Introducing the modern data stack
The modern data stack is a combination of tools, technologies, and platforms that are designed to simplify the process of extracting, converting, and loading data from several sources into a centralized storage system. The stack components are generally chosen to fit the company’s needs exactly, hence promoting simplicity in addition to being cost effective. This stack enables businesses to manage, analyze, and gain insights from their data to make educated decisions. The current data stack’s components can be broadly classified in the following figure.
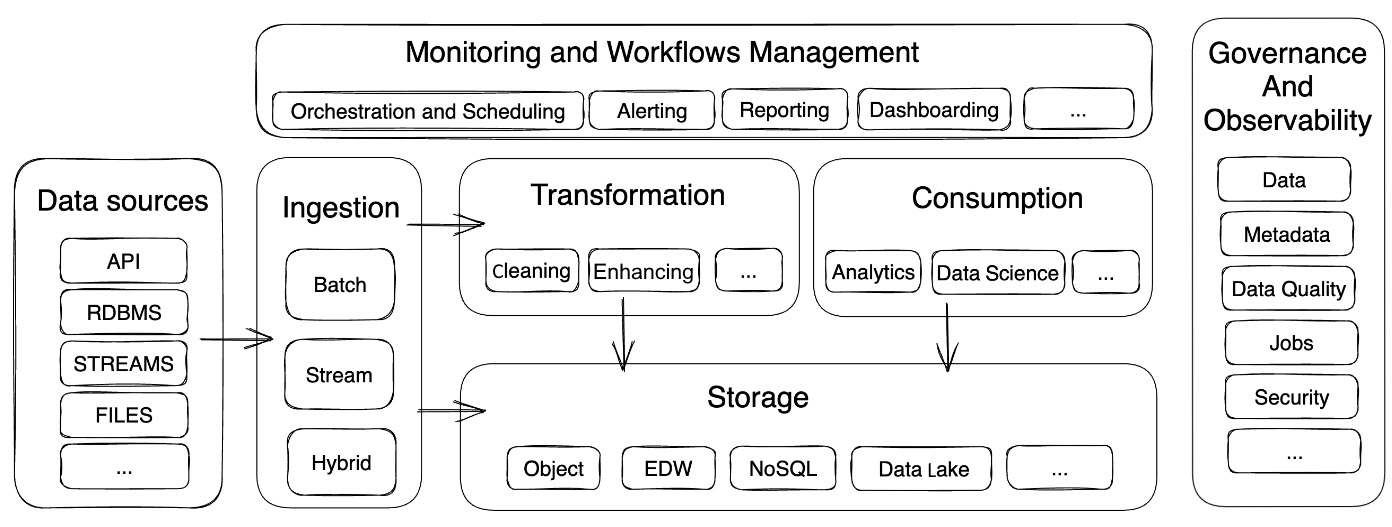
Figure 2.3 – Overview of the modern data stack
Initially, it is essential to identify the components encompassing the recognition, capturing, and measurement of data integrity for the information being integrated into the data platform. The modern data stack, with its multitude of components, provides organizations with a flexible and scalable framework for managing and deriving value from their data. By adopting the right tools, technologies, and platforms, organizations can create a powerful data ecosystem that supports their data-driven decision-making and business objectives.
Data sources
The data stack starts with the data sources, which can include relational databases, NoSQL databases, flat files, APIs, or data streams generated by sensors or devices. These sources are responsible for producing the raw data that will be ingested, processed, and stored within the modern data stack.
Tip
Data sources are the starting point of the modern data stack, providing the raw data that will be ingested, processed, and stored within the stack. Organizations should identify and evaluate their existing and potential data sources to determine their relevance, quality, and availability for their business objectives.
Data ingestion
Data ingestion refers to the process of moving and replicating data from various sources and loading it into the first step of the data layer with minimal transformation. Data ingestion can be used with real-time streaming, change data capture, APIs, or batching. Ingestion is the first step to ensure a smooth and efficient data transfer process. Tools such as Airbyte or Fivetran can help build this layer.
Storage
The modern data stack includes various storage technologies for managing and storing data. Various storage options exist, ranging from solutions that primarily provide efficient storage in terms of performance and non-specialized redundancy in the analytical aspect but are capable of adapting to different situations, to more specialized solutions offering high performance during data intersections required for various layers such as a data warehouse. The choice of data storage depends on the organization’s specific requirements and the type of data being managed. Technologies such as MinIO, Ceph, or Scality, which are distributed object storage systems compliant with S3 API, can be a good foundation for the storage layer.
Transformation
Data transformation is the process of combining data from different sources and creating a unified view. This process involves data cleansing, validation, enrichment, and transformation (filter, mapping, lookup, aggregate, and so on) to ensure data consistency and quality. At this stage, data transformation plays a crucial role. It facilitates the transfer and synchronization of various data types and formats between systems and applications. This step is commonly called data integration. Compute engines such as dbt or Spark can help process your data.
