety of needs for the processing and management of that data. How will cloudlets cope with this demand?
Increased responsiveness, flexibility, and greater control over data to reduce privacy breach risk are strong (and not the only) reasons for adopting Edge Analytics in the development of your mobile or IoT service. It presents a real opportunity in the differentiation of service offerings in a market that is only going to get more competitive in the near future. Consider both the different options that are available to you, and the benefits and pitfalls of those options.
Peter Worthy is an Interaction Designer currently completing a PhD exploring human values and the design of IoT technology in a domestic environment. Professionally Peter’s interests range from design research methods, understanding HCI and UX in emerging technologies, and physical computing. Pete also works at a University tutoring across a range of subjects and supporting a project that seeks to develop context aware assistive technology for people living with dementia.