In this chapter, we approached two different ways in which to solve an RL problem. The first is through the estimation of state-action values that are used to choose the best next action, so-called Q-learning algorithms. The second involves the maximization of the expected reward policy through its gradient. In fact, these methods are called policy gradient methods. In this chapter, we showed the advantages and disadvantages of such approaches, and demonstrated that many of these are complementary. For example, Q-learning algorithms are sample efficient but cannot deal with continuous action. Instead, policy gradient algorithms require more data, but are able to control agents with continuous actions. We then introduced DPG methods that combine Q-learning and policy gradient techniques. In particular, these methods overcome the global maximization of the Q-learning algorithms...




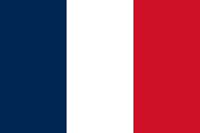


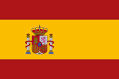



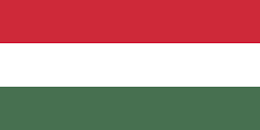

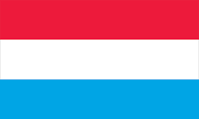





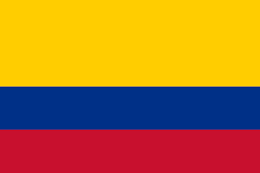
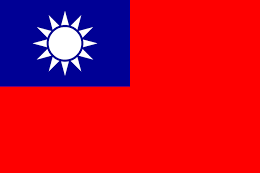
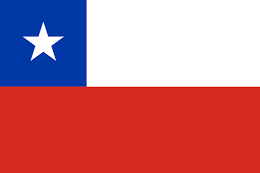



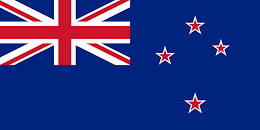
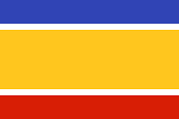
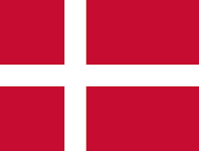
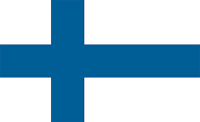







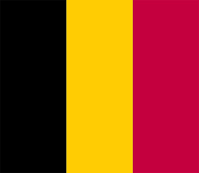

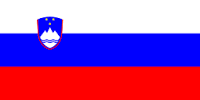





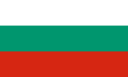


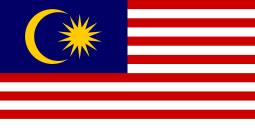



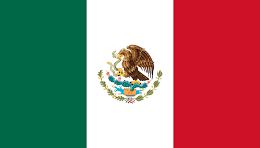