Note
Transformation is a key component of the modern data stack, as it ensures that the ingested data is consistent and standardized for analysis and consumption. Organizations should define their transformation logic and rules based on their business requirements and target system specifications.
Consumption
Data consumption can take various forms, with different methods employed to analyze and visualize information for distinct purposes. Three common approaches to data consumption include reporting/dashboarding, data science, and enterprise performance management (EPM).
Reporting and dashboarding are essential tools for organizations to effectively monitor their performance and make data-driven decisions. Reports provide structured and detailed information on various aspects of a business, while dashboards offer a visual representation of key performance indicators (KPIs) and metrics, allowing stakeholders to quickly grasp the overall health of the organization. The usage of technologies such as Tableau software combined with Presto-based solutions can help achieve that.
EPM is a comprehensive approach to company planning, consolidation, and reporting. EPM entails combining several management procedures, such as budgeting, forecasting, and financial analysis, to improve an organization’s overall performance. EPM assists businesses in achieving their goals and maintaining a competitive edge in the market by connecting business strategies with operational procedures.
Data science is an interdisciplinary field that combines cutting-edge tools and algorithms to extract insights from huge and complicated databases. Data scientists use techniques such as machine learning, statistical modeling, and artificial intelligence to forecast future trends, uncover patterns, and optimize business processes, allowing firms to make more informed strategic decisions.
Tip
Consumption is the ultimate goal of the modern data stack, as it enables organizations to analyze and visualize their integrated data for various purposes. Organizations should choose the appropriate tools and methods for data consumption based on their analytical needs and capabilities.
Management and monitoring
Workflow management and monitoring ensure a seamless execution of processes and timely delivery of accurate information. Workflow management focuses on designing, automating, and coordinating the various tasks, streamlining the process, and minimizing the risk of errors. On the other hand, monitoring upholds the effectiveness and dependability of data integration workflows. By continuously tracking the progress of data integration tasks, monitoring helps identify potential bottlenecks, performance issues, and data discrepancies. This real-time oversight allows organizations to proactively address problems and ensure data quality.
Data governance and observability
The set of policies, methods, and practices that regulate data collection, storage, and use is known as data governance. It tackles issues such as data quality, security, privacy, and compliance in order to ensure that data is accurate, consistent, and accessible to authorized users. A well-executed data governance structure can assist firms in maintaining data trust, reducing risks, and improving decision-making capabilities.
Observability, on the other hand, refers to the ability to monitor and comprehend the many components of a data ecosystem. It is necessary to monitor and visualize metrics, logs, and traces in order to get insight into the performance, dependability, and functionality of data pipelines, systems, and applications. Effective observability enables organizations to proactively identify and fix issues, maximize resource utilization, and ensure continuous data flow across their infrastructure. Observability, as opposed to monitoring, is concerned with the quality and consumption of data within the organization rather than technological factors. In many cases, tools such as DataHub can be very helpful in implementing observability.
The role of cloud-based technologies in the modern data stack
Cloud-based technologies have played a significant role in shaping the modern data stack, providing organizations with greater flexibility, scalability, and cost effectiveness compared to traditional on-premises solutions. Nonetheless, the cloud strategy is not limited to the public cloud but can also be implemented through various solutions within the private cloud. The following points highlight the importance of cloud-based technologies in the modern data stack:
- Scalability: Cloud-based services provide nearly limitless scalability, allowing businesses to quickly and easily modify their computing, storage, and processing capabilities to meet their needs. This adaptability assists businesses in avoiding overprovisioning and ensuring that they only pay for the resources they use.
- Cost effectiveness: Organizations can decrease capital costs on hardware, software, and maintenance by embracing cloud-based infrastructure and services. Cloud providers’ pay-as-you-go pricing model helps enterprises to better manage their operational costs while benefiting from cutting-edge technologies and functionalities.
- Speed and agility: Cloud-based solutions enable enterprises to swiftly provision and deploy new data stack components, allowing them to respond to changing business requirements more quickly. Businesses can experiment with new tools and technologies using cloud-based services without making large upfront infrastructure costs.
- Global availability: Cloud companies have data centers in multiple regions throughout the world, guaranteeing users have minimal latency and high availability. With a worldwide presence, businesses can store and process data closer to their customers, boosting performance and user experience.
- Integration and interoperability: Cloud-based data stack components are designed to interact smoothly with other cloud services, making it easier to connect and coordinate data activities across many platforms. This compatibility makes data handling more streamlined and efficient.
- Managed services: Cloud service providers provide managed services for various data stack components such as data integration, transformation, storage, and analytics. These managed services handle the underlying infrastructure, maintenance, and updates, allowing businesses to focus on essential business processes and gain value from their data.
