If your client says they have an abundance of good quality data, be sure to take it with a pinch of salt. Data collection and processing are cost – and time-intensive tasks. There is always a chance that some data within the organization may not be of as high a quality as another data set. The problems in time series are often compounded by the time element. Due to challenges in data, organizations need to recalculate metrics and make changes to historical data, source additional data and build a mechanism to store it, as well as reconcile data when there are different definitions of data or a new data source doesn't reconcile with the previous sources. Data processing and collection may not be difficult for an organization if the requirement was for data going forward; historical data recalibration across a large time period, on the other hand, will present some challenges, as shown in the following diagram:
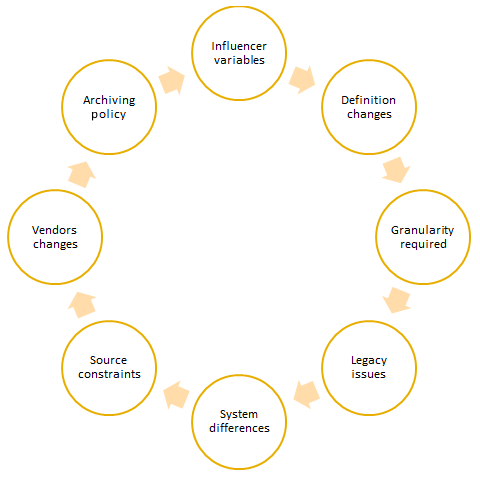