If you look back at Figure 10, the vector-to-sequence model would correspond to the decoder funnel shape. The major philosophy is that most models usually can go from large inputs down to rich representations with no problems. However, it is only recently that the machine learning community regained traction in producing sequences from vectors very successfully (Goodfellow, I., et al. (2016)).
You can think of Figure 10 again and the model represented there, which will produce a sequence back from an original sequence. In this section, we will focus on that second part, the decoder, and use it as a vector-to-sequence model. However, before we go there, we will introduce another version of an RNN, a bi-directional LSTM.
Bi-directional LSTM
A Bi-directional LSTM (BiLSTM), simply put, is an LSTM that analyzes a sequence going forward and backward, as shown in Figure 14:
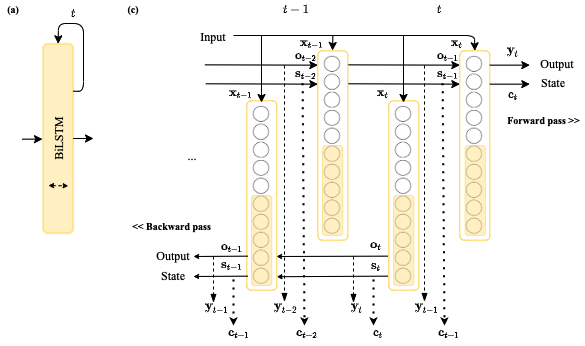
Consider the following examples of sequences...