ML use cases in media and entertainment
The media and entertainment (M&E) industry encompasses the production and distribution of various forms of content, such as films, television, streaming content, music, games, and publishing. The industry has undergone significant changes due to the growing prevalence of streaming and over-the-top (OTT) content delivery over traditional broadcasting. M&E customers, having access to an ever-increasing selection of media content, are shifting their consumption habits and demanding more personalized and enhanced experiences across different devices, anytime, anywhere. The industry is also characterized by intense competition, and to remain competitive, M&E companies need to identify new monetization channels, improve user experience, and improve operational efficiency. The workflow for media production and distribution is illustrated in the following diagram:
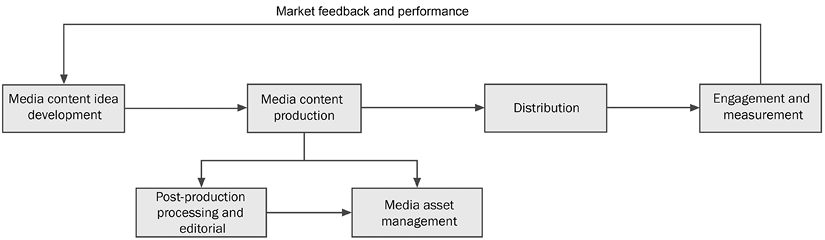
Figure 2.10: Media production and distribution workflow
In recent years, I have seen M&E companies increasingly adopting ML in the different stages of the media lifecycle, such as content generation and content distribution, to improve efficiency and spur business growth. For instance, ML has been used to enhance content management and search, develop new content development, optimize monetization, and enforce compliance and quality control.
Content development and production
During the initial planning phase of the film production lifecycle, content producers need to make decisions on the next content based on factors such as estimated performance, revenue, and profitability. To aid this process, filmmakers have adopted ML-based predictive analytics models to help predict the popularity and profitability of new ideas by analyzing factors such as casts, scripts, the past performance of different films, and target audience. This allows producers to quickly eliminate ideas with limited market potential and focus their efforts on developing more promising and profitable ideas.
To support personalized content viewing needs, content producers often segment long video content into smaller micro-segments around certain events, scenes, or actors, so that they can be distributed individually or repackaged into something more personalized to individual preferences. This ML-based approach can be used to create video clips by detecting elements such as scenes, actors, and events for different target audiences with varying tastes and preferences.
Content management and discovery
M&E companies with vast digital content assets need to curate their content to create new content for new monetization opportunities. To support this, these companies need rich metadata for the digital assets to enable different content to be searched and discovered. Consumers also need to search for content easily and accurately for different usages, such as for personal entertainment or research. Without metadata tagging, discovering relevant content is quite challenging. As part of the digital asset management workflow, many companies hire humans to review and tag this content with meaningful metadata for discovery. However, manual tagging is very costly and time-consuming, leading to insufficient metadata for effective content management and discovery.
Computer vision models can automatically tag image and video content for items such as objects, genres, people, places, or themes. ML models can also interpret the meaning of textual content such as topics, sentiment, entities, and sometimes video. Audio content can be transcribed into text using ML techniques for additional text analysis. ML-based text summarization can help you summarize long text as part of the content metadata generation. The following diagram illustrates where ML-based analysis solutions can be incorporated into the media asset management flow:

Figure 2.11: ML-based media analysis workflow
ML-based solutions are being increasingly adopted by M&E companies to streamline media asset management workflows. Overall, these solutions can lead to significant time and cost savings for M&E companies while improving the user experience for consumers.
Content distribution and customer engagement
Nowadays, media content such as films and music is increasingly being distributed through digital video on demand (VOD) and live streaming on different devices, bypassing traditional media such as DVDs and broadcasting, providing consumers with a variety of media content choices. As a result, media companies are facing challenges in customer acquisition and retention. To keep users engaged and on their platforms, M&E companies are focusing on highly personalized product features and content. One effective way to achieve highly personalized engagement is through a content recommendation engine, which uses viewing and engagement behavior data to train ML models that target individuals based on their preferences and viewing patterns.
This allows for a diverse range of media content to be recommended to users, including videos, music, and games.
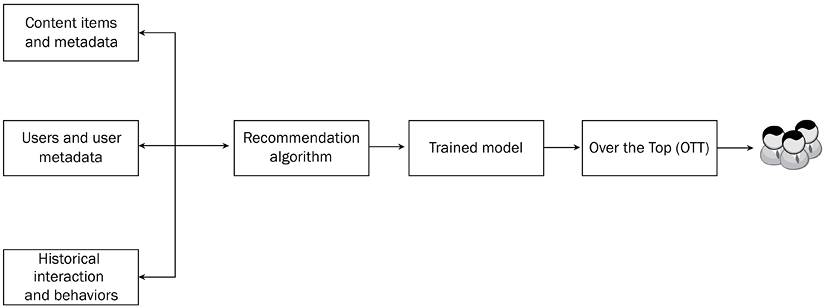
Figure 2.12: Recommendation ML model training
Recommendation technologies have been around for many years and have improved greatly over the years. Nowadays, recommendation engines can learn patterns using multiple data inputs, including historical interactions, sequential patterns, and the metadata associated with the users and content. Modern recommendation engines can also learn from the user’s real-time behaviors/decisions and make dynamic recommendations based on them.