Dimensionality reduction
Word embedding is now a basic building block for natural language processing. GloVe, or word2vec, or any other form of word embedding will generate a two-dimensional matrix, but it is stored in one-dimensional vectors. Dimensonality here refers to the size of these vectors, which is not the same as the size of the vocabulary. The following diagram is taken from https://nlp.stanford.edu/projects/glove/ and shows vocabulary versus vector dimensions:
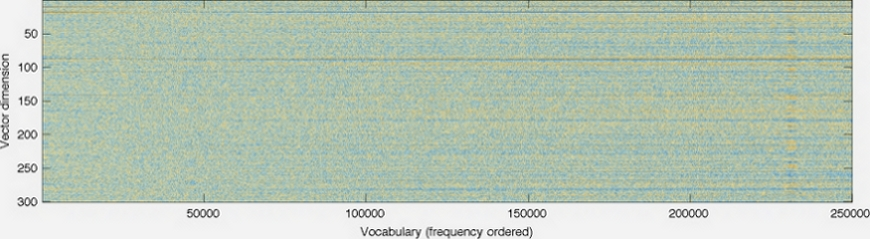
The other issue with large dimensions is the memory required to use word embeddings in the real world; simple 300 dimensional vectors with more than a million tokens will take 6 GB or more of memory to process. Using such a lot of memory is not practical in real-world NLP use cases. The best way is to reduce the number of dimensions to decrease the size. t-Distributed Stochastic Neighbor Embedding (t-SNE) and principal component analysis (PCA) are two common approaches used to achieve dimensionality reduction. In the next...