In the MNIST example, we used the Softmax activation function as our last layer. You may recall that the layer generated an array of 10 probability scores, adding up to 1 for a given input. Each of those 10 scores referred to the likelihood of the image being presented to our network corresponding to one of the output classes (that is, it is 90% sure it sees a 1, and 10% sure it sees a 7, for example). This approach made sense for a classification task with 10 categories. In our sentiment analysis problem, we chose a sigmoid activation function, because we are dealing with binary categories. Using the sigmoid here simply forces our network to output a prediction between 0 and 1 for any given instance of data. Hence, a value closer to 1 means that our network believes that the given piece of information is more likely to be a positive review, whereas...




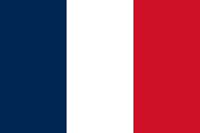


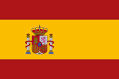



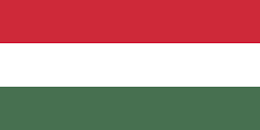

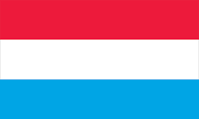





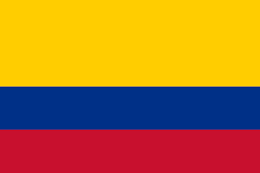
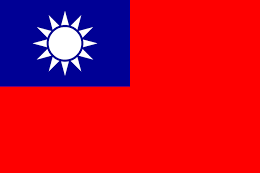
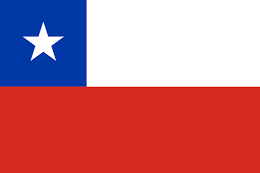



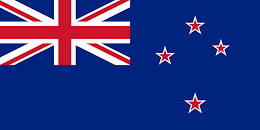
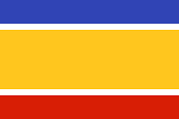
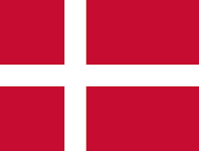
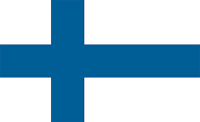







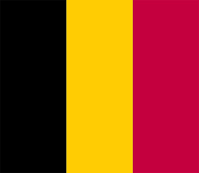

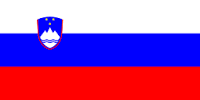





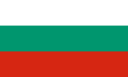


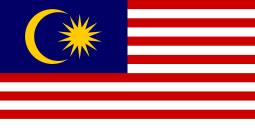



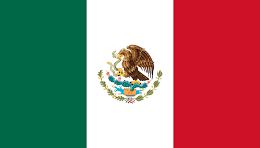
