ML at scale
This book is about implementing ML at scale and how to use H2O.ai technology to succeed in doing so. What specifically do we mean by ML at scale? We can see three contexts and challenges of scale during the ML life cycle – building models from large datasets, deploying these models in enterprise production environments, and executing the full range of ML activities within the complexities of enterprise processes and stakeholders. This is summarized in the following figure:
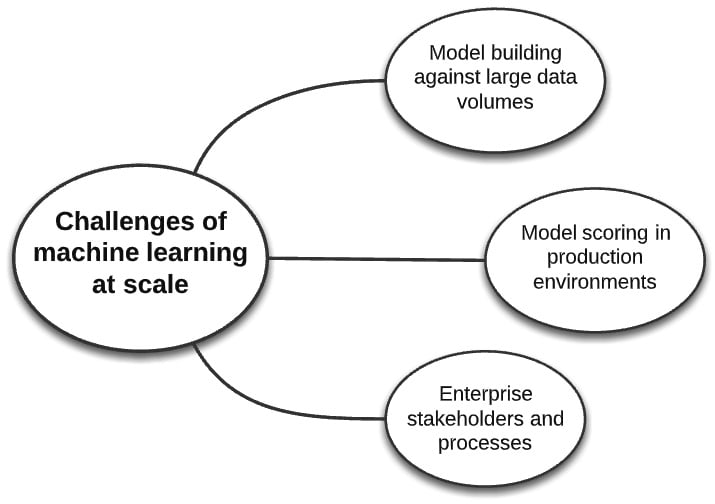
Figure 1.1 – The challenges of ML at scale
Let's drill down further on these challenges. Before doing so, we will oversee a generic conception of the ML life cycle, which will be useful as a reference throughout the book.