Tidying when multiple variables are stored as column names
One particular flavor of messy data appears whenever the column names contain multiple different variables themselves. A common example of this scenario occurs when age and sex are concatenated together. To tidy datasets like this, we must manipulate the columns with the pandas str
accessor, an attribute that contains additional methods for string processing.
Getting ready...
In this recipe, we will first identify all the variables of which some will be concatenated together as column names. We then reshape the data and parse the text to extract the correct variable values.
How to do it...
- Read in the men's
weightlifting
dataset, and identify the variables:
>>> weightlifting = pd.read_csv('data/weightlifting_men.csv') >>> weightlifting
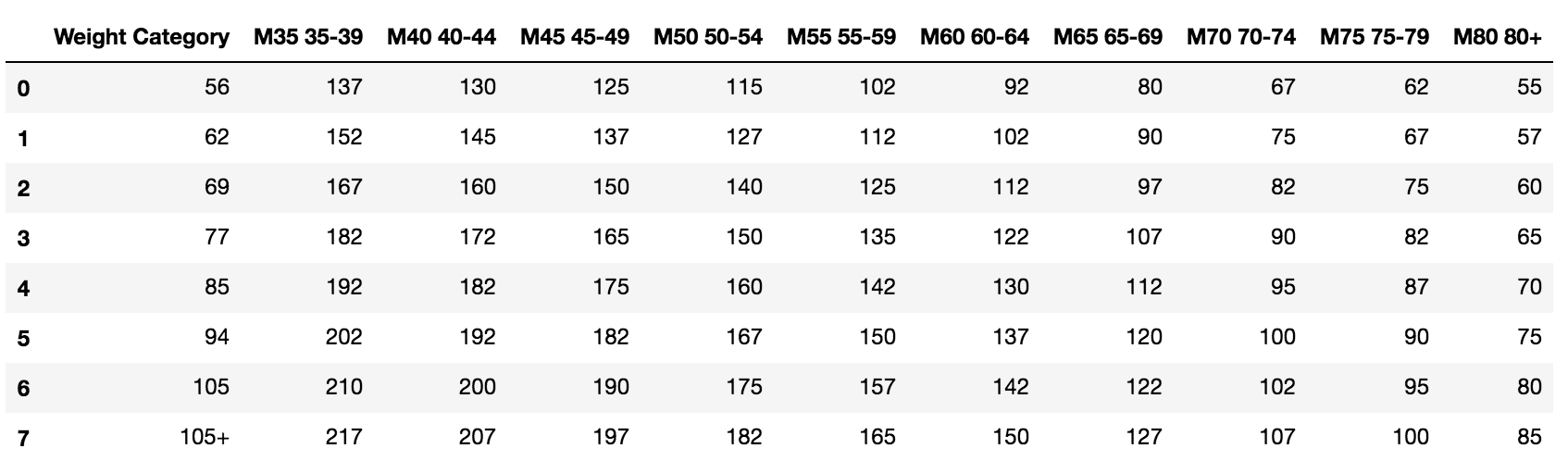
- The variables are the weight category, sex/age category, and the qualifying total. The age and sex variables have been concatenated together into a single cell. Before we can separate them...