- Security and compliance: Cloud companies invest heavily in security and compliance to ensure that their services fulfill industry standards and regulations. Organizations can benefit from advanced security features such as encryption, identity and access control, and network security by employing cloud-based services to protect their data and maintain compliance with data protection requirements.
- Tools and services ecosystem: The cloud ecosystem is home to a wide range of tools and services designed to meet the needs of the modern data stack. This diverse ecosystem enables enterprises to choose the finest tools and solutions for their individual use cases and objectives, fostering innovation and driving growth.
The paradigm has clearly shifted, as cloud-based technologies have transformed the modern data stack, offering businesses the flexibility, scalability, and cost effectiveness required to manage their data assets effectively. Organizations may build a robust, agile, and secure data stack that supports data-driven decision-making and business goals by implementing cloud-based solutions.
The evaluation of the data stack from traditional to cloud-based solutions
Over the years, the data stack has evolved significantly, shifting from traditional on-premises solutions to cloud-based technology. The necessity to manage rapidly growing volumes of data, as well as the growing need for real-time data processing and analytics, has fueled this change.
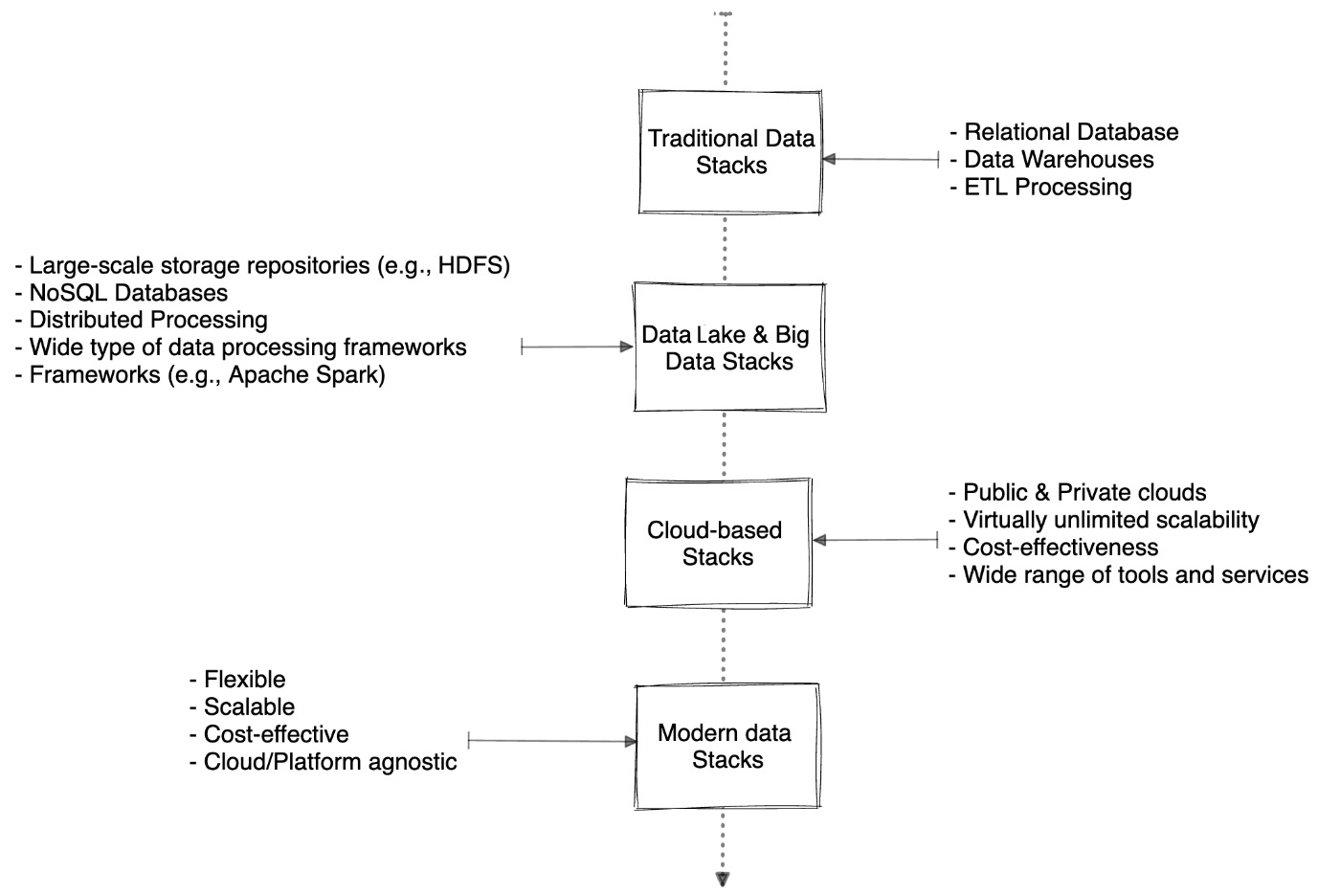
Figure 2.4 – Evolution of data stack
Traditional data stack
In the early days of data management, organizations primarily relied on monolithic, on-premises solutions such as relational databases and data warehouses. These systems were designed to handle structured data and were often limited in terms of scalability, flexibility, and integration capabilities. Data integration and processing tasks were typically performed using ETL processes, which were often time consuming and resource intensive.
