We discussed optimal goal-seeking strategies in the context of the MABP, but let's now discuss them more generally:
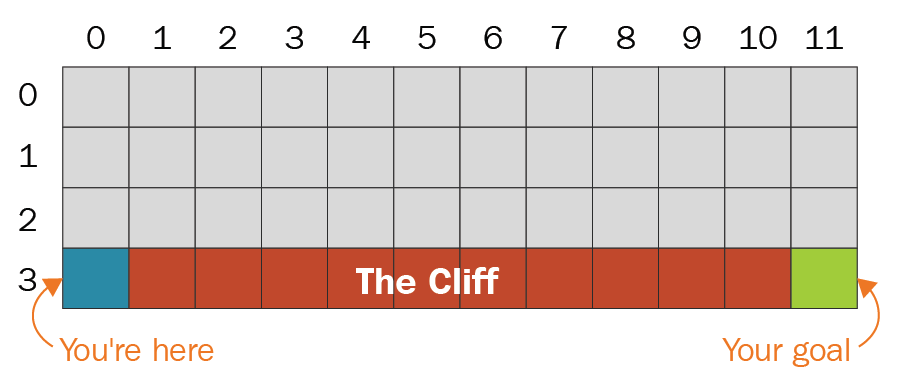
As we briefly discussed in Chapter 1, Brushing Up on Reinforcement Learning Concepts, regarding the differences between Q-learning and State-Action-Reward-State-Action (SARSA), we can sum those differences up as follows: Q-learning takes the optimal path to the goal, while SARSA takes a suboptimal but safer path, with less risk of taking highly suboptimal actions.
In the well-known cliff-walking problem, the goal is to start at the bottom left square in the preceding diagram and get to the bottom right square while getting the highest score possible. If you step on a blue square, then you get a penalty of -100 points, and if you step on a gray square, then you get a penalty of -1 points.
Here, the optimal...