All that we are doing here is applying a set of weights (that is, a filter) to local input spaces for feature extraction. We do this iteratively, moving our filter across the input space in fixed steps, known as a stride. Moreover, the use of different filters allows us to capture different patterns from a given input. Finally, since the filters convolve over the entire image, we are able to spatially share parameters for a given filter. This allows us to use the same filter to detect similar patterns in different locations of the image, relating to the concept of spatial invariance discussed earlier. However, these activation maps that a convolutional layer outputs are essentially abstract high-dimensional representations. We need to implement a mechanism to reduce these representations into more manageable dimensions, before we go ahead...




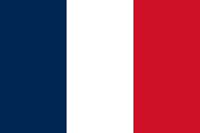


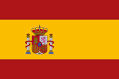



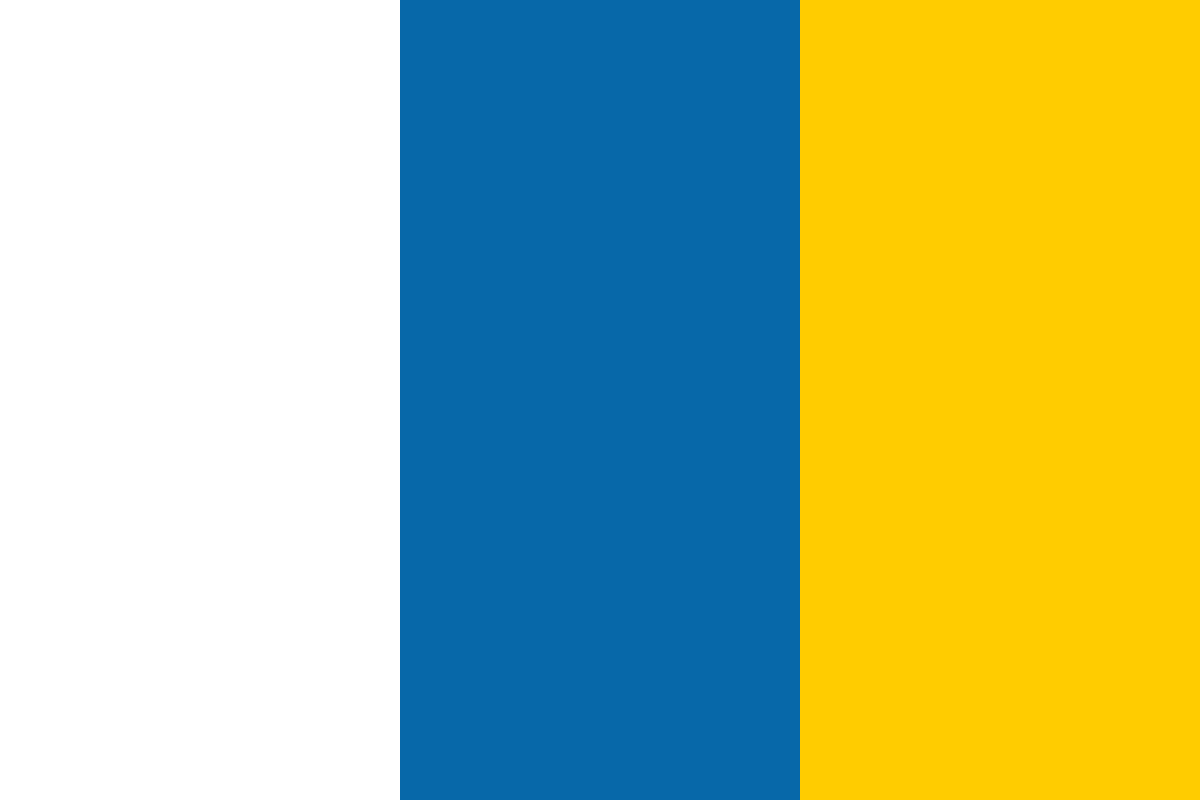
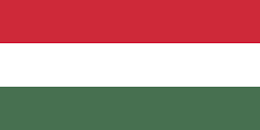

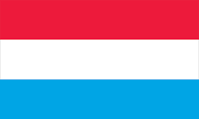





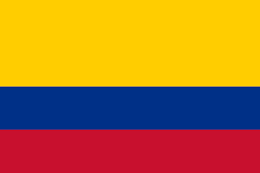
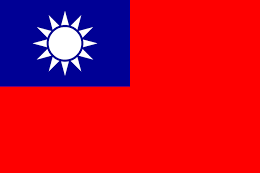
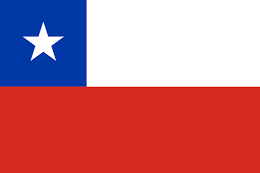



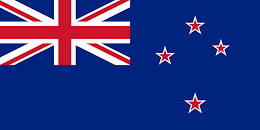
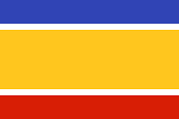
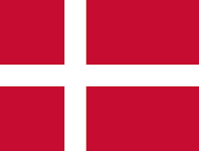
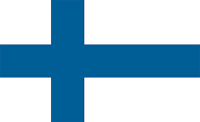







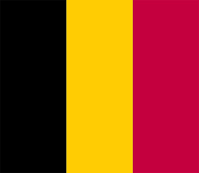

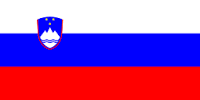





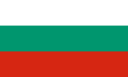


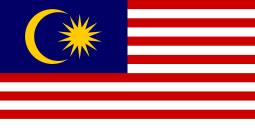



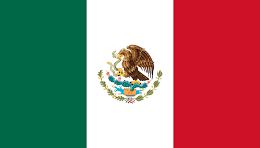
