Essentially, there are two ways for us to retrieve and update knowledge about our real world. One is to store the knowledge in vector space and read the file to our memory during runtime using programs such as Word2Vector and BERT. Another approach is to load the knowledge into a graph database, such as Neo4j, and retrieve and query the data. The strength and weakness of both approaches lies in speed and transparency. For high-speed subject classification, in-memory models fare better, but for tasks that require transparency, such as banking decisions, the updating of data requires full transparency and permanent record keeping. In these cases, we will use a graph database. However, like the example we briefly covered in Chapter 7, Sensing Market Sentiment for Algorithmic Marketing at Sell Side, NLP is required to extract information from the document before we can store the information in graph format.




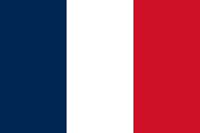


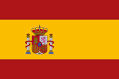



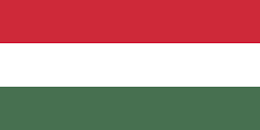

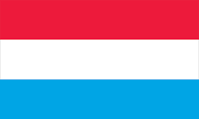





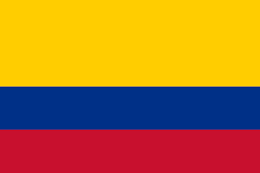
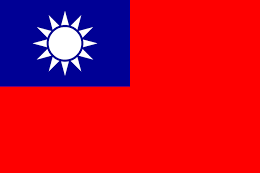
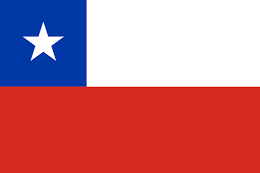



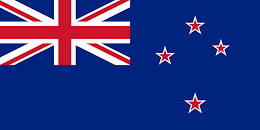
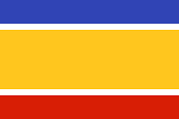
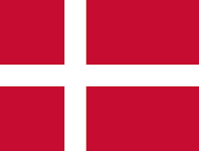
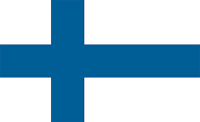







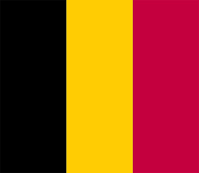

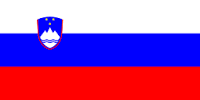





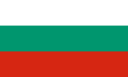


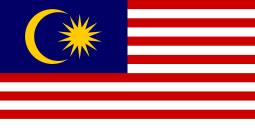



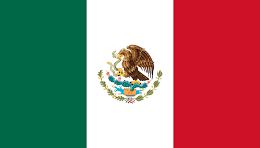
