



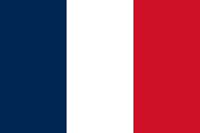


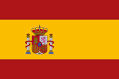



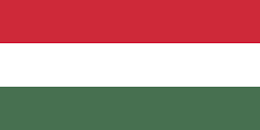

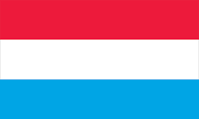





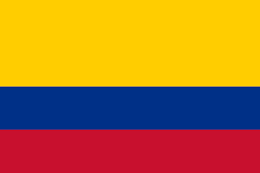
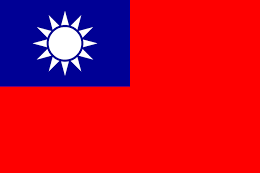
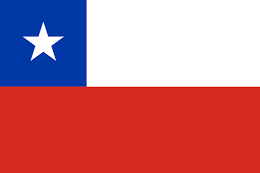



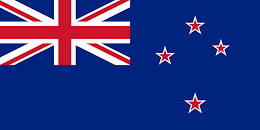
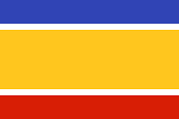
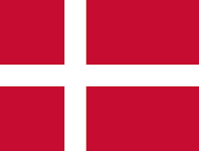
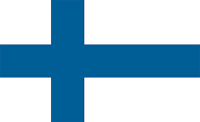







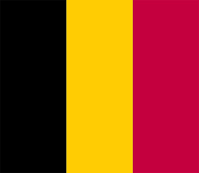

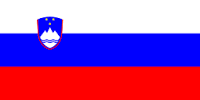





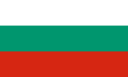


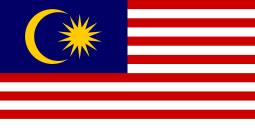



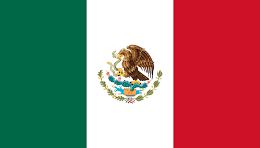

Climate change effects are now visible in all countries around the globe. The world is witnessing phenomena like higher temperature, flooding, ice-melting, and much more. There have been many technologies invented in the last decade to help humans understand and adapt to these climatic changes.
Earlier this month, researchers from Montreal Institute for Learning Algorithms, ConscientAI Labs and Microsoft Research came up with a project that aims to generate images which will depict accurate, vivid, and personalized outcomes of climate change using Machine Learning (ML) and Cycle-Consistent Adversarial Networks (CycleGANs). This will enable individuals to make more informed choices about their climate future by creating an understanding of the effects of climate change, while maintaining scientific credibility using climate model projections.
The project is to develop a Machine Learning (ML) based tool. This tool will show in a personalized way, the probable effect that climate change will have on a specific location familiar to the viewer. When given an address, the tool will generate an image projecting transformations which are likely to occur there, based on a formal climate model.
For the initial version, the generated images consist of houses and buildings specifically after flooding events. The challenge in generating realistic images using Cycle-Consistent Adversarial Networks (CycleGANs) is to collect the training data needed in order to extract the mapping function. The researchers manually searched open source photo-sharing websites for images of houses from various neighborhoods and settings, such as suburban detached houses, urban townhouses, and apartment buildings. They have gathered over 500 images of non-flooded houses and the same number of flooded locations, and re-sized them to 300x300 pixels.
The networks were trained using the publicly available PyTorch. The CycleGAN model for 200 epochs were trained using the trained images, using the Adam solver with a batch size of 1 and training the model from scratch with a learning rate of 0.0002. As per the CycleGAN training procedure, the learning rate is constant for the first 100 epochs and linearly decayed to zero over the next 100 epochs.
The trained CycleGAN model was successful in learning an adequate mapping between grass and water, which could be applied to generate fairly realistic images of flooded houses. This will work best with single-family, suburban-type houses which are surrounded by an expanse of grass. From the 80 images in the test set, it was found that about 70% were successfully mapped to realistically flooded houses.
This initial version of the CycleGAN model will illustrate the feasibility of applying generative model to create personalized images of an extreme climate event i.e., flooding, that is expected to increase in frequency based on climate change projections. Subsequent versions of this model will integrate more varied types of houses and surroundings, as well as different types of climate-change related extreme event phenomena (i.e. droughts, hurricanes, wildfires, air pollution etc), depending on the expected impacts at a given location, as well as forecast time horizons. There’s still scope for improvement with regard to the color scheme of the generated images and the visual artifacts.
Furthermore to channel the emotional response of the public, into behavioural change or actions, the researchers are planning another improvement to the model called ‘choice knobs’. This will enable users to visually see the impact of their personal choices, such as deciding to use more public transportation, as well as the impact of broader policy decisions, such as carbon tax and increasing renewable portfolio standards.
The projects greater aim is to help the general population progress towards more visible public support for climate change mitigation steps on a national level, facilitating governmental interventions and helping make the required rapid changes to a global sustainable economy.
The researchers have stated that they need to explore more physical constraints to GAN training in order to incorporate more physical knowledge into these projections. This will enable a GAN model to transform a house to its projected flooded state and also take into account the forecast simulations of the flooding event represented by the physical variable outputs and probabilistic scenarios by a climate model for a given location.
Few developers have liked the idea of using technology, to produce realistic images depicting the effect of climate change in your own hometown which may make people understand the adverse effects of it.
https://twitter.com/jameskobielus/status/1129392932988096513
While some developers are not sure if showing people a picture of their house submerged in water is going to create any difference. A user on Hacker news comments, “The threshold for believing the effects of climate change has to change from reading/seeing to actually being there and touching it. Or some far more reliable system of remote verification has to be established”
Another user adds, “Is this a real paper? It's got to be a joke, right? a parody? It's literally a request to develop images to be used for propaganda purposes. And for those who will say that climate change is going to end the world, yeah, but that doesn't mean we should develop propaganda technology that could be used for some other political purpose.”
There are already many studies/evidences to make people aware of the effects of climate change, depicting a picture of their house submerged in water is not going to move them anymore. Climate change is already happening and effecting our day to day lives. What we need now are stronger approaches towards analysing, mitigating, and adapting to these changes and inspiring more government policies to fight against these climate changes.
To know more details about the project, head over to the research paper.
Amazon employees get support from Glass Lewis and ISS on its resolution for Amazon to manage climate change risks
ICLR 2019 Highlights: Algorithmic fairness, AI for social good, climate change, protein structures, GAN magic, adversarial ML and much more
Responsible tech leadership or climate washing? Microsoft hikes its carbon tax and announces new initiatives to tackle climate change