There are many activation functions available for a neural network to use. We shall see a few of them here.
Different activation functions
Linear function
The simplest activation function, one that is commonly used for the output layer activation function in neural network problems, is the linear activation function represented by the following formula:
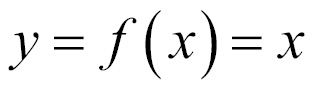
The output is same as the input and the function is defined in the range (-infinity, +infinity). In the following figure, a linear activation function is shown:
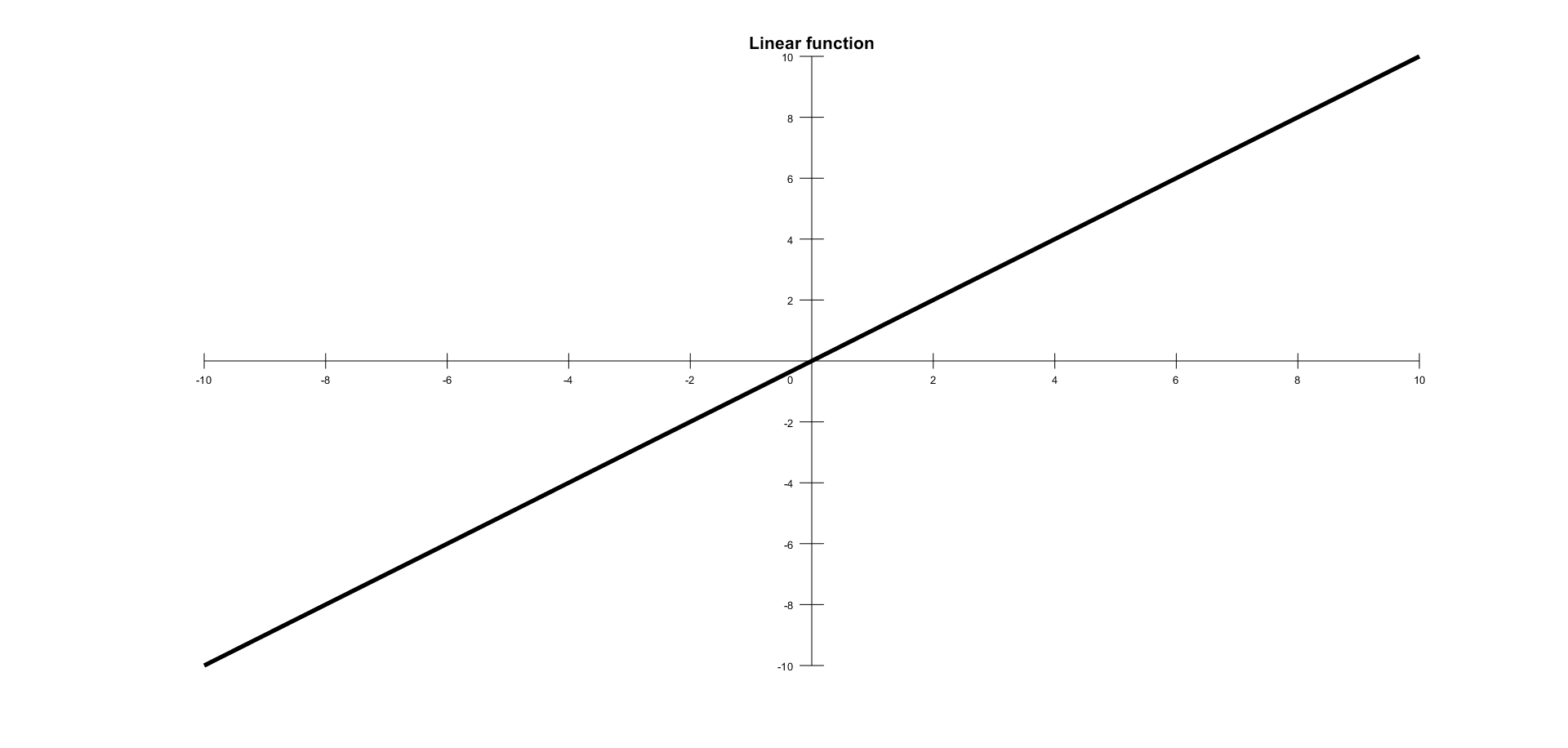
Unit step activation function
