The GRU can be considered the younger sibling of the LSTM, which we will look at Chapter 6, Long-Short Term Memory Networks. In essence, both leverage similar concepts to modeling long-term dependencies, such as remembering whether the subject of the sentence is plural, when generating following sequences. Soon, we will see how memory cells and flow gates can be used to address the vanishing gradient problem, while better modeling long term dependencies in sequence data. The underlying difference between GRUs and LSTMs is in the computational complexity they represent. Simply put, LSTMs are more complex architectures that, while computationally expensive and time-consuming to train, perform very well at breaking down the training data into meaningful and generalizable representations. GRUs, on the other hand, while computationally less intensive, are limited in their representational...




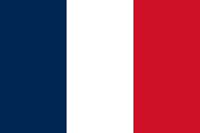


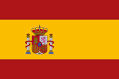



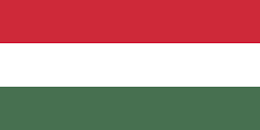

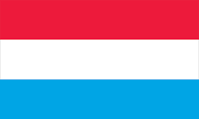





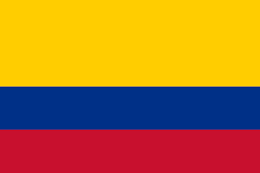
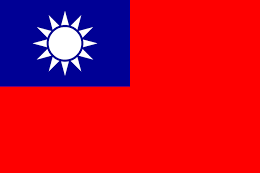
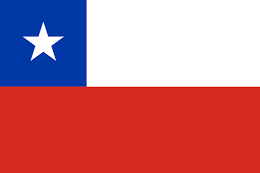



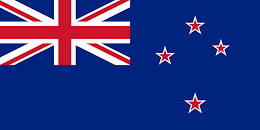
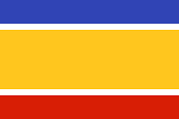
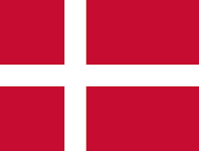
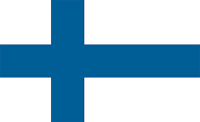







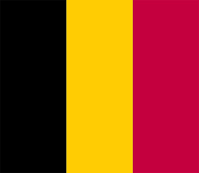

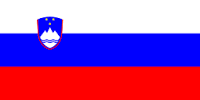





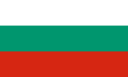


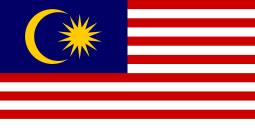



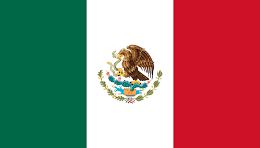
