So far, we have been using accuracy as the default metric for evaluating classification models. We used it because it is the most intuitive metric—it is just the proportion of cases correctly predicted by the classifier. So, an accuracy of 0.75 (or 75%) means that, on average, we should expect the classifier to make an accurate prediction 75% of the time. Although sometimes useful, this metric is very limited. Evaluating a classifier, even a binary classifier such as the one we are working with in the credit card default problem, is tricky.
In this section, we examine how we can make a more detailed evaluation of a binary classification model. We will use the credit card default dataset again, so we need to load and prepare everything again for this chapter. Let's run the code we have in one of the notebooks for this chapter. As...




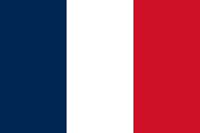


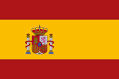




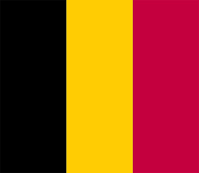
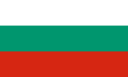
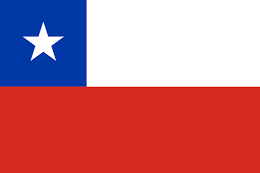
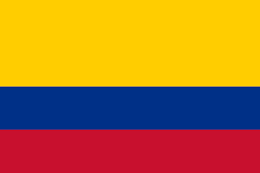
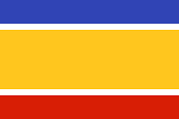

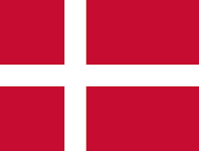



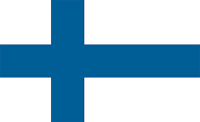

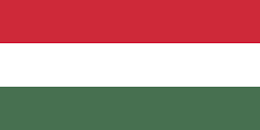






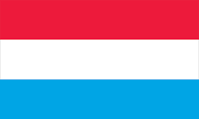
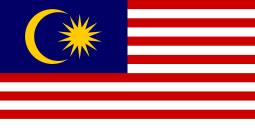

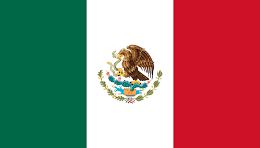

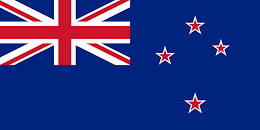







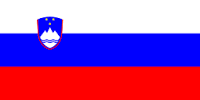




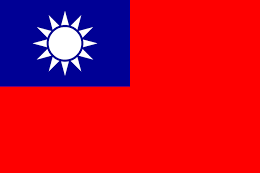