A unit step activation function is a much-used feature in neural networks. The output assumes value 0 for negative argument and 1 for positive argument. The function is as follows:
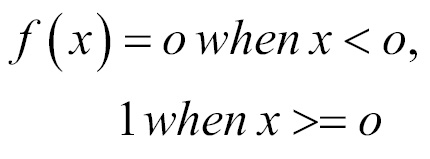
The range is between (0,1) and the output is binary in nature. These types of activation functions are useful for binary schemes. When we want to classify an input model in one of two groups, we can use a binary compiler with a unit step activation function. A unit step activation function is shown in the following figure:
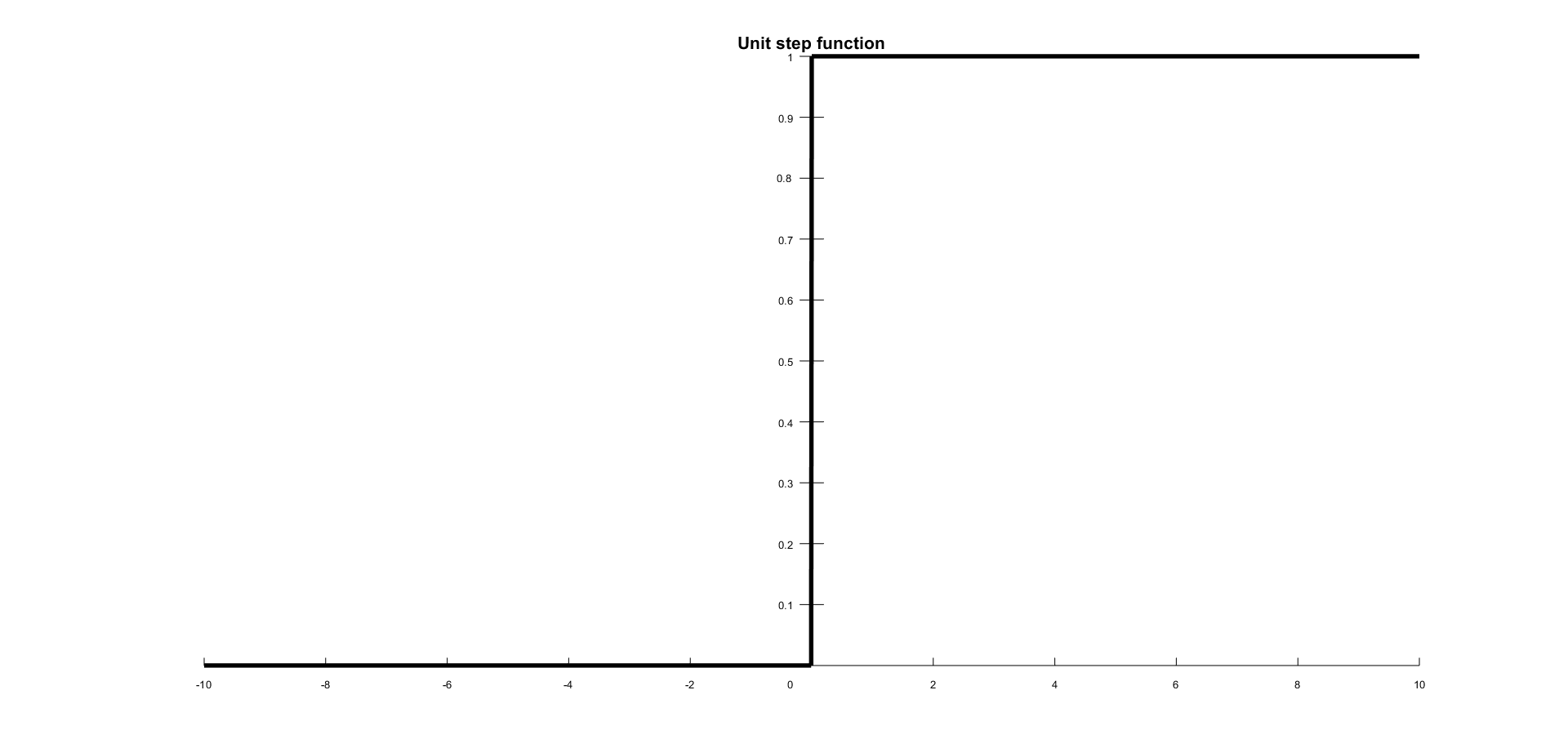
Sigmoid
