We shall take a step-by-step approach to understand the forward and reverse pass with a single hidden layer. The input layer has one neuron and the output will solve a binary classification problem (predict 0 or 1). In the following figure is shown a forward and reverse pass with a single hidden layer:
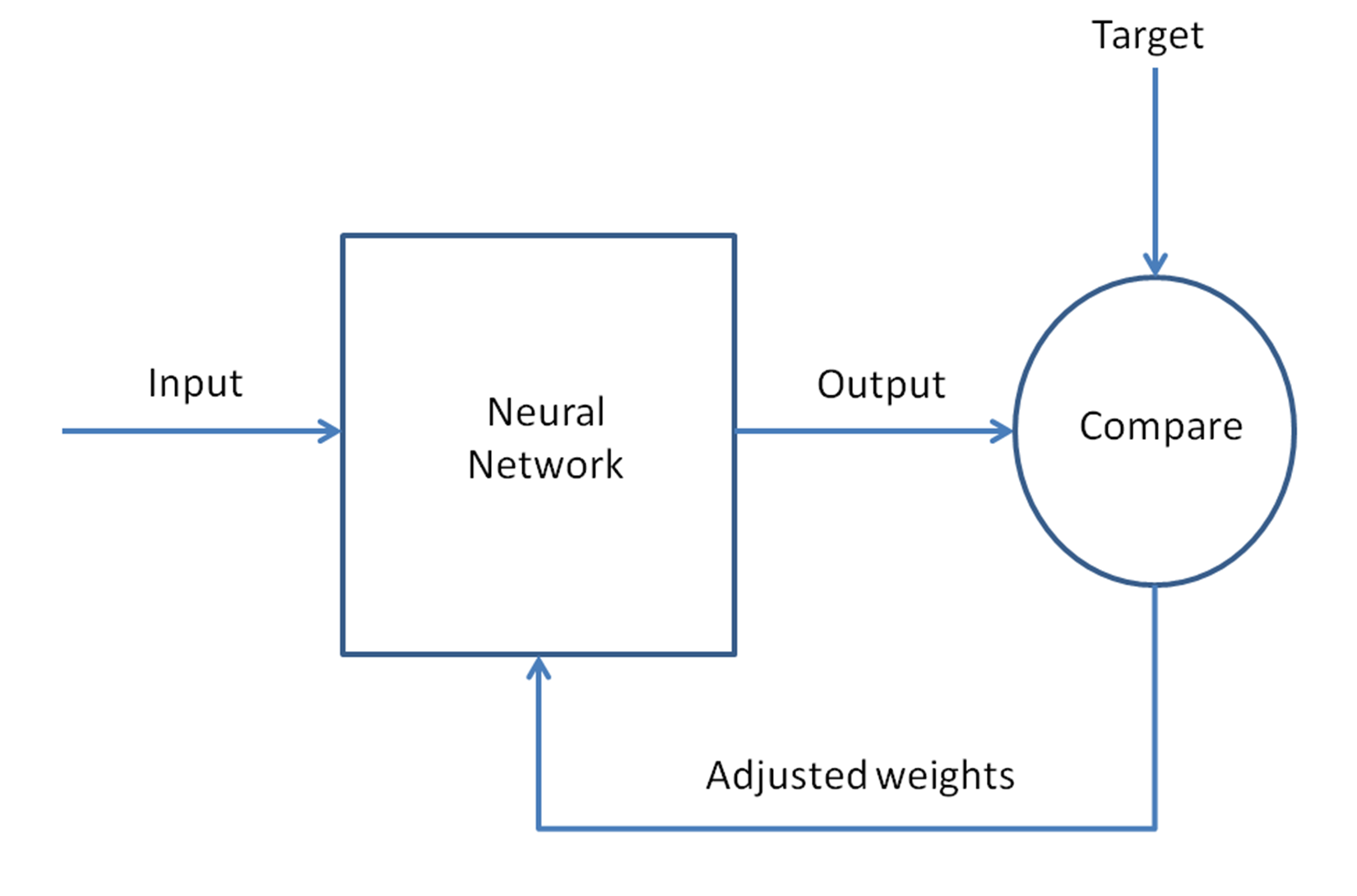
Next, let us analyze in detail, step by step, all the operations to be done for network training:
- Take the input as a matrix.
- Initialize the weights and biases with random values. This is one time and we will keep updating these with the error propagation process.
- Repeat the steps 4 to 9 for each training pattern (presented in random order), until the error is minimized.
- Apply the inputs to the network.
- Calculate the output for every neuron from the input layer, through the hidden layer(s), to the output layer.
- Calculate the error at the outputs: actual minus predicted.
- Use the output error to compute error signals for previous layers. The partial derivative of the activation function is used to compute the error signals.
- Use the error signals to compute weight adjustments.
- Apply the weight adjustments.
Steps 4 and 5 are forward propagation and steps 6 through 9 are backpropagation.
The learning rate is the amount that weights are updated is controlled by a configuration parameter.
The complete pass back and forth is called a training cycle or epoch. The updated weights and biases are used in the next cycle. We keep recursively training until the error is very minimal.
We shall cover more about the forward and backpropagation in detail throughout this book.