The emergence of big data technologies and data lake architecture
The advent of big data technologies, such as Hadoop and NoSQL databases, marked a significant shift in the data stack landscape. These technologies were designed to handle large volumes of unstructured and semi-structured data, providing organizations with the ability to process and analyze diverse data sources. The implementation of distributed processing systems has significantly enhanced the handling and examination of large-scale data collections.
With the growing need to store and process various types of data, data lakes emerged as a popular alternative to traditional data warehouses. Data lakes are large-scale storage repositories that can store raw, unprocessed data in its native format, offering greater flexibility and scalability. Organizations began adopting data lake architectures to accommodate the diverse data types and sources they were working with, enabling them to perform more advanced analytics and derive deeper insights.
Cloud-based solutions
As cloud computing gained popularity, businesses began to use cloud-based services to construct and manage their data stacks. The cloud had various advantages over traditional options, including nearly limitless scalability, cost effectiveness, and access to a diverse set of tools and services. Cloud-based data storage solutions grew in popularity as a means of storing data on the cloud, while managed services offered scalable data warehousing and analytics capabilities.
Modern data stack
The modern data stack draws upon the cumulative advancements of previous iterations, harnessing the best aspects of each stack to deliver an optimized solution. This modern approach to data management is highly versatile, assuring its relevance and adaptability in today’s fast-changing technological scene. The introduction of IoT is a crucial development that has altered the modern data stack. With billions of connected devices across the world continuously producing large volumes of data, IoT has spurred the demand for efficient and scalable streaming solutions. These systems are specifically intended to handle real-time data processing, allowing enterprises to make more educated decisions based on current facts. The modern data stack also stresses data quality, governance, and security, ensuring that enterprises can trust and successfully manage their data.
The benefits of adopting a modern data stack approach
Adopting a modern data stack approach brings numerous benefits to organizations, allowing them to leverage the latest technologies and best practices in data management, integration, and analytics. Some of the key benefits of embracing a modern data stack include the following:
- Scalability: Modern data stacks are built on cloud-based technologies that offer virtually unlimited scalability, enabling organizations to handle growing volumes of data without worrying about infrastructure limitations. As data needs grow or fluctuate, the modern data stack can easily scale up or down to accommodate these changes, ensuring optimal performance and cost efficiency.
- Flexibility: The modern data stack is designed to accommodate diverse data sources and types, providing organizations with the ability to integrate and process data from various systems and formats. This flexibility allows organizations to derive insights from a wide range of data, supporting more comprehensive and informed decision-making.
- Agility: By leveraging modern data stack tools and services, organizations can accelerate their data integration, transformation, and analytics processes, enabling them to quickly respond to changing business requirements and market conditions. This agility helps organizations to stay competitive and adapt to the rapidly evolving business landscape.
- Cost efficiency: The adoption of a modern data stack built on cloud-based technologies enables organizations to take advantage of pay-as-you-go pricing models and eliminate the need for costly on-premises infrastructure investments. This cost efficiency allows organizations to optimize their data management expenses and allocate resources more effectively.
- Improved data quality and governance: A modern data stack emphasizes the importance of data quality, governance, and security. By adopting best practices and leveraging advanced data quality tools, organizations can ensure that their data is accurate, complete, and consistent, which in turn leads to more reliable insights and decision-making.
- Real-time data processing and analytics: The modern data stack enables organizations to process and analyze data in real time, allowing them to react to events and trends as they happen. This capability is particularly valuable for businesses that need to make timely decisions based on the latest data, such as those in finance, marketing, and operations.
- Ease of use and collaboration: Modern data stack tools and services are often designed with user friendliness and collaboration in mind, making it easier for teams to work together and access the data they need. This ease of use and collaboration helps organizations break down data silos and foster a more data-driven culture.
Adopting a modern data stack approach offers organizations numerous benefits, including scalability, flexibility, agility, cost efficiency, improved data quality, real-time analytics, and ease of use. By embracing the modern data stack, organizations can build a robust and agile data infrastructure that supports their data-driven decision making and business objectives.
Next, we’ll discuss culture and strategy.