The sigmoid function is a mathematical function that produces a sigmoidal curve; a characteristic curve for its S shape. This is the earliest and often used activation function. This squashes the input to any value between 0 and 1, and makes the model logistic in nature. This function refers to a special case of logistic function defined by the following formula:
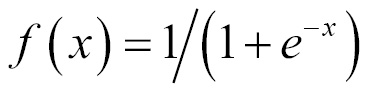
In the following figure is shown a sigmoid curve with an S shape:
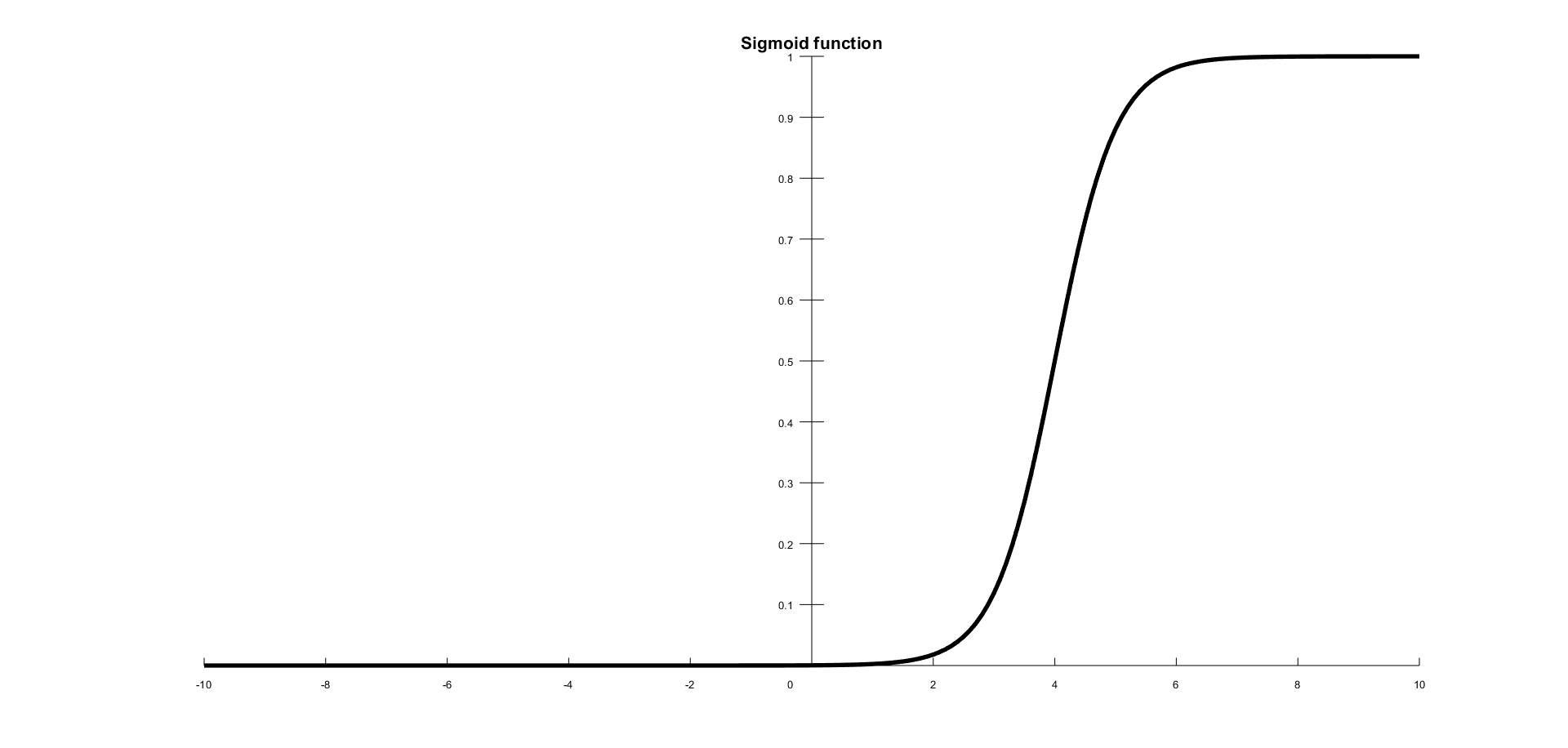
Hyperbolic tangent
Another very popular and widely used activation feature is the tanh function. If you look at the figure that follows, you can notice that it looks very similar to sigmoid; in fact, it is a scaled sigmoid function. This is a nonlinear function, defined in the range of values (-1, 1), so you need not worry about activations blowing up. One thing to clarify is that the gradient is stronger for tanh than sigmoid (the derivatives are more steep). Deciding between sigmoid and tanh will depend on your gradient strength requirement. Like the sigmoid, tanh also has the missing slope problem. The function is defined by the following formula:
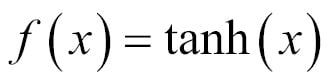
In the following figure is shown a hyberbolic tangent activation function:
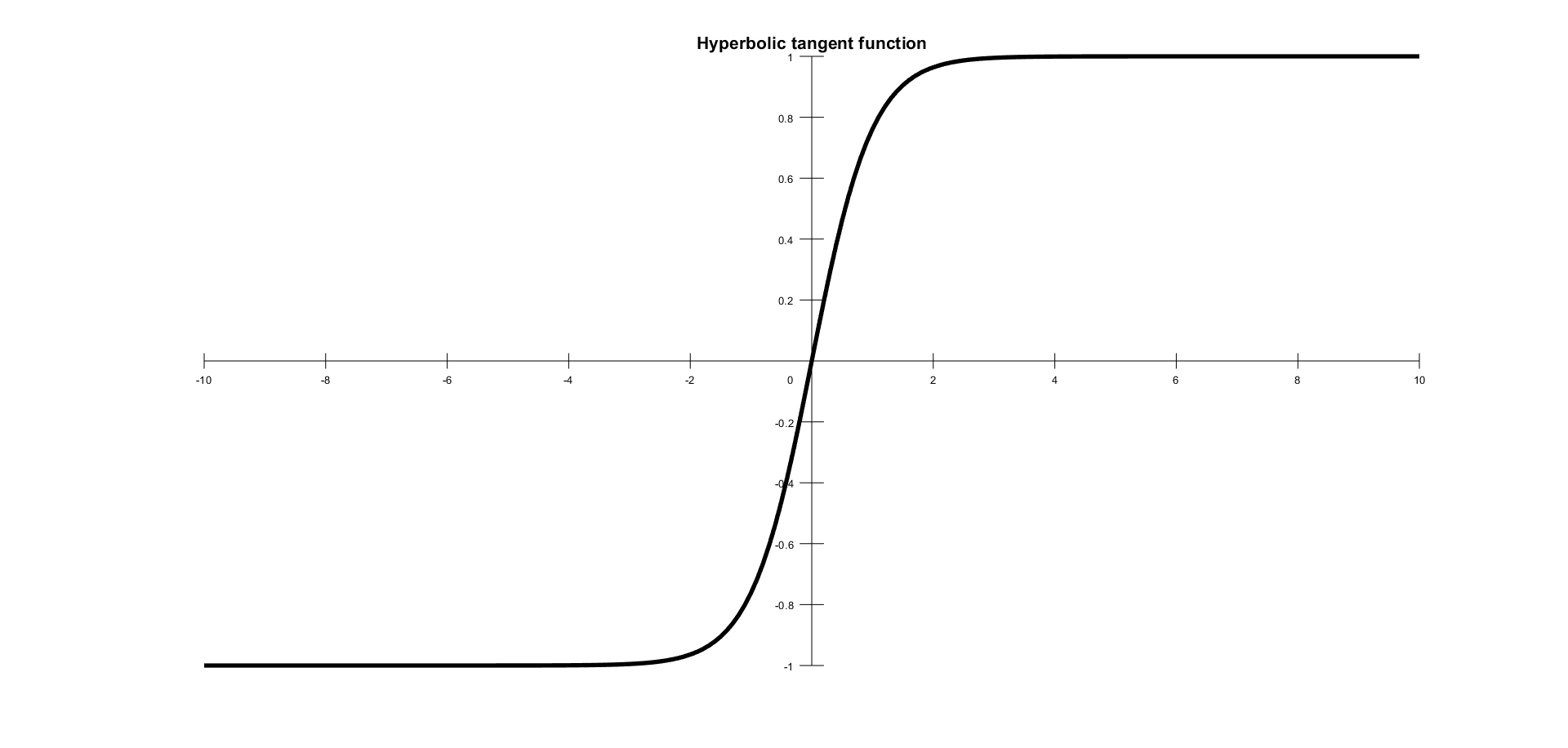
This looks very similar to sigmoid; in fact, it is a scaled sigmoid function.
