In this chapter, we started by giving the definition of an artificial neural network, and showed you how individual ANs can be combined into dense layers, which combine together into a full-on deep neural network. We then implemented a dense layer in CUDA-C and made an appropriate corresponding Python wrapper class. We also included functionality to add ReLU and sigmoid layers on the outputs of a dense layer. We saw the definition and motivation of using a softmax layer, which is used for classification problems, and then implemented this in CUDA-C and Python. Finally, we implemented a Python class so that we could build a sequential feed-forward DNN from the prior classes; we implemented a cross-entropy loss function, and then used this in our loss function in our implementation of gradient descent to train the weights and biases in our DNN. Finally, we used our implementation...




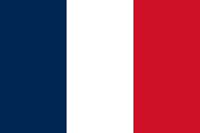


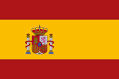



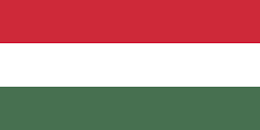

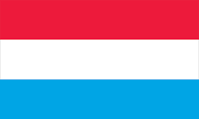





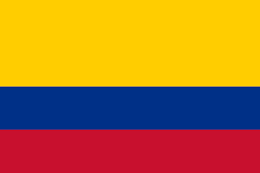
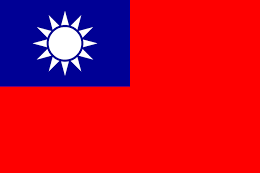
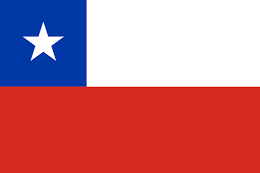



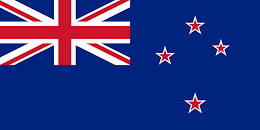
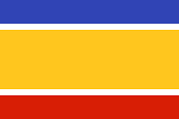
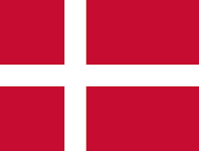
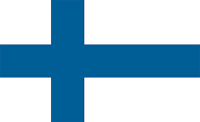







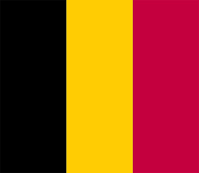

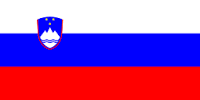





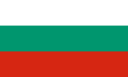


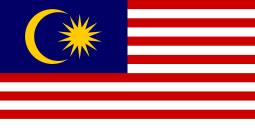



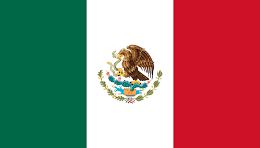
