Testing the baseline model
In this section, we will implement the code, which will give us an idea about how good or how bad our trained ML models perform in a validation set. We are using the mean accuracy score and the AUC-ROC score.
Here, we have generated five different classifiers and, after performing testing for each of them on the validation dataset, which is 25% of held-out dataset from the training dataset, we will find out which ML model works well and gives us a reasonable baseline score. So let's look at the code:.
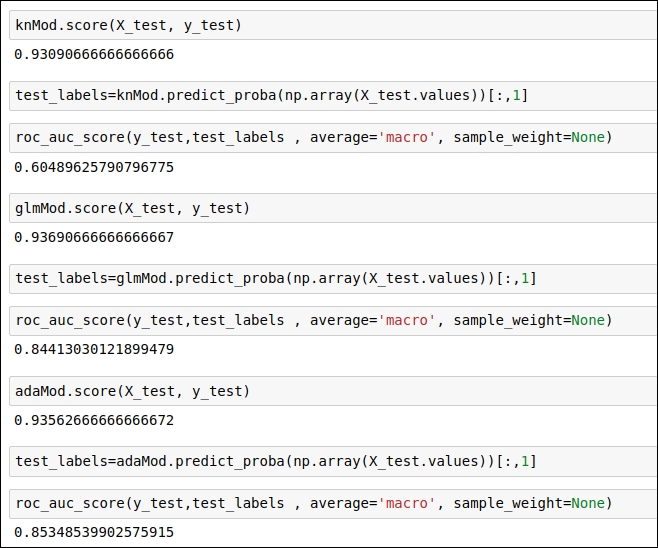
Figure 1.55: Code snippet to obtain a test score for the trained ML model
In the preceding code snippet, you can see the scores for three classifiers.
Refer to the code snippet in the following figure:
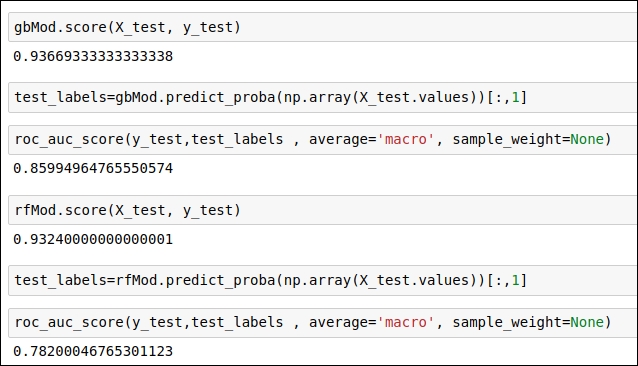
Figure 1.56: Code snippet to obtain the test score for the trained ML model
In the code snippet, you can see the score of the two classifiers.
Using the score()
function of scikit-learn, you will get the mean accuracy score, whereas, the roc_auc_score()
function will provide you with the ROC-AUC score, which is more significant for us because the mean accuracy score considers only one threshold value, whereas the ROC-AUC score takes into consideration all possible threshold values and gives us the score.
As you can see in the code snippets given above, the AdaBoost and GradientBoosting classifiers get a good ROC-AUC score on the validation dataset. Other classifiers, such as logistic regression, KNN, and RandomForest do not perform well on the validation set. From this stage onward, we will work with AdaBoost and GradientBoosting classifiers in order to improve their accuracy score.
In the next section, we will see what we need to do in order to increase classification accuracy. We need to list what can be done to get good accuracy and what are the current problems with the classifiers. So let's analyze the problem with the existing classifiers and look at their solutions.