Rectified Linear Unit
Rectified Linear Unit (ReLU) is the most used activation function since 2015. It is a simple condition and has advantages over the other functions. The function is defined by the following formula:
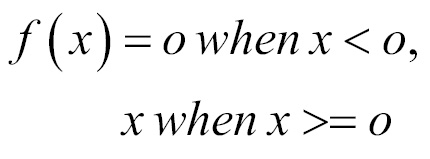
In the following figure is shown a ReLU activation function:
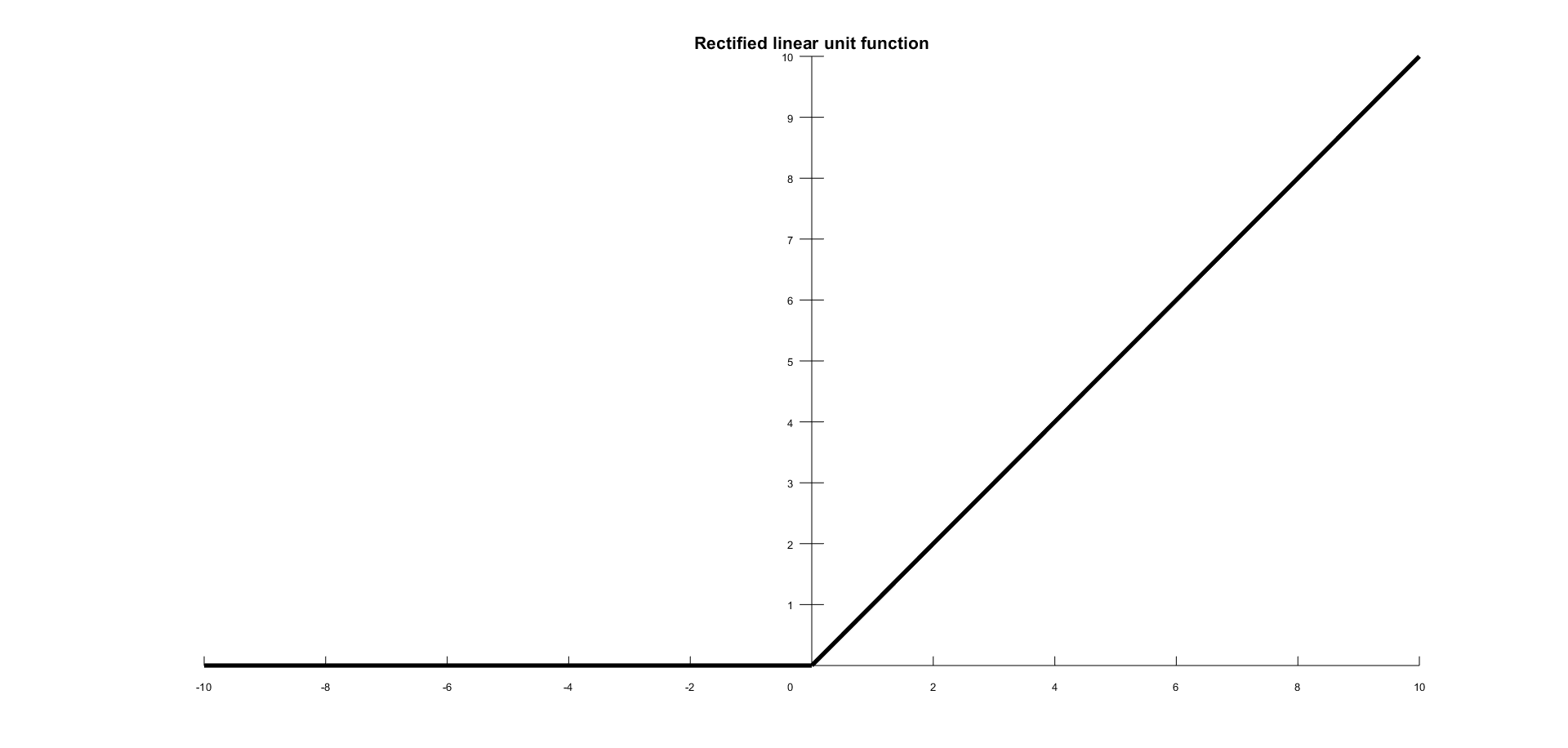
The range of output is between 0 and infinity. ReLU finds applications in computer vision and speech recognition using deep neural nets. There are various other activation functions as well, but we have covered the most important